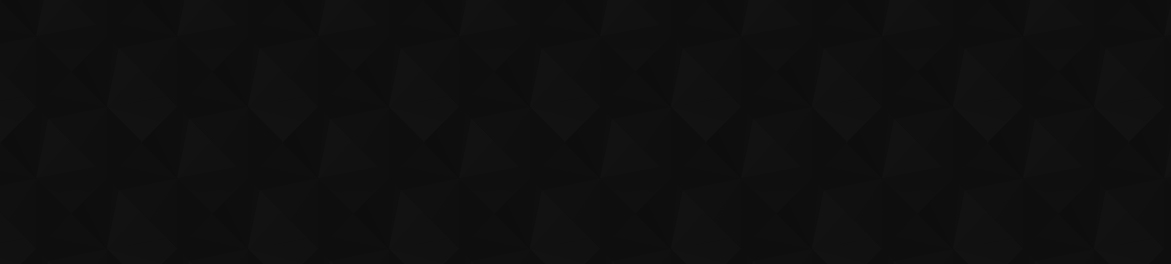
- 56
- 51 596
Bill's Neuroscience
Приєднався 16 бер 2020
Principal Component Analysis and High Dimensional Data in R. Part 3
Principal Component Analysis and High Dimensional Data in R. Part 3
Переглядів: 64
Відео
Principal Component Analysis and High Dimensional Data in R. Part 2
Переглядів 514 місяці тому
Principal Component Analysis and High Dimensional Data in R. Part 2
Principal Component Analysis and High Dimensional Data in R. Part 1
Переглядів 884 місяці тому
Principal Component Analysis and High Dimensional Data in R. Part 1
Presentations I - How to give a scientific poster
Переглядів 556Рік тому
Presentations I - How to give a scientific poster
Presentations II - How to give a scientific talk
Переглядів 126Рік тому
Presentations II - How to give a scientific talk
Biostatistics 10
Переглядів 412 роки тому
We learn about the assumption of independence, why it's so important, and what to do if we violate this assumption.
Beginners guide to Machine Learning, Deep Learning and AI
Переглядів 812 роки тому
Beginners guide to Machine Learning, Deep Learning and AI
Epilepsy 6 - Focus on Absence Epilepsy
Переглядів 1,2 тис.3 роки тому
Epilepsy 6 - Focus on Absence Epilepsy
Epilepsy 5 - Cellular mechanisms of Epilepsy
Переглядів 5413 роки тому
Epilepsy 5 - Cellular mechanisms of Epilepsy
These are really good videos! Ty
Amazing info😊
As an aspiring neuroscientist I really appreciate these videos
love this channel
hey, great work !
I wish I saw this content as a senior in high school. Starting with the simple wire characteristics is accessible
Have you ever try ilastik? If yes how do you think it is compared to others?
I've heard of it, but I've never used it, and I don't know anyone who does. But from scanning through their code base, it is being actively maintained, so that is always a good sign. Looks like it would be something useful to be familiar with, because of t's general purpose nature.
Thank you
Loved this so much.. could you please elaborate why the current in voltage clamp is in the opposite direction?
Good question. It is fundamentally just a convention. When voltage clamp papers were first being published, some people would show positive current entering the cell via ion channels (and hence being opposed by negative current being applied by the amplifier) as going up the page (i.e. positive). Other people would show it as a negative current (because that is what the amplifier actually did). The convention became to report what the amplifier did. This is because this is actually the truth. As in, if we show the current that the amplifier applied, we are truly, definitely showing what the amplifier applied. We ASSUME (or perhaps hope) that this is equal and opposite to the current flowing through ion channels, but it is never exactly accurate (and in some cases can be very far from accurate). So we stick to what we know happened, and hope it is an accurate representation of what happened at the ion channels.
thank you. wonderful video.
Thank you. Very useful for quickstarting reading papers regarding these.
thank god for you good sir 😭
Thank you for this
Agreed with the other commment. Can anyone explain why for every positive charge going into a neuron one must come out? I thought the point is there are imbalances between extra/intraceullar concentrations.
I understand the confusion. It is a very common source of difficulty. I think the confusion primarily comes what "current" means when it comes to a capacitor. But the basic answer as to "why" is because "physics". In circuit theory, it's called "Kirchhoff's Current Law", which states that the sum of all currents entering and exiting a node must equal zero, i.e. if a positive charge enters a place, then either a negative charge must enter, or a positive charge must leave. But to try to give you a mental model, imagine a tiny bubble of membrane, with a single sodium channel in the membrane. Inside our bubble there are an equal number of positive and negative charges, and the same applies outside the cell, so the transmembrane voltage is zero. The sodium channel briefly opens, and despite there being no electrochemical gradient, a Na+ ion happens to move from outside the cell, to inside the cell. The instant that happens, the electric field inside the cell changes, with the voltage inside the cell now being positive. This attracts some negative charges outside the cell to the membrane, and repels some positive charges. This net movement of charge IS a current. Indeed, it will (in total) exactly equal and opposite to the current that moved into the cell. This current is usually described as the capacitive current. While the movement of the ion through the ion channel is called the ionic current. So Iion = -Icap. i.e all the movement of charge into a via ion channels cell, ends up charging up the membrane capacitor. This rule only applies in "isopotential spheres" like out membrane bubble. But in large cells, it fundamentally still holds, it's just that there is one more place for current to flow: down dendrites and axons, i.e. axial current. There you can say: Iion = -Icap -Iaxial. I hope that helps.
Thank you very very much for these enjoyable videos.
Thank uuuuuuuuuuuuuuuuuuuu
Thanks. This is Interesting! 1:34 I know that brain waves are of four or five types. The highest being Gamma waves (30 to 80 Hz). Now, when I see something like 5000! Hz, I feel I'm missing something.
Thanks for doing this video! Great help for my exam :)
great explanation
Highly underrated channel. So glad, I came across it.
good videos, but please take good sleep
Yeah, sorry about that. I had a newborn at the time.
thanks a lot for your sharing
Absolutely enjoyed your video series!! Super clear and informative, helped me a lot with understanding terminologies, being able to interpret ephys figures, and more. Keep making videos, please!!!!
Bright enthusiastic student asks: "So is setting up an animal for 2 photon imagining fun?" Jaded neuroscientist says: "Sure its fun, fun in the same way sitting in a bath tub of tabasco sauce and shoving shards of glass up your butt is fun"
Loving these videos Bill
incredible. just incredible. you have a remarkable gift of making some of the most complex concepts sound so easy and simple. thank you so much for sharing your knowledge online for free.
isn't it True that neuralink killed more primates than most researchers?
Very helpful tutorials for beginners!
Great video.
I appreciate your explanations in all of your videos. But the stifled yawning is terrible.
Sorry about the yawns. I do feel bad about them. But I had a new born at the time. I'm going to re-record them in the second half of this year hopefully.
Great 👍
So the 2-photon or multi-photon process doesn't need any real middle energy state (k), as long as they are condensed enough and can add up, the excitation would happen?
Thank you so much for the detailed explanation..
Do you think non-parametric tests with two factors having an effect is a good area of research?
man I didnt get anything! Im sure there are ways to improve your way of speech!
I have a New Zealand accent, because I grew up in New Zealand. But if you're having trouble, turn on closed captions.
what do you mean by 'improve the speech'. the man took out time to explain you. now you want him to 'speak different'?!?!??!!?!??! audacity.
Thank you for you efforts
This is SUCH a good video. Thank you very much for posting this.
Great video
Thanks. The causality discourse you made was very useful for understanding the underlying mechanism!
Can whole-cell patch clamp be an in vivo electrophysiology technique?
Can it? Absolutely. It wasn't invented for doing that, but it's pretty common these days.
I have just added the equivalent components for the HH model through Linear Circuit. The output of each component is not something like an Action Potential. How can I get that by inserting the same component and values in the Linear Circuit section? Thanks.
Thanks for this tutorial. I need to simulate the whole neuron with the electrical circuit. Can I do this by NEURON software itself? If not, which app do you suggest me to use to simulate the neuron with circuits? Thanks
So, theoretically, you can build up an entire neuron in circuit builder, compartment by compartment. But I would strongly advise against it. Typically, one would build the neuron with Neuron Code (i.e. in a HOC file), then just import the model into circuit builder, which then just appears as a single element in the circuit. Then you can add on whatever circuit you want.
@@bilz0r Thanks, I need to do it due to variations of the components. BTW, would you mind if I ask you how I can import the model into Linear Circuit?
@@mohammadrezakhodashenaskha5006 Variation of what components? Like membrane resistance? You don't need the circuit builder for that. You get access to the soma of the cell with the second from bottom component in the Linear Circuit Builder, Arrange menu. Right now I can't remember what you do if you want access to any other component.
@@billsneuroscience2598 Thanks but I'm afraid I couldn't understand how I can get access to the cell's soma from the the second from bottom component in the Linear Circuit Builder! You mean the "Keep Connected" toggle?
@@mohammadrezakhodashenaskha5006 No, on the other side of the window. Below the resistor, capacitor... op amp. Second from the bottom.
Thanks for the video!! Quick question: What was the threshold called? Didn´t quite catch that part :)
Hi Victor, the threshold where the input rate must be above to cause LTP (or be below to cause LTD) is usually called θM or "Theta M"
@@bilz0r Thank you!
@@bilz0r Thank you!