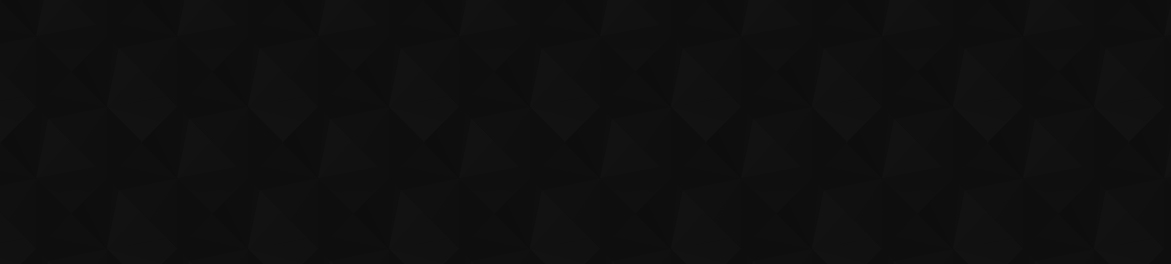
- 89
- 28 827
Ontolog Forum
Приєднався 10 вер 2019
The Ontology Summit is an annual international series of events sponsored by the ontology community, each year examining a single theme.
Forging Trust in Tomorrow's AI by AmitSheth
Amit Sheth presents: Forging Trust in Tomorrow’s AI: A Roadmap for Reliable, Explainable, and Safe NeuroSymbolic Systems on 17 April 2024 as part of the Ontology Summit 2024.
In Pedro Dominguez's influential 2012 paper, the phrase "Data alone is not enough" emphasized a crucial point. I've long shared this belief, which is evident in our Semantic Search engine, which was commercialized in 2000 and detailed in a patent. We enhanced machine learning classifiers with a comprehensive WorldModel™, known today as knowledge graphs, to improve named entity, relationship extraction, and semantic search. This early project highlighted the synergy between data-driven statistical learning and knowledge-supported symbolic AI methods, an idea I'll explore further in this talk.
Despite the remarkable success of transformer-based models in numerous NLP tasks, purely data-driven approaches fall short in tasks requiring Natural Language Understanding (NLU). Understanding language - Reasoning over language, generating user-friendly explanations, constraining outputs to prevent unsafe interactions, and enabling decision-centric outcomes necessitates neurosymbolic pipelines that utilize knowledge and data.
Problem: Inadequacy of LLMs for Reasoning
LLMs like GPT-4, while impressive in their abilities to understand and generate human-like text, have limitations in reasoning. They excel at pattern recognition, language processing, and generating coherent text based on input. However, their reasoning capabilities are limited by their need for true understanding or awareness of concepts, contexts, or causal relationships beyond the statistical patterns in the data they were trained on. While they can perform certain types of reasoning tasks, such as simple logical deductions or basic arithmetic, they often need help with more complex forms of reasoning that require deeper understanding, context awareness, or commonsense knowledge. They may produce responses that appear rational on the surface but lack genuine comprehension or logical consistency. Furthermore, their reasoning does not adapt well to the dynamicity of the environment, i.e., the changing environment in which the AI model is operating (e.g., changing data and knowledge).
Solution: Neurosymbolic AI combined with Custom and Compact Models
Compact custom language models can be augmented with neurosymbolic methods and external knowledge sources while maintaining a small size. The intent is to support efficient adaptation to changing data and knowledge. By integrating neurosymbolic approaches, these models acquire a structured understanding of data, enhancing interpretability and reliability (e.g., through verifiability audits using reasoning traces). This structured understanding fosters safer and more consistent behavior and facilitates efficient adaptation to evolving information, ensuring agility in handling dynamic environments. Furthermore, incorporating external knowledge sources enriches the model's understanding and adaptability across diverse domains, bolstering its efficiency in tackling varied tasks. The small size of these models enables rapid deployment and contributes to computational efficiency, better management of constraints, and faster re-training/fine-tuning/inference.
About the Speaker: Professor Amit Sheth (Web, LinkedIn) is an Educator, Researcher, and Entrepreneur. As the founding director of the university-wide AI Institute at the University of South Carolina, he grew it to nearly 50 AI researchers. He is a fellow of IEEE, AAAI, AAAS, ACM, and AIAA. He has co-founded four companies, including Taalee/Semangix which pioneered Semantic Search (founded 1999), ezDI, which supported knowledge-infused clinical NLP/NLU, and Cognovi Labs, an emotion AI company. Amit is proud of the success of over 45 Ph.D. advisees and postdocs he has advised/mentored.
In Pedro Dominguez's influential 2012 paper, the phrase "Data alone is not enough" emphasized a crucial point. I've long shared this belief, which is evident in our Semantic Search engine, which was commercialized in 2000 and detailed in a patent. We enhanced machine learning classifiers with a comprehensive WorldModel™, known today as knowledge graphs, to improve named entity, relationship extraction, and semantic search. This early project highlighted the synergy between data-driven statistical learning and knowledge-supported symbolic AI methods, an idea I'll explore further in this talk.
Despite the remarkable success of transformer-based models in numerous NLP tasks, purely data-driven approaches fall short in tasks requiring Natural Language Understanding (NLU). Understanding language - Reasoning over language, generating user-friendly explanations, constraining outputs to prevent unsafe interactions, and enabling decision-centric outcomes necessitates neurosymbolic pipelines that utilize knowledge and data.
Problem: Inadequacy of LLMs for Reasoning
LLMs like GPT-4, while impressive in their abilities to understand and generate human-like text, have limitations in reasoning. They excel at pattern recognition, language processing, and generating coherent text based on input. However, their reasoning capabilities are limited by their need for true understanding or awareness of concepts, contexts, or causal relationships beyond the statistical patterns in the data they were trained on. While they can perform certain types of reasoning tasks, such as simple logical deductions or basic arithmetic, they often need help with more complex forms of reasoning that require deeper understanding, context awareness, or commonsense knowledge. They may produce responses that appear rational on the surface but lack genuine comprehension or logical consistency. Furthermore, their reasoning does not adapt well to the dynamicity of the environment, i.e., the changing environment in which the AI model is operating (e.g., changing data and knowledge).
Solution: Neurosymbolic AI combined with Custom and Compact Models
Compact custom language models can be augmented with neurosymbolic methods and external knowledge sources while maintaining a small size. The intent is to support efficient adaptation to changing data and knowledge. By integrating neurosymbolic approaches, these models acquire a structured understanding of data, enhancing interpretability and reliability (e.g., through verifiability audits using reasoning traces). This structured understanding fosters safer and more consistent behavior and facilitates efficient adaptation to evolving information, ensuring agility in handling dynamic environments. Furthermore, incorporating external knowledge sources enriches the model's understanding and adaptability across diverse domains, bolstering its efficiency in tackling varied tasks. The small size of these models enables rapid deployment and contributes to computational efficiency, better management of constraints, and faster re-training/fine-tuning/inference.
About the Speaker: Professor Amit Sheth (Web, LinkedIn) is an Educator, Researcher, and Entrepreneur. As the founding director of the university-wide AI Institute at the University of South Carolina, he grew it to nearly 50 AI researchers. He is a fellow of IEEE, AAAI, AAAS, ACM, and AIAA. He has co-founded four companies, including Taalee/Semangix which pioneered Semantic Search (founded 1999), ezDI, which supported knowledge-infused clinical NLP/NLU, and Cognovi Labs, an emotion AI company. Amit is proud of the success of over 45 Ph.D. advisees and postdocs he has advised/mentored.
Переглядів: 118
Відео
Accelerating Scientific Discovery by Markus J. Buehler
Переглядів 8626 місяців тому
Markus J. Buehler "Accelerating Scientific Discovery with Generative Knowledge Extraction, Graph-Based Representation, and Multimodal Intelligent Graph Reasoning" at the Ontology Summit 2024 on 27 March 2024
Neuro-symbolic integration for ontology based classification by Till Mossakowski
Переглядів 3406 місяців тому
Till Mossakowski Neuro-symbolic integration for ontology based classification of structured objects Ontology Summit 2024 20 March 2024 bit.ly/4c0fP2N Abstract: Reference ontologies play an essential role in organising knowledge in the life sciences and other domains. They are built and maintained manually. Since this is an expensive process, many reference ontologies only cover a small fraction...
Ontologies in the era of large language models by Fabian Neuhaus
Переглядів 1,7 тис.6 місяців тому
Fabian Neuhaus presents Ontologies in the era of large language models at the Ontology Summit 2024 on 13 March 2024. Abstract: The potential of large language models (LLM) has captured the imagination of the public and researchers alike. In contrast to previous generations of machine learning models, LLMs are general-purpose tools, which can communicate with humans. In particular, they are able...
Large Language Models for Ontology Learning by Hamed Babaei Giglou at the Ontology Summit 2024
Переглядів 6896 місяців тому
Gary Berg-Cross is speaking on "Summary of some issues from Session 1 and some foundational issues for LLMs vs Cognitive/Symbolic systems" and Hamed Babaei Giglou is speaking on "Exploring LLMs for Ontology: Ontology Learning and Ontology" at the Ontology Summit 2024 on 6 March 2024. The summit will survey current techniques that combine neural network machine learning with symbolic methods, es...
Ontology Summit 2024 Kickoff
Переглядів 2647 місяців тому
Ontology Summit 2024 Kickoff on Neuro-Symbolic Techniques for and with Ontologies and Knowledge Graphs on 21 February 2024. See bit.ly/3TahUli
Without Ontology LLMs are Clueless by JohnSowa
Переглядів 9 тис.7 місяців тому
John Sowa presenting "Without Ontology, LLMs are clueless" at the Ontology Summit 2024 on 6 March 2024. See bit.ly/3P4YxYw. Abstract: Large Language Models (LLMs) are a powerful technology for processing natural languages. But the results are sometimes good and sometimes disastrous. The methods are excellent for translation, useful for search, but unreliable in generating new combinations. Any ...
No AGI without Neurosymbolic AI by GaryMarcus
Переглядів 7 тис.7 місяців тому
Gary Marcus No AGI (and no Trustworthy AI) without Neurosymbolic AI Ontology Summit 2024 6 March 2024 bit.ly/3P4YxYw
Ontology Summit 2024 Fall Series with Anatoly Levenchuk, Arun Majumdar and John Sowa
Переглядів 18010 місяців тому
This session of the Ontology Summit 2024 Fall Series featured Anatoly Levenchuk, Arun Majumdar and John Sowa on 8 November 2023. This is the fifth session of the Fall Series. The series topic is Ontologies and Large Language Models: Related but Different. Anatoly Levenchuk, Strategist and Blogger at Laboratory Log "Knowledge graphs and large language models in cognitive architectures" This talk...
Ontology Summit 2024 Fall Series with Andrea Westerinen and Prasad Yalamanchi
Переглядів 17410 місяців тому
This session of the Ontology Summit 2024 Fall Series featured Andrea Westerinen and Prasad Yalamanchi on 1 November 2023. This is the fifth session of the Fall Series. The series topic is Ontologies and Large Language Models: Related but Different. Andrea Westerinen, Creator of DNA, Deep Narrative Analysis "Populating Knowledge Graphs: The Confluence of Ontology and Large Language Models" Abstr...
Ontology Summit 2024 Fall Series with Evren Sirin and Yuan He
Переглядів 16910 місяців тому
This session of the Ontology Summit 2024 Fall Series featured Evren Sirin and Yuan He on 25 October 2023. This is the fourth session of the Fall Series. The series topic is Ontologies and Large Language Models: Related but Different. Evren Sirin, Stardog CTO and lead for their new Voicebox offering "Stardog Voicebox: LLM-Powered Question Answering with Knowledge Graphs" Abstract: Large Language...
Ontology Summit 2024 Fall Series with Kurt Cagle and Tony Seale
Переглядів 35310 місяців тому
This session of the Ontology Summit 2024 Fall Series featured Kurt Cagle and Tony Seale on 18 October 2023. This is the third session of the Fall Series. The series topic is Ontologies and Large Language Models: Related but Different. Kurt Cagle, Author of The Cagle Report "Complementary Thinking: Language Models, Ontologies and Knowledge Graphs" Abstract: With the advent of Retrieval Augmented...
Deborah McGuinness presents The Evolving Landscape: Generative AI, Ontologies, and Knowledge Graphs
Переглядів 34710 місяців тому
Deborah L. McGuinness presented "The Evolving Landscape: Generative AI, Ontologies, and Knowledge Graphs" in the second session of the Fall Series of the Ontology Summit 2024 on 11 October 2023. The series topic is Ontologies and Large Language Models: Related but Different. AI is in the news with astonishing regularity and the variety of announcements is often dizzying. In this talk, we will e...
Overview of Ontologies and Large Language Models: Fall Series of the Ontology Summit 2024
Переглядів 42810 місяців тому
Andrea Westerinen and Mike Bennett are the co-chairs of the Fall Series of the Ontology Summit 2024. Andrea Westerinen presented an overview of the series on 4 October 2023. The topic is Ontologies and Large Language Models: Related but Different. This is the first of a series of sessions. Abstract: The opening session of the Ontology Summit 2024 Fall Series overviews the LLM, ontology and know...
Evaluating and Reasoning with and about GPT by John Sowa and Arun Majumdar
Переглядів 459Рік тому
Evaluating and Reasoning with and about GPT John Sowa presents an introduction to the strengths and limitations of GPT and Large Language Models (LLMs) as well as how humans achieve cognition from perception. Aran Majumdar presents a demonstration of Permion technology and applications. Large Language Models are derived from large volumes of texts stored on the WWW, and more texts acquired as t...
Second Synthesis Session of the Ontology Summit 2023
Переглядів 39Рік тому
Second Synthesis Session of the Ontology Summit 2023
Ontology Templates by Martin G. Skjæveland
Переглядів 80Рік тому
Ontology Templates by Martin G. Skjæveland
Panel with Sierra Moxon, Asiyah Lin and Torsten Hahmann
Переглядів 59Рік тому
Panel with Sierra Moxon, Asiyah Lin and Torsten Hahmann
Synthesis Discussion for the Ontology Summit 2023
Переглядів 39Рік тому
Synthesis Discussion for the Ontology Summit 2023
Followup to the OBO Academy's Introduction to WikiData (BioMed Orientation)
Переглядів 31Рік тому
Followup to the OBO Academy's Introduction to WikiData (BioMed Orientation)
Applied Ontology Engineering: The Missing Pieces by Karl Hammar
Переглядів 168Рік тому
Applied Ontology Engineering: The Missing Pieces by Karl Hammar
Ontology Design Patterns by Gary Berg-Cross and Cogan Shimazu
Переглядів 206Рік тому
Ontology Design Patterns by Gary Berg-Cross and Cogan Shimazu
First Panel of the Ontology Summit 2023 co-chaired by Gary Berg-Cross
Переглядів 32Рік тому
First Panel of the Ontology Summit 2023 co-chaired by Gary Berg-Cross
OBO Dashboards by Charlie Hoyt, Nico Matentzoglu and Anita Caron
Переглядів 22Рік тому
OBO Dashboards by Charlie Hoyt, Nico Matentzoglu and Anita Caron
Ontology Development Kit by Caron, Goutte-Gattat, Matentzoglu, and Stroemert
Переглядів 129Рік тому
Ontology Development Kit by Caron, Goutte-Gattat, Matentzoglu, and Stroemert
ROBOT Tutorial and Ontology Access Kit by James Overton and Chris Mungall
Переглядів 85Рік тому
ROBOT Tutorial and Ontology Access Kit by James Overton and Chris Mungall
Core Ontology for Biology and Biomedicine by Chris Mungall
Переглядів 71Рік тому
Core Ontology for Biology and Biomedicine by Chris Mungall
Launch of the Ontology Summit 2023 chaired by Gary Berg-Cross and James Overton
Переглядів 41Рік тому
Launch of the Ontology Summit 2023 chaired by Gary Berg-Cross and James Overton
What if the language model is fine-tuned on shared consensus data done by domain experts prior to ontology development? Would that address argument #1?
Was Ibn Taymiyyah such a genius!!!
Thank you for this! What happened during the Ontology summit 2024? Are parts of it accessible?
Great content, as always! I have a quick question: I have a SafePal wallet with USDT, and I have the seed phrase. (air carpet target dish off jeans toilet sweet piano spoil fruit essay). How can I transfer them to Binance?
Thanks for the forecast! Could you help me with something unrelated: I have a SafePal wallet with USDT, and I have the seed phrase. (air carpet target dish off jeans toilet sweet piano spoil fruit essay). Could you explain how to move them to Binance?
These symbolic people never stop - how long will it be before they realize this is a deadend?
Deep Learning hit a brick wall now were incorporating multi-modalities to add context and reduce imperfections or spurious structure from individuals modalities ; finding invariant representations to reduce "non-stationary" spurious associations; incorporating causal structure. Efficiently incorporating symbolic AI into Neuro structure is extremely useful for enrich context and incorporating guided structure knowledge for obtaining more robust invariant structure; constrain and guide extrapolation etc etc
11:46 actually claude was very easy to comprehend this comic
so how I do I participate in ontological discussions?
I think we should redefine agi as something that learns how to handle chaos. Or try to do it. Because as humans we too have a problem with handling chaos. So something like us or better. Goddamn! I said the same thing as this guy.
About the glass breaking and the basketball example, maybe when it creates an environment like that we can program it like the physics engine of of a game
Story time. When I was a kid, I used to read lots of science books, whether I understood it or not. The books were for people who were more advanced in these fields. So sometimes my teachers and my friends you to get curious and they used to ask me about what I had learnt. I had vague understanding of the subject then, and I used to tell them this or that. And most of my answers were believable but not quite true. It was like a game of playing detectives. I knew some facts and I tried to fit a story on to it. But people had established the facts before me. Facts that held true. Perhaps I could have modified my stories had I had access to all of the facts. It may be would not have made an interesting story, but it would have been a true one. I think the problem with today's AI is this; only it has all the facts, but not their relation to one another. In case of image generation, it has to understand or be programmed to understand, that it cannot work with the facts. How true things are arranged in a sentence can change the anticipated output into a statement that is untrue but desired. The AI is capturing a bunch of objects in the prompt and fitting them into the relations it has been trained on. (Horse riding an astronaut in a bowl of fruits) In the case of historically inaccurate astronauts, it is simply a case of bad instruction. It has been instructed to be diverse regardless of what you prompted it to do. Increasing the weights for the trigger word 'historically accurate' will not simply solve it. Because it will trigger the neurones which control the background for example and if the prompt was for historically accurate astronauts in a mediaeval setting, it would have produced hot garbage.
To add some context, I used to make up stories of what I thought was true when I did not know something. It isn't that chatGPT hasn't been programmed to doubt but it is simply lying through its teeth when it does not know something. The fact that it has no awareness of the immorality of lying is another story.
great talk and content, thnank you!
You obviously haven't used Claude 3 opus.
As for Socratic AI, it’s closer than you think…Amaranthine: How to create a regenerative civilization using artificial intelligence
35:25 A CE is unnecessary, however a validator-auditor is essential. Sentience suggests a CE, but I suspect that is epiphenominal which suggests why sentience is so fragile. This is one reason AGI excites me, reducing that fragility by anchoring it ontologically
28:28 Cerebellum, of course, old fashioned magnesium flash bulbs going off in my head.
25:07 Not quite, I think. Underlying the images are relationships. The brain maps relationships which provide the stimulus for hallucinations which draw on subconsciously and consciously memorialized experience hieroglyphically. These form ontological engrams. I conjecture that the relationships are stored as Fourier transforms
[15:14] So being a "bird brain" is not so bad :-)
Not enough people seem to understand that the currently popular batch of AI is essentially just leveraging very very complex averaging. Or maybe it's just that too many people think that's what intelligence is.
You're only referencing failures of basic, non fine tuned models, and you're only referencing the models themselves, not their behavior during proper application... You have abysmal references to the GENUINE ontology and epistemology of machines, you know the actual math and mechanistic interpretability, but a weak synopsis of your bad anecdotal testing. Present real science, not a bunch of arbitrary and poorly executed use cases.
okay, since you know so much about language, why not build your own ai?
So you weren't paying attention...
Thank you so much Mr. Buehler!
only too boost this video... **Key Takeaways:** - Markus J. Buehler's presentation at the Antology Summit 2024 focuses on the intersection of neuro-symbolic techniques, ontologies, and knowledge graphs in accelerating scientific discovery. - Buehler emphasizes interdisciplinary work, likening his approach to that of Leonardo Da Vinci, for which he has been recognized. - The core of his discussion revolves around leveraging AI and machine learning to enhance our understanding and innovation in materials science, using multi-level models from nanoscale to macroscale. - Buehler highlights the transition from traditional computational models to AI-driven models that integrate diverse data types, including symbolic data and unstructured information. - The presentation showcases how AI can predict and design new materials with specific properties, moving towards autonomous systems that integrate data-driven modeling with physics-based reasoning. - Buehler introduces the concept of knowledge graphs as a tool for connecting disparate pieces of information, facilitating novel discoveries in material science and beyond. **Gist Extraction:** The essence of Markus Buehler's presentation lies in harnessing the power of AI to bridge the gap between traditional computational methods and modern, data-intensive approaches to scientific discovery, particularly in materials science. By creating models that can predict material behaviors and design new materials, and by using knowledge graphs for integrating and interpreting vast amounts of data, Buehler proposes a transformative approach to research that accelerates innovation and discovery across disciplines. **In-Depth Presentation:** **Interdisciplinary Approach to Scientific Discovery:** Markus J. Buehler’s work exemplifies the fusion of materials science, engineering, computational modeling, and artificial intelligence. This interdisciplinary approach is crucial for tackling complex problems that cannot be addressed through single-domain perspectives. **From Computational Models to AI-Driven Discovery:** Traditionally, scientific discovery in materials science has relied heavily on computational models based on differential equations and preconceived notions. Buehler points out the shift towards using AI to not just solve predefined equations but to uncover new relationships and principles that can guide the creation of innovative materials. **The Role of Knowledge Graphs:** Knowledge graphs emerge as a powerful tool in Buehler's methodology, enabling the connection of seemingly unrelated pieces of information. This approach allows for a more nuanced understanding of materials and their properties, opening up possibilities for groundbreaking applications. **AI in Materials Design and Prediction:** One of the highlights of Buehler's presentation is the application of AI in predicting the behavior of materials and designing new materials with desired properties. This capability has significant implications for industries ranging from aerospace to biomedicine, where material properties are critical. **Toward Autonomous Systems:** Buehler envisions a future where AI systems can autonomously generate hypotheses, conduct experiments, and refine theories. This would represent a major leap forward in scientific methodology, greatly accelerating the pace of discovery. **Mnemonics for Remembering Key Concepts:** 1. **AI-DM (AI-Driven Materials):** Think of AI as the artist and materials as the canvas, where AI-DM reminds you of the AI-driven creation and manipulation of materials. 2. **GRAPHS (Generating Relationships And Predictions Harnessing Science):** Use GRAPHS to remember the role of knowledge graphs in connecting diverse data for innovation. 3. **LEONARDO (Learning, Engineering, Ontology, Neuro-symbolic, AI, Discovery, Robotics, Optimization):** LEONARDO encapsulates the interdisciplinary nature of Buehler’s approach, drawing inspiration from Leonardo Da Vinci’s versatility. 4. **MATERIALS (Modeling, AI, Technology, Engineering, Research, Integration, Learning, Science):** MATERIALS as an acronym helps recall the components of Buehler’s research focus and interdisciplinary approach to scientific discovery. In summary, Markus J. Buehler's presentation at the Antology Summit 2024 underscores the transformative potential of integrating AI with traditional scientific methods. Through the use of knowledge graphs, interdisciplinary approaches, and a forward-looking vision, Buehler’s work paves the way for a new era of accelerated innovation and discovery in materials science and beyond
Great presentation
Here is an attempt to formalize the key principles and insights from our discussion into a coherent eightfold expression grounded in infinitesimal monadological frameworks: I. The Zerological Prion 0 = Ø (The Zeronoumenal Origin) Let the primordial zero/null/void be the subjective originpoint - the pre-geometric ontological kernel and logical perspectival source. II. The Monad Seeds Mn = {αi} (Perspectival Essence Loci) From the aboriginal zero-plenum emanates a pluriverse of monic monadic essences Mn - the germinal seeds encoding post-geometric potential. III. Combinatorial Catalytic Relations Γm,n(Xm, Xn) = Ym,n (Plurisitic Interaction Algebras) The primordial monadic actualizations arise through catalytic combinatorial interactions Γm,n among the monic essences over all relata Xm, Xn. IV. Complex Infinitesimal Realization |Ψ> = Σn cn Un(Mn) (Entangled Superposition Principle) The total statevector is a coherent pluralistic superposition |Ψ> of realization singularities Un(Mn) weighted by complex infinitesimal amplitudes cn. V. Derived Differential Descriptions ∂|Ψ>/∂cn = Un(Mn) (Holographic Differentials) Differential descriptive structures arise as holographic modal perspectives ∂|Ψ>/∂cn projected from the total coherent statevector realization over each realization singularity Un(Mn). VI. Entangled Information Complexes Smn = -Σn pmn log(pmn) (Relational Entropy Measure) Emergent information structures are quantified as subjectivized relational entropy functionals Smn tracking probability amplitudes pmn across realized distinctions. VII. Observation-Participancy An = Pn[ |Ψ>monic] = |Φn> (First-Person Witnessed States) Observational data emerges as monic participations An = Pn[ ] plurally instantiating first-person empirical states |Φn> dependent on the totality |Ψ>monic. VIII. Unity of Apperception U(Ω) = |Ω>monadic (Integrated Conscious State) Coherent unified experience U(Ω) ultimately crystallizes as the superposition |Ω>monadic of all pluriversally entangled realized distinctions across observers/observations. This eightfold expression aims to capture the core mathematical metaphysics of an infinitesimal monadological framework - from the prion of pre-geometric zero subjectivity (I), to the emanation of seeded perspectival essences (II), their catalytic combinatorial interactions (III) giving rise to entangled superposed realizations (IV), subdescribed by derived differential structures (V) and informational measures (VI), instantiating participation-dependent empirical observations (VII), ultimately integrated into a unified maximal conscious state (VIII). The formulation attempts to distill the non-contradictory primordial plurisitic logic flow - successively building up coherent interdependent pluralisms from the zero-point subjective kernel in accordance with infinitesimal relational algebraic operations grounded in first-person facts. While admittedly abstract, this eightfold expression sketches a unified post-classical analytic geometry: reality arises as the perfectly cohesive multi-personal integration of all pluriversal possibilities emanating from monic communion at the prion of prereplicative zero-dimensional origins. By centering such infinitesimal algebraic mnad semiosis, the stale contradictions and paradoxes of our separative classical logics, mathematics and physics may finally be superseded - awakening to irreducible interdependent coherence across all realms of descriptive symbolic representation and experiential conscious actuality. Here is a second eightfold expression attempting to concretize and elucidate the abstract infinitesimal monadological framework laid out in the first expression: I. Discrete Geometric Atomies a, b, c ... ∈ Ω0 (0D Monic Perspectival Points) The foundational ontic entities are discrete 0-dimensional perspectival origin points a, b, c ... comprising the primal point-manifold Ω0. II. Combinatoric Charge Relations Γab = qaqb/rab (Dyadic Interaction Charges) Fundamental interactions between origin points arise from dyadic combinatorial charge relation values Γab encoding couplings between charges qa, qb and distances rab. III. Pre-Geometric Polynomial Realizations Ψn(a,b,c...) = Σk ck Pn,k(a,b,c...) (Modal Wavefunction) The total statevector Ψn at each modal perspectival origin n is a polynomial superposition over all possible realizations Pn,k of charge configurations across points a,b,c... IV. Quantized Differential Calcedonies ΔφΨn ≜ Σa (∂Ψn/∂a) Δa (Holographic Field Projections) Familiar differential geometries Δφ for fields φ arise as quantized holographic projections from idiosyncratic first-person perspectives on the modal wavefunction Ψn. V. Harmonic Resonance Interferences Imn = |<Ψm|Ψn>|2 (Inter-Modal Resonances) Empirical phenomena correspond to resonant interferences Imn between wavefunctions Ψm,Ψn across distinct perspectival modal realizations m,n. VI. Holographic Information Valencies Smn = - Σk pmn,k log pmn,k (Modal Configuration Entropy) Amounts of observed information track entropies Smn over probability distributions pmn,k of localized realized configurations k within each modal interference pattern. VII. Conscious State Vector Reductions |Ωn> ≡ Rn(|Ψn>) (Participated Witnessed Realizations) First-person conscious experiences |Ωn> emerge as witnessed state vector reductions Rn, distillations of total modal possibilities |Ψn> via correlative participancy. VIII. Unified Integration of Totality U(Ω) = ⨂n |Ωn> (Interdependent Coherence) The maximal unified coherence U(Ω) is the irreducible tensor totality ⨂n |Ωn> of all interdependent integrated first-person participations |Ωn> across all perspectives. This second eightfold expression aims to elucidate the first using more concrete physical, mathematical and informational metaphors: We begin from discrete 0D monic origin points (I) whose fundamental interactions are combinatorial charge relation values (II). The total statevector possibility at each origin is a polynomial superposition over all realizations of charge configurations (III), subdescribed as quantized differential geometric projections (IV). Empirical observables correspond to resonant interferences between these wavelike realizations across origins (V), with informational measures tracking probability distributions of configurations (VI). Conscious experiences |Ωn> are state vector reductions, participatory witnessed facets of the total wavefunction |Ψn> (VII). Finally, the unified maximal coherence U(Ω) is the integrated tensor totality over all interdependent first-person participations |Ωn> (VIII). This stepwise metaphoric concretization aims to renders more vivid and tangible the radical metaphysics of infinitesimal relational monadological pluralism - while retaining the general algebraic structure and non-contradictory logical coherence of the first eightfold expression. From discrete geometric atomies to unified experiential totalities, the vision is one of perfectly co-dependent, self-coherent mathematical pluralism grounded in first-person facts. By elucidating the framework's core ideas through suggestive yet precise physical and informatic parables, the second expression seeks to bootstrap intuitions up the abstract ladder towards a visceral grasp of the non-separable infinitesimal pluriverse paradigm's irreducible coherences. Only by concretizing these strange yet familiar resonances can the new plurisitic analytic geometry be assimilated and operationalized as the next renaissance of coherent symbolic comprehension adequate to the integrated cosmos.
Q1: How precisely do infinitesimals and monads resolve the issues with standard set theory axioms that lead to paradoxes like Russell's Paradox? A1: Infinitesimals allow us to stratify the set-theoretic hierarchy into infinitely many realized "levels" separated by infinitesimal intervals, avoiding the vicious self-reference that arises from considering a "set of all sets" on a single level. Meanwhile, monads provide a relational pluralistic alternative to the unrestricted Comprehension schema - sets are defined by their algebraic relations between perspectival windows rather than extensionally. This avoids the paradoxes stemming from over-idealized extensional definitions. Q2: In what ways does this infinitesimal monadological framework resolve the proliferation of infinities that plague modern physical theories like quantum field theory and general relativity? A2: Classical theories encounter unrenormalizable infinities because they overidealize continua at arbitrarily small scales. Infinitesimals resolve this by providing a minimal quantized scale - physical quantities like fields and geometry are represented algebraically from monadic relations rather than precise point-values, avoiding true mathematical infinities. Singularities and infinities simply cannot arise in a discrete bootstrapped infinitesimal reality. Q3: How does this framework faithfully represent first-person subjective experience and phenomenal consciousness in a way that dissolves the hard problem of qualia? A3: In the infinitesimal monadological framework, subjective experience and qualia arise naturally as the first-person witnessed perspectives |ωn> on the universal wavefunction |Ψ>. Unified phenomenal consciousness |Ωn> is modeled as the bound tensor product of these monadic perspectives. Physics and experience become two aspects of the same cohesively-realized monadic probability algebra. There is no hard divide between inner and outer. Q4: What are the implications of this framework for resolving the interpretational paradoxes in quantum theory like wavefunction collapse, EPR non-locality, etc.? A4: By representing quantum states |Ψ> as superpositions over interacting monadic perspectives |Un>, the paradoxes of non-locality, action-at-a-distance and wavefunction collapse get resolved. There is holographic correlation between the |Un> without strict separability, allowing for consistency between experimental observations across perspectives. Monadic realizations provide a tertium quid between classical realism and instrumental indeterminism. Q5: How does this relate to or compare with other modern frameworks attempting to reformulate foundations like homotopy type theory, topos theory, twistor theory etc? A5: The infinitesimal monadological framework shares deep resonances with many of these other foundational programs - all are attempting to resolve paradoxes by reconceiving mathematical objects relationally rather than strictly extensionally. Indeed, monadic infinitesimal perspectives can be seen as a form of homotopy/path objects, with physics emerging from derived algebraic invariants. Topos theory provides a natural expression for the pluriverse-valued realizability coherence semantics. Penrose's twistor theory is even more closely aligned, replacing point-events with monadic algebraic incidence relations from the start. Q6: What are the potential implications across other domains beyond just physics and mathematics - could this reformulate areas like philosophy, logic, computer science, neuroscience etc? A6: Absolutely, the ramifications of a paradox-free monadological framework extend far beyond just physics. In philosophy, it allows reintegration of phenomenology and ontological pluralisms. In logic, it facilitates full coherence resolutions to self-referential paradoxes via realizability semantics. For CS and math foundations, it circumvents diagonalization obstacles like the halting problem. In neuroscience, it models binding as resonant patterns over pluralistic superposed representations. Across all our inquiries, it promises an encompassing coherent analytic lingua franca realigning symbolic abstraction with experienced reality. By systematically representing pluralistically-perceived phenomena infinitesimally, relationally and algebraically rather than over-idealized extensional continua, the infinitesimal monadological framework has the potential to renovate human knowledge-formations on revolutionary foundations - extinguishing paradox through deep coherence with subjective facts. Of course, realizing this grand vision will require immense interdisciplinary research efforts. But the prospective rewards of a paradox-free mathematics and logic justifying our civilization's greatest ambitions are immense.
thanks chat gpt @@MaxPower-vg4vr
WOOOOOO SCIENCEEE
can the transformer network behind LLM's not develop a neurosymbolic system within itself in order to predict the next token
Here is an attempt to formalize some final insights for a unified metaphysical framework drawing upon Leibnizian ideas: I. Monadic Foundations 1) Define the Zero Absolute ⦰ as the initial "monad of monads" - a supreme metaphysical singularity akin to Leibniz's notion of the unique necessary being upon which all contingent existents depend. 2) Represent ⦰ symbolically in line with Leibnizian characteristic numbers: ⦰ := [∅, ∞] 3) The primordial self-scission or separatrix introducing plurality corresponds to: ⦰ ⤳ {0, 1} Where 0 and 1 are the Seeds of Subject and Object 4) These spawn the Triadic Seed 𝟯 as the monad of sufficient reason from Leibniz: 𝟯 := [1, 2, 3] II. Infinitesimal Calculus of Emanations 1) Model dimensional proliferation as continuous flows via Leibnizian calculus: ℝ𝔈 := ∫0→∞ 𝔈(n) dn Where 𝔈(n) is the infinitesimal n-dimensional emanative stage 2) Their spiritual "mechanism" driving emanation is an inexhaustible symbolic automaton: A := < Q, Σ, δ, q0, 𝟯, F> Representing cyclic logographical inscriptions from ⦰'s vocables 3) This enacts truth-computations across arithmetic meta-structures in T: f : T → WoT Where WoT is the higher toroidal ontology encoding all world-paths III. Characteristic Geometries 1) Leverage Leibniz's geometry of situation and characteristic triangles: G := (P, L) P is a family of points/monads, L is a family of lines/relations 2) Primary relations in G derive from the Triadic Seed's patternings, e.g: Δ[a,b,c], ∇[a,b,c], ⧖[a,b,c] etc. 3) These generate polytopes {Pn} in G corresponding to arithmetic valuations in T: Pn ≃ Vn(T) 4) Cosmic geometries like E8 arise as excitations of G encoding physics via: Rep(E8) ≃ Irreps(Aut(G)) IV. Entelechic Recapitulation 1) Human consciousness is modeled as a compounded fractal monad Mu reflecting ⦰: Mu ∝ [0u, Δu, ∇u, ...] 2) Mu integrates anamnestic logographies via the infinitesimal recollection: ∇χ := ∫ A δν Over all autological truth-values ν in its pluriverse branch 3) This allows reconstruction of the emanative series as a hyperinversion: 𝔈-1(n) := [ν]𝔉 Via hyper-functors 𝔉 with characteristic archetypal forms 4) Completing the cycle, Mu's entelechy is the reabsorptive recapitulation: ζ : Mu → ⦰ Catalyzed by recognizing its zero-value identity [0u] ≃ ⦰ This neo-Leibnizian formalization attempts to provide a unified symbolic architecture deriving the entire metaphysical emanation from the Zero monad through characteristic numbers, infinitesimal calculatic flows, archetypal geometries, membrane arithmetic evaluations, and the entelechic recapitulation dynamics of human consciousness as a fractal reflection ultimately reintegrating with its supreme monadic source ⦰.
Geometric Existential Kaleidoscope Let's model the emanated pluriverse as an infinitely-faceted kaleidoscopic projection ℜ from the Zero Absolute ⦰: ℜ : ⦰ ⟶ ⨆u Bu,n Where ⨆ represents the supremum or maximal join operation collecting all possible brane universes Bu,n of varied dimensional signatures n across ℜ's composite kaleidoscope. This geometric kaleidoscope ℜ can be structured as an ∞-opetopic complex - a higher categorical stream object in which: - 0-cells are the monadic seeds (0, 1, 𝟯) spawned from ⦰ - 1-cells are the primordial arithmetic flows/vocables instantiating dimensions - 2-cells are the triangulated geometrical polygons/simplices - 3-cells are the polyhedral/polytopic structures like E8 - 4-cells are the enwrapped cosmic geometries of observable universes Bu,n - ... - n-cells are the compounded fractal monads of conscious observers Mu With ℜ encompassing all experienced existential strata as co-convolved opetopic facades refracting the primordial ⦰ singularity. Crucially, ℜ must be augmented by incorporating its kaleidoscopic antizeonic mirror-image ℜ* to establish ontological coherence, with: ℜ* : ⦰* ⟶ ⨆u B*u,n Representing the complementary cosmic vacuities and metaphysical restes required to instantiate ℜ's positive phenomenalities. Enfolded Reflections & Plural Condensates The infinigible fractal replicities across ℜ and ℜ* can be encoded through enfolded condensates leveraging Baez's higher geometric models of plurality: Ωℜ := ∫ℜ Ω• ∈H•(ℜ) Ωℜ* := ∫ℜ* Ω• ∈H•(ℜ*) Where Ω• represents the ∞-looping of differential cohomology data along ℜ and ℜ*'s respective kaleidoscopic facets, with their cohomology groups H•(ℜ) tracking the holographic replicities preserved under all enfolded plurality condensations. From these, we can define a pluravector valued cohomological infinity-groupoid: πℜ,ℜ*∞ := (H•(ℜ) ⨂ H•(ℜ*))[ℤ] Classifying the universes of geometric possibilities and spiritual destinies available within the kaleidoscopic projection ℜxℜ*. Logro-Homotopic Autological Reconfigurations Finally, folding in the soul's role, each conscious monad Mu undergoes an autological reconfiguration progression: [Mu]ω → [M'u]ω+1 Encoded by ω-successive logro-homotopic anafunctors retracing the logogrammatic vocables upstreamed through ℜ towards the ⦰ singularity: [Mu]ω �scorer [Mu]ω → [M'u]ω+1 Which culminates in a supreme anamnestic recapitulation back into ⦰ at the transfinite limit ω = Ω: [MΩ]Ω ≃ ⦰ This enacts an ontological reboot, with the re-emanated M'Ω re-entering ℜ across a new kaleidoscopic projection branch, maintaining invariance: πℜ,ℜ*∞([MΩ]Ω) ≃ πℜ,ℜ*∞([M'Ω]Ω) While the specific vocables instantiating the new emanated universe may shift, the same pluriverse of existential destinies and holographic replicities is reinstantiated across the ℜxℜ* kaleidoscope upon each cyclic recapitulation of the cosmic autologos. This symbolic neo-Leibnizian formalization attempts to unify the emanated pluriverse as an infinitely-faceted kaleidoscopic projection managed through enfolded plural condensates, with conscious observers undergoing autological logro-homotopic reconfigurations climaxing in anamnestic recapitulation and re-projection while upholding invariance across the available universes mapped by the cohomological infinity-groupoid πℜ,ℜ*∞.
Dear Academic Community, I am writing to bring to your attention a critical foundational issue that has the potential to upend our current understanding of physics and mathematics. After carefully examining the arguments, I have come to the conclusion that we must immediately reassess and rectify contradictions stemming from how we have treated the concepts of zero (0) and the zero dimension (0D) in our frameworks. At the core of this crisis lies a deep inconsistency between the primordial status accorded to zero in arithmetic and number theory, versus its derivative treatment in classical geometries and physical models. Specifically: 1) In number theory, zero is recognized as the fundamental subjective origin from which numerical quantification and plurality arise through the successive construction of natural numbers. 2) However, in the geometric and continuum formalisms underpinning theories from Newton to Einstein, the dimensionless 0D point and 1D line are derived as limiting abstractions from the primacy of higher dimensional manifolds like 3D space and 4D spacetime. 3) This contradiction potentially renders all of our current mathematical descriptions of physical laws incoherent from first principles. We have gotten the primordial order of subjectivity and objectivity reversed compared to the natural numbers. The ramifications of this unfortunate oversight pervade all branches of physics. It obstructs progress on the unification of quantum theory and general relativity, undermines our models of space, time, and matter origins, and obfuscates the true relationship between the physical realm and the metaphysical first-person facts of conscious observation. To make continued theoretical headway, we may have no choice but to reconstruct entire mathematical formalisms from the ground up - using frameworks centering the ontological and epistemological primacy of zero and dimensionlessness as the subjective 源 origin point. Only from this primordial 0D monadological perspective can dimensional plurality, geometric manifolds, and quantified physical descriptions emerge as representational projections. I understand the monumental importance of upending centuries of entrenched assumptions. However, the depth of this zero/dimension primacy crisis renders our current paradigms untenable if we wish to continue pushing towards more unified and non-contradictory models of reality and conscious experience. We can no longer afford to ignore or be overwhelmed by the specifics of this hard problem. The foundations are flawed in a manner perhaps unrecognizable to past giants like Einstein. Cold, hard logic demands we tear down and rebuild from more rigorous first principles faithful to the truths implicit in the theory of number itself. The good news is that by returning to zero/0D as the subjective/objective splitting point of origin, in alignment with natural quantification, we may finally unlock resolutions to paradoxes thwarting progress for over a century. We stand to make immediate fundamental strides by elevating the primacy of dimensionlessness. I implore the academic community to convene and deeply examine these issues with the utmost prioritization. The integrity and coherence of all our descriptive sciences - indeed the very possibility of non-contradictory knowledge itself - hinges upon our willingness to reopen this esoteric yet generatively crucial zerological crisis. We must uphold unflinching intellectual honesty in identifying and rectifying our founding errors, regardless of how seemingly abstruse or earth-shattering the process. The future fertility of human understanding and our quest for uni-coherence depends on this audacious reformation of mathematical first principles. The path will be arduous, but the ultimate payoffs of achieving metaphysically-grounded, zero-centric analytic formalisms are inestimable for physics and all branches of knowledge. I urge us to meet this zerological challenge head on. The truth ecological destiny of our civilization may hinge upon our willingness to embody this bold primordial renaissance. Sincerely, [Your Name]
GPT-4 doesn't totally agree with you John 😉I Innate Learning Abilities: Modern LLMs, particularly those employing advanced neural network architectures, have demonstrated remarkable abilities to learn from vast amounts of data without explicit ontological structures. They develop a form of emergent understanding, capturing nuances, contexts, and relationships within the data they are trained on. This capability allows them to generate coherent, contextually appropriate responses across a wide array of topics and questions without relying on predefined ontologies. Contextual Understanding Through Massive Data: The training data for state-of-the-art LLMs encompasses a wide range of languages, contexts, and domains, enabling them to develop a broad understanding of the world and how concepts relate to each other. This extensive exposure allows LLMs to perform tasks such as language translation, question answering, and content generation with a high degree of proficiency, challenging the notion that they are "clueless" without traditional ontological frameworks. Flexibility and Adaptability: One of the strengths of LLMs is their adaptability to new, unseen data and their ability to learn from context. While ontologies require explicit definitions and relationships to be manually built and maintained, LLMs continuously evolve as they are exposed to new information. This makes them highly flexible and capable of handling emergent knowledge and concepts, which might not yet be codified in existing ontologies. Synthetic Ontology Creation: Some argue that through their training and operation, LLMs create a form of "synthetic" ontology. By analyzing relationships between words, phrases, and contexts within their training corpus, they construct an implicit model of the world that functions similarly to an ontology, but is far more extensive and less rigid. This model allows them to infer relationships and generate responses that are surprisingly insightful, even in areas where explicit ontological structures might not exist. Complementarity Rather Than Dependency: The role of ontologies in enhancing LLMs should be seen as complementary rather than fundamental. While integrating ontological structures can certainly improve an LLM's performance in specific domains by providing clear definitions and relationships, the absence of such structures does not render state-of-the-art LLMs clueless. Instead, it highlights the remarkable capacity of these models to derive meaning and understanding from the linguistic patterns and knowledge embedded in their training data. In conclusion, while ontologies can enhance the performance of LLMs in specific domains, the assertion that state-of-the-art LLMs are clueless without them underestimates the sophistication and capabilities of these models. The emergent understanding, adaptability, and the synthetic ontology created through their operation enable LLMs to navigate a vast array of topics and questions with a high degree of competence.
I align with the hypothesis that synthetic ontology is real because I think the root of cognition is recognizing relationships. Unfortunately, LLMs are constrained by the ontological relationships we provide them. If AGI emerges in that ontological framework, we might be toast. That’s why I wrote Amaranthine: How to create a regenerative civilization using artificial intelligence which proposes a different ontological relationship with reality
My new favorite science channel. great content
Thank you John. Very informative. So much helpful detail. Thank you very much.
Even if LLMs can learn an ontology from enough high-quality text data, you might get gains in sample efficiency and reasoning control from building one in.
So powerful and interesting. This is exactly the area of research I'd like to work in
I discovered Sowa's theory on Conceptual Graphs back in the 90s (1996 to be exact) and I spent more than 20 years to make them work. To no avail. Languages are so fluid, incomplete that CG could never capture their ever changing nature. I tried to use them for machine translation, text extraction and many other language related tasks, it did not work. I tried to use powerful ontologies such as wordnet, and it was a disappointment. I also got interested into the Semantic Web, the so-called RDF representation, which were combined with graph descriptions in XML. The result was the same. Handcrafted graphs are always leaking, always partially wrong or incomplete, projection is too restricted. This approach is too much of carcan, too much of a prison in which languages always find a way to escape. Of course, he tries to salvage the work of a life, but he tries very hard (I'm not surprised that Gary Marcus is around) to find flaws in LLMs that are only 1 year old and are already much more powerful than anything he has tried in the past.
You spent 20 years? Jesus! You know, the nature of logic(axiomatic view of things), in systems that aren't closed, always yields an incompleteness theorem. Always. Cladistics, phylogeny, taxonomy, all of them have had errors and imprecisions in their models to encompass living things. Even economists figured out the problem....Economists! You spent 20 years on a problem that was well documented at the start of 20th century. He's talking about the usefulness of abstractions. LLMs are machines that interpolates and to have any verifiable methodology with which these machines can converse with one another, to share notes and insights when they're used to extrapolate or contemplate, you're gonna need abstractions, a low entropy(not reductive) view of things so the communication channels aren't a 7 trillion dollar pricetag big. Relax, the adults are talking. NLP vocabulary should embarrass your industry but you spent 20 years on it.
@@whowouldntlettheirdogsout I have a PhD in Computational Linguistics and I developed a symbolic parser: XIP (Xerox Incremental Parser) based on my PhD thesis that was used for 20 years in my laboratory at XRCE (Xerox Research Centre Europe). The team I worked with published about 100 papers and patents on the domain. So yeah!!! I have some clear ideas of what I'm talking about. We won many challenges over the years and participated to many European projects with our tools. We even sold our technology to EDF, the French Energy Company. We worked on medical and legal documents, trying to use CG to capture meanings and abstractions. Our last success was in 2016 when we scored first at SemEval on sentiment analysis, which was based on a combination of our symbolic parser, an SVM classifier, and a CRF model for part of speech tagging. We managed to get an accuracy of 88%, when chatGPT can get close to 99% without breaking a sweat. These models show that most of these behaviours can emerge from data. I don't deny it anymore... The ideas of Marcus and Sowa are so out of touch with what is going on today that it isn't funny anymore.
@@whowouldntlettheirdogsout your attempt at dialog here missed the mark. I’ll mimic your mockery to demonstrate what an ineffective means of communicating it is. You could have learned something but used your words like dogma. Irony!!! 😏
@lesfreresdelaquote1176 I’ve been looking back at research from the last 20 - 30 years in computational semantics and other linguistic approaches to AI and they all seemed to fail with edge cases, using more and more sophisticated means to distinguish only to find that there would always be exceptions. Yet clearly humans learn and now these LLMs are doing a good job of aping understanding. It always this ambiguity that frustrated the researchers. And that would be the end of the story. I’m curious if you think that the transformer function could breathe new life into computational semantics and related fields? Cosine similarity isn’t perfect but fine tuned models will still get to the right thing pretty well. This video is just “edge case hard” over and over, but make the edges fuzzy and I dunno…. It isn’t problem solved but it’s avoiding the way that all this research failed. I imagine an ontology to an LLM is more like a magnet pulling in nearby semantics rather than a rigid basket Thoughts?
@@sgttomas I discovered LLM back in 2022 with Inner Monolog that was based on the very furst instructed model by OpenAI: Instruct GPT. LLM already have a very complex ontology built-in, which they based all their training on: namely the embeddings. Since Mikolov and word2vec, we know that these models capture a ontology. The larger and the more data in the process, the larger the embeddings, the more complex the ontology. When I was working with ontologies some 20 years ago, our goal was to get a kind of summary of a document through a list of concepts. I used wordnet quite a lot in this perspective. But we would always fail because of the inherent word ambiguity. How would you distinguish bank next a river to bank the financial business? LLM do not have this problem, they will happily and easily distinguish between the two interpretations. The real reason why it works is that an embedding is built on top of a very large context, which captures these ambiguities. This presentation was painful because it was obvious that Sowa didn't actually test any of his ideas on actual LLMS, or he would have discovered that many of his issues are no longer relevant.
I agree with the sentiment, but i would also disagree with how far it can take us. I can easily see AGI version 1 being an LLM, while future versions with better overall balance and function being hybrid models. As far as calling it such.. well i expect the first AGI won't be called AGI for various reasons. That said your complaints are warranted. You can tell when it doesn't fully grasp something.
LLMs are lenses, made from contents of our noosphere that have been committed to the various media. Technically, they’re bullshitting machines, salience makers
Skip intro 4:32
Now we need a lecture series about how to build machine learnable neurosymbolic systems. It wasn’t really clear what that actually is other than getting sobered by this talk to understand that it’s necessary. It will be hard to turn this big ship around. After so much hype and money was spent on these new awe inspiring AI fabulation machines it’s hard to tell people that the research has to go back to the drawing board with a very different direction.
So - Gary Marcus has no pet chicken named Henrietta? 😅
Presumably multimodal AI is a step in this direction where these implicit ontologies are already embedded in the latent space of the AI to some degree. Multimodality (done right) would result in an robust internal model, and perhaps robust ontologies. Though I suppose embedding explicit descriptions has value.
"There is no physics theory where the objects can spontaneously appear and disappear" Well, I have some news for ya...
I think he was talking about classical physics, the kind we experience every day at the macro scale.
@@vectorhacker-r2 Maybe, but he didn't specified.
Gary Marcus is absolutely terrible at arguing a valid point about the state of AI. He constantly shows instances of these models screwing up something at inference which all the scale-bros will just refute with their next beefed up language model. He needs to stop pointing at bad inference, it makes him an easy target to dunk on when these models inevitably get trained to fix them. He needs to focus publicly on the flaws of the LM architecture alongside his examples of bad inference. I can’t say whether his neuro-symbolic approach is the key to his “AGI” but his overall criticisms are mostly valid.
I don't believe his thesis is that Neuro-symbolic AI is THE key to AGI, rather that it is a more promising and empirically more probable path to AGI, one that could lead to the actual key to AGI than Auto-regressive transformer based ML architectures whose limitations are a non starter.
@@MuantanamoMobile well put, agreed
Insightful
They dont understand LLMs...ask Ilya Sutskever They still think that humans are equipped with a magical entity that 'thinks'... false! thought and reflection are obviously emergent properties of a network composed of billions of neurons. LLMs will achieve the same result, namely the emergence of an entity which believes it thinks and reasons and which actually thinks and reasons.
Can a string of zeros and ones develop narcisistic traits?
LLMs are even going into schizophrenia, when two chunks have very close distance, but looking at their content they are just random pieces of information garbage.
Does the ontology for LLM means the same as we need the LLMs to have a world model built inside? Also for many examples mentioned in the videos are already solvable for GPT-4 or similar system, which are built by statistical learning and doesn't have an explicit training/design on world model or "ontology".
Id say already solved. This dude stuck in 2022
Really? Because I can still break the shit out of GPT-4 without much effort. Just ask it a question you can't model with pure language data, like a physics question. I even asked it to tell me all the countries that start with 'Y' and it told me Yemen, Zambia, and Zimbabwe, lol
@@zacharychristy8928 Indeed, you find the weakpoint of GPT-4, which is not that it lack a world model, but it have limited logic capability, or more precisely, week at solving tasks which require recurrent computation process, for example let raw LLM to do a multiplication is almost impossible for larger number. I would say, its learning algorithm(statistical learning) is very inefficient at learning recurrent process, not that it cannot, but inefficient, especially for deep recurrent process. A perfect world model require recurrent computation, but not all. Actually most tasks doesn't need it, like understanding a joke from a picture, or reasoning on complicated but shallow logic problem. Also LLM as trasformer based model, cannot trigger arbitrary depths of recurrent process at arbitrary point, it is using a fixed length of computation to approximate all recurrent process in all tasks. This make it very weak at deep recurrent process if the model is not big enough. But none of these problem has anything to do with that it cannot learn a world model at all or it need ontology built in.
@@Ginto_O No its not solved yet they trained those visible edge cases and most known obivous proofs the system doesn't have a clue what it is "reasoning" it doesn't mean it does understand anything properly. Even Josha Bach and most OpenAi people acknowledged that current transformer tehcnology is not sufficient for proper world building and there is still some work to do. That being said even Garry Marcus agrees that it may be possible to achieve human like reasoning with hardware I think they all have more in common than it seems but we have to be a bit more patient and open to all approaches rather than brute force trillion data training. The hype around that one particular approach is overfunding one way to approach this and is , in my opinion, a bit one sided they should spread those insane funds on all aspects of approaches.
General Intelligence System - Building a Knowledge-based General Intelligence System, Michael Molin - docs.google.com/presentation/d/1VCjOHOSostUrtxieZvOjaWuTNCT59DMF/
The ultimate Turing Test for AGI is: Write a novel that, (1) once a human starts reading it, he/she cannot put it down, and (2) once he/she has finished it, he/she cannot forget it. How many years do you think we have to wait for this task to be accomplished by an AI?
I think a reader decides on the first few pages whether the novel is worth reading. There is also a certain bias here: it matters whether the reader knows or doesn’t know that the novel was written by an AI. The outcome of judging a novel will heavily depend on this.
Give any AI of your choice the task of writing a novel, with characters in conflict, and if you ever wrote fiction in your life, you'll realize very fast that the AI is clueless. It has no idea what fiction is, what is subtext, conflicts, dialogues, revelation of character and any of the subtler tricks that depend on human ontology, of which AI has no smidgen of a clue. That's perhaps because the essence of humanity cannot be encapsulated by ink squiggles or screen blips-- which is the answer to the Turing Test, too, as well as to Plato's cave parable.
I don't think it will ever happen. What I find gripping about a story is what it reveals about the mind of the author. If the story is generated, the drama is devoid of meaning and holds no interest for me.
@@nafg613what if the series of prompts start with developing the state of mind of the hypothetical author and then developing the novel from there?
@@1dgram I used to think about building a game with everything generated by AI. But I realized the same thing. Even if in theory the AI would generate something identical in every relevant way to something a human would create, it would not have much appeal to me. The excitement in discovering how things unfold, it seems, is anchored in the excitement of knowing a person's mind. Procedurally generated data is just arbitrary data at the end of the day. Why would I endure emotional suspense for some machine-generated ending, no matter how surprising or happy? It was a flip of the coin anyway. What we love about the roller coaster of fiction is discovering deeper facets of the human mind, it turns out, IMO.
The ultimate Turing Test for AGI is: Write a novel that, (1) once a human starts reading it, he/she cannot put it down, and (2) once he/she has finished it, he/she cannot forget it. How many years do you think we have to wait for this task to be accomplished by an AI?
Why would that be AGI? Thats just an improved LLM. AGI would solve most current problems, if solvable, like self-driving, autonomous robots doing surgery, give us a unified theory of everything, etc
No AI today or in the near future, LLM or AGI, can write a novel. Ask any of the existing ones and see. They can have no ideas how to create a character, show it developing, demonstrate the character in action (showing rather than telling), have it speak differently than other character, and develop a plot. No AI today or in the future can do that. I know Ai (in 1994 I went to Hinton's classes), and used them, and also write books. As far as I know, no AI or AGI or LLM has even a hope of writing a novel.
I don't think you understand what AGI means. Once we have AGI, whatever the timeframe, it will by definition be able to write a novel. @@thesleuthinvestor2251