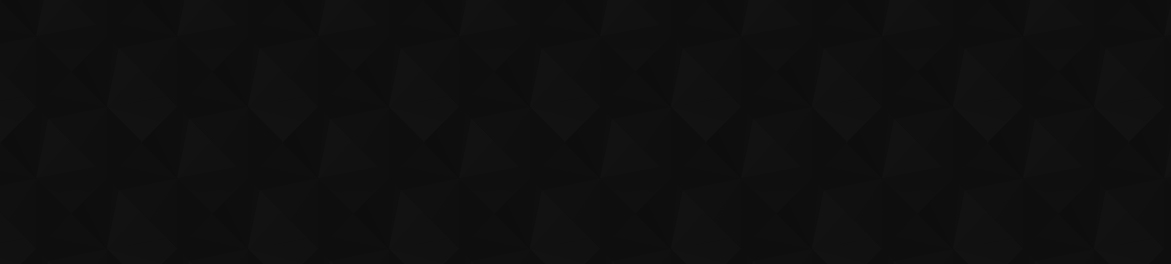
- 43
- 128 700
Ricardo Vinuesa
Sweden
Приєднався 23 січ 2021
News on artificial intelligence (AI), fluid mechanics, computational fluid dynamics (CFD) and sustainability from the VinuesaLab (www.vinuesalab.com/) at KTH Royal Institute of Technology.
More technical details in my Google Scholar: scholar.google.com/citations?user=UbyF8_oAAAAJ&hl=en
More news in my Twitter account: mobile. ricardovinuesa
More technical details in my Google Scholar: scholar.google.com/citations?user=UbyF8_oAAAAJ&hl=en
More news in my Twitter account: mobile. ricardovinuesa
Transformers for chaotic systems and fluid mechanics
Marcial Sanchis-Agudo explains us the fundamentals of transformers, and how they can be used in the context of complex physics problems such as chaotic systems and fluid-mechanics applications!
More details:
✅ www.nature.com/articles/s41467-024-45578-4
✅ arxiv.org/abs/2308.12874
More details:
✅ www.nature.com/articles/s41467-024-45578-4
✅ arxiv.org/abs/2308.12874
Переглядів: 428
Відео
Large language models for problems in Physics
Переглядів 1,5 тис.3 місяці тому
In this video by Marcial Sanchis-Agudo, we describe the self-attention mechanism which is the basis of transformers, and how it can be used for problems in physics. In particular, for temporal predictions in fluid mechanics. Check out some of our work on transformers!! ✅ www.nature.com/articles/s41467-024-45578-4 ✅ arxiv.org/abs/2308.12874
Introduction to machine learning, Part 5: The proper-orthogonal decomposition (POD)
Переглядів 1,6 тис.3 місяці тому
To conclude this first introduction series to ML, we derive and describe in detail a widely used method for analysis of high-dimensional fluid-flow systems: the proper-orthogonal decomposition (POD). Additional information in the excellent book by Steve Brunton and Nathan Kutz: www.databookuw.com/ I also acknowledge Scott Dawson for his input on this material.
Introduction to machine learning, Part 4: The Method of Snapshots
Переглядів 1,4 тис.3 місяці тому
In this part of the ML series we discuss the interpretation of left and right singular vectors and describe in detail the Method of Snapshots. We also discuss other methods related to SVD. Additional information in the excellent book by Steve Brunton and Nathan Kutz: www.databookuw.com/ I also acknowledge Scott Dawson for his input on this material.
Deep reinforcement learning for flow control in aeronautics
Переглядів 1,2 тис.4 місяці тому
In this video we provide an overview of how novel deep-reinforcement-learning (DRL) methods are currently being used in the context of flow control. These techniques have the potential to revolutionize the aeronautics industry, aiming towards more sustainable air travel.
Introduction to machine learning, Part 3: Truncated SVD and eigendecomposition
Переглядів 1,8 тис.4 місяці тому
Following our series on machine learning, here we first introduce the truncated SVD. Then we dive into the mathematical connections between the SVD and the eigendecomposition. Additional information in the excellent book by Steve Brunton and Nathan Kutz: www.databookuw.com/ I also acknowledge Scott Dawson for his input on this material.
Classifying methods in artificial intelligence
Переглядів 1,4 тис.4 місяці тому
❓Do you get confused with terms such as artificial intelligence, machine learning, deep learning or data-driven methods? ❓What is the difference between supervised, unsupervised and reinforcement learning? ✅ In this video we provide a classification of the various techniques, types of learning and we clarify their differences and similarities!!
Introduction to machine learning, Part 2: The economy singular-value decomposition (SVD)
Переглядів 1,7 тис.4 місяці тому
In this video we continue with the introductory lectures on the basics of machine learning. After discussing the full SVD, here we derive the economy SVD and discuss its applications. Additional information in the excellent book by Steve Brunton and Nathan Kutz: www.databookuw.com/ I also acknowledge Scott Dawson for his input on this material.
Introduction to machine learning, Part 1: The singular-value decomposition (SVD)
Переглядів 2,8 тис.5 місяців тому
This video starts a series of introductory lectures on the basics of machine learning. Here we introduce the widely used singular-value decomposition (SVD), we discuss its applications and start with some basic mathematical definitions. Additional information in the excellent book by Steve Brunton and Nathan Kutz: www.databookuw.com/ I also acknowledge Scott Dawson for his input on this material.
How is machine learning improving computational fluid dynamics?
Переглядів 6 тис.5 місяців тому
In this video we provide an overview of emerging trends for computational-fluid-dynamics (CFD) developments enabled by machine learning (ML)! ✅Article on perspectives: ieeexplore.ieee.org/abstract/document/10124114 ✅Review paper on ML and CFD: www.nature.com/articles/s43588-022-00264-7 ✅Article on autoencoders and transformers for reduced-order models (ROMs): www.nature.com/articles/s41467-024-...
Finding completely new turbulent structures with explainable AI
Переглядів 2 тис.6 місяців тому
🎥Here we extend our previous method using explainable deep learning from numerical data to a completely new experimental dataset. ✅As before, this objective way to assess importance reveals that the most important Q events are not necessarily the ones with the highest Reynolds shear stress!! ✅Furthermore, by applying the SHAP framework point by point, we find that the completely new, SHAP-based...
Explainable AI to study structures in turbulence
Переглядів 2,4 тис.7 місяців тому
🎥In this video we use a framework for deep-learning explainability to identify the most important Q events in a numerical channel at Retau=125. ✅This objective way to assess importance reveals that the most important Q events are not necessarily the ones with the highest Reynolds shear stress!! ✅The work is led by Andrés Cremades, see the paper here: arxiv.org/abs/2302.01250
Multi-agent reinforcement learning (MARL) versus single-agent RL (SARL) for flow control
Переглядів 3,1 тис.9 місяців тому
In this video we compare the performance of both multi-agent reinforcement learning (MARL) and single-agent RL (SARL) in the problem of heat transfer control in Rayleigh-Bénard convection. We show the potential of MARL when many agents cooperate to maximize the overall reward!! - This video is based on results from this paper: pubs.aip.org/aip/pof/article/35/6/065146/2900730/Effective-control-o...
Predicting turbulence with transformers
Переглядів 3,3 тис.9 місяців тому
In this video we explain some of the basic mechanisms of transformers, the deep-learning architectures present in the most novel large language models (LLMs). We discuss their potential to predict turbulent flows, and in particular to reproduce complex temporal dynamics. More information in these references: - ROMs and transformers: arxiv.org/abs/2304.03571 - Inflow conditions and transformers:...
Visualizing turbulence
Переглядів 2,9 тис.10 місяців тому
Can one understand some basic principles within the chaotic nature of turbulence? In this video we describe some principles to understand interactions among turbulent structures and get insight into turbulent flows!! Some interesting related material: ✅ Our work on turbulence around wings: www.sciencedirect.com/science... ✅ RANS equations and physics-informed neural networks (PINNs): pubs.aip.o...
Keynote lecture at ETC18 in Valencia, Spain
Переглядів 2 тис.11 місяців тому
Keynote lecture at ETC18 in Valencia, Spain
The building blocks of turbulence: coherent structures
Переглядів 3,8 тис.Рік тому
The building blocks of turbulence: coherent structures
Controlling turbulence through deep reinforcement learning
Переглядів 2,6 тис.Рік тому
Controlling turbulence through deep reinforcement learning
The physics of airplane wings and history of the flow
Переглядів 2,8 тис.Рік тому
The physics of airplane wings and history of the flow
Explaining turbulence through deep learning
Переглядів 1,6 тис.Рік тому
Explaining turbulence through deep learning
Introduction to deep learning for fluid mechanics
Переглядів 11 тис.Рік тому
Introduction to deep learning for fluid mechanics
Deep reinforcement learning to control Rayleigh-Bénard convection (Part 2/4: SARL controller)
Переглядів 2,3 тис.Рік тому
Deep reinforcement learning to control Rayleigh-Bénard convection (Part 2/4: SARL controller)
Deep reinforcement learning to control Rayleigh-Bénard convection (Part 4/4: coalesced cell)
Переглядів 2,2 тис.Рік тому
Deep reinforcement learning to control Rayleigh-Bénard convection (Part 4/4: coalesced cell)
Deep reinforcement learning to control Rayleigh-Bénard convection (Part 3/4: MARL controller)
Переглядів 2,3 тис.Рік тому
Deep reinforcement learning to control Rayleigh-Bénard convection (Part 3/4: MARL controller)
Deep reinforcement learning to control Rayleigh-Bénard convection (Part 1/4: baseline convergence)
Переглядів 2,7 тис.Рік тому
Deep reinforcement learning to control Rayleigh-Bénard convection (Part 1/4: baseline convergence)
Artificial intelligence and climate action
Переглядів 1,2 тис.Рік тому
Artificial intelligence and climate action
Physics-informed neural networks for fluid mechanics
Переглядів 19 тис.Рік тому
Physics-informed neural networks for fluid mechanics
Deep learning for spatial predictions in turbulent flows
Переглядів 3,6 тис.Рік тому
Deep learning for spatial predictions in turbulent flows
Seminar Ramón Pozuelo: High-performance computing and turbulence with pressure gradients
Переглядів 1,1 тис.Рік тому
Seminar Ramón Pozuelo: High-performance computing and turbulence with pressure gradients
👍👍👏👏
😮 I like it!
Great Video, Professor Venussa.
Thank you!!
Very interesting talk! Have you tried comparing the performance of your ROM in terms of both prediction time horizon and accuray with other projection-based ROMs such as operator inference? I see that the time horizon of prediction is 50 \Delta t. Is \Delta t DNS time step?
Excellent question! Yes, we made some comparisons with other methods, see here: www.nature.com/articles/s41467-024-45578-4 www.sciencedirect.com/science/article/pii/S0142727X23001534
Thank you,professor, I’ve read a lot of your papers,that’s cool!
Thank you very much!!
This is the first ever video i watched about ML for CFD and find nearly five ML techniques and the way it is implemented. Thanks
Next video please.
Brilliant, thank you.
Happy that you enjoyed it!
Great series of videos. If we have a PIV dataset which is not temporal and each velocity snapshot is u(x,y), the A matrix would define what property of the velocity field? Is it still temporal or \Phi defines variables in y direction and A defines streamwise variable? Many thanks
Just to understand better: if the dataset is not temporal, what are the different snapshots? Aren’t they taken at different instants? You can have 2D snapshots (2D modes in Phi) and then temporal coefficients ai(t). Can you explain the dataset in more detail?
@@rvinuesa Snapshots are 2D images of the velocity field taken at different instants. Each snapshot is independent of the others, and the ensemble average of the statistics is compared with the statistics of a canonical boundary layer. In this dataset, we aimed to study coherent structures of wall turbulence, such as Uniform Momentum Zones (UMZs). I wonder if we can recognize these structures using the POD method instead of histogram-based approaches. If I have just one image (no temporal sequence) and want to decompose this image using the POD method, can I recognize UMZs by selecting the largest eigenvalues?
Quite informative and very well explained. Thanks for such an amazing video !
you have very nice similar energy to Ricardo, keep it up!
Well done Marcial!
Thanks as always for the videos on machine learning!
Thanks for following the series!!
Great series. Thanks. Can you please elaborate a little bit how can we interpret the POD mode shapes? I mean by looking at the highest energy mode shape, let's say, what can we understand about the turbulent flow?
It depends on the case, but a clear example is how you can interpret the structures in the wake of a cylinder based on POD modes
Amazing series of data driven science
Thank you so much!!
Looking forward to the next video for long awaited POD details :)
I wish i was your student
Thanks a lot for sharing and taking time! Could you please share the topics you will talk about in this series? Also, do you have any plan going through enginerring optimization?
When this SVD series is finished we will have several videos on transformers. Yes, I will talk about optimization in the future!
@@rvinuesa Awesome!
Many Thanks for your valuable videos. I hope the next video is Dynamic Mode Decomposition DMD 😊
The next one is POD 🙂. DMD will come in the future!! 👌
@@rvinuesa Many thanks
I kinda already give like before watching the full video
Thats great Professor, I am joining your session on 6th May, 2024, as well. Looking to validate some case-studies in this domain.
Great to have you in the session!!
from Valencia? you keep working even on vacation, what a education focused man you are
Can you suggest what should be the learning pathway for applying ML in CFD? Suppose one is a fresh mechanical engineering graduate and is not a tremendously expert in CFD but has basic understanding of CFD but not much expertise in AI/ML?
I think it is important to have a very strong foundation in fluid mechanics and CFD. Then you can dive into ML and apply methods from the fundamental understanding. Hope this helps!
stockholm!
Nice and simple explanation. Waiting for the new videos in the series.What are the total number of videos that will be uploaded in this series?
We will probably have a couple more videos on SVD🙂
Thanks for another great video. Please make a comment on POD vs SVD in the next video !!
This is exactly the topic of a lecture coming up very soon! Stay tuned 🙂
why didn't my notification work! good to check sometimes if new videos are up
Your videos have priceless value. Could you deal more with scientific computing and numerical linear algebra (krylov subspaces, GMRES, iterative solvers, etc...)
Those are interesting topics! After the ML series I am thinking about creating one on numerics and CFD. Stay tuned!
Me encantan tus vídeos.
Muchas gracias!! 🙂
Could you recommend some important,typical paper about this?I would like to know more,thank you.
Have a look at this paper: www.nature.com/articles/s43588-022-00264-7
@@rvinuesa thank you.
interesting
Great series. Insightful. Can you please elaborate the difference between SVD and POD? Is it the same??
Good question! POD is based on the SVD algorithm. Full video on this coming up soon!!
Im going to need to watch this a couple more times
As many times as you want 😜
waited for new video
Thanks!
Thank you very much for this wonderful lecture.
Thank you!!
Looking forward to the next video and the series! Loved the use of the words "Norway" and "Madrid" for clarity, and that you mentioned the applications (not just time-space)
wow
Thank you very much for this lecture. Looking forward to following this whole series on the introduction to ML.
Fantastic!
Thankyou Professor 😃
thanks again for one of your great videos, it seems we are getting better hardware to help with your explanations even more! :D
This is amazing
Thank you so much!
Amazing!
Thank you!!
Such an informative video! 👍
Thank you!
Thanks for the effort! May I please know what CFD and Turbulence model were used?
You can find the details of the simulation here: www.cambridge.org/core/journals/journal-of-fluid-mechanics/article/an-adversepressuregradient-turbulent-boundary-layer-with-nearly-constant-beta-simeq-14-up-to-retheta-simeq-8700/AF766FD668168304FCBAC66369AE7F5F The code is based on a Fourier-chebyshev discretization. The resolution is almost DNS, with a very small stabilizing forcing acting on the smallest scales
Hello Ricardo, I started working in same field. DRL for Active Flow Control. Looking forward to connect and learn this case-study. Thanks
Would you make a video about how to create a dataset for fluid mechanics or turbulence?
That’s a good suggestion! In the meantime, we have some videos in the channel about turbulence simulations obtained via DNS
Sehr gut
The part where you are calculating SHAP values using linear combinations could be justified even more. Some bounds on * How many modes to approximate the error? * How many coalitions would give sufficiently good estimate of SHAP? are good mathematical problems to study. Also SHAP here is being applied to the fluctuations. We could equally do this to another metric, say the Reynolds stress components. Overall, this is a good idea to start looking into as a flow field metric in subsequent studies
Thanks for the comments, some answers: 1) These would not be modes, but rather individual features. We have around 200 per field and several thousands of fields 2) Typically on the order of 10 per structure
Note that we do it for the instantaneous structures of intense Reynolds shear stress
thank you for this @!
thanks again for your video