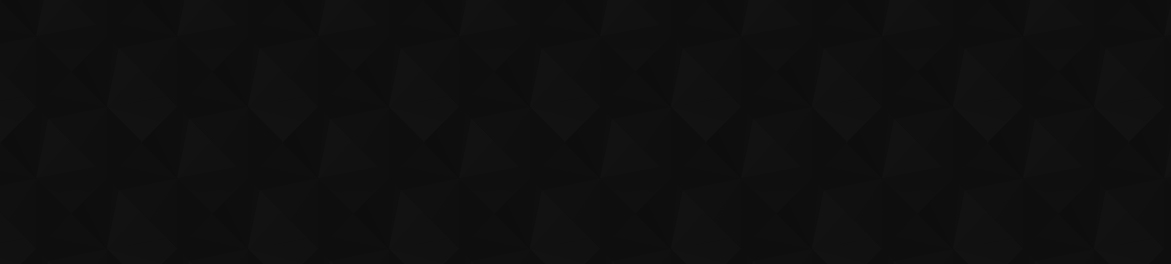
- 139
- 99 892
Tecton
United States
Приєднався 18 жов 2020
Tecton solves the hardest part of Machine Learning - the data. Tecton empowers data scientists and engineers to connect to existing data sources, build features collaboratively, deploy them to production instantly, and serve them for model training and real-time prediction. Learn more at: www.tecton.ai/
Building Plaid's ML Fraud Detection Application, Signal
Watch this virtual fireside chat to explore best practices in designing and deploying ML fraud detection applications.
Our guest speaker, Renault Young, a Software Engineer at Plaid, joined Kevin Stumpf, CTO and Co-founder of Tecton, to do a deep dive into Renault's experiences in designing and developing Plaid’s ML infrastructure for Signal, Plaid's payment fraud detection and prevention application.
In this chat, Renault and Kevin discussed:
How the Plaid team set up the data foundations they needed to start building an ML platform
The technical challenges they faced in developing the Signal application, including how they solved for out-of-order transaction data for billions of bank transactions around the world
How they use On-Demand Feature Views to look for patterns in transaction data to prevent fraudulent transactions in real time
The benefits derived from this new architecture-such as improved cost management, and better workspace and access controls-along with a look into future optimizations
Our guest speaker, Renault Young, a Software Engineer at Plaid, joined Kevin Stumpf, CTO and Co-founder of Tecton, to do a deep dive into Renault's experiences in designing and developing Plaid’s ML infrastructure for Signal, Plaid's payment fraud detection and prevention application.
In this chat, Renault and Kevin discussed:
How the Plaid team set up the data foundations they needed to start building an ML platform
The technical challenges they faced in developing the Signal application, including how they solved for out-of-order transaction data for billions of bank transactions around the world
How they use On-Demand Feature Views to look for patterns in transaction data to prevent fraudulent transactions in real time
The benefits derived from this new architecture-such as improved cost management, and better workspace and access controls-along with a look into future optimizations
Переглядів: 7
Відео
Building a Real Time Fraud Detection System at Signifyd 1
Переглядів 71Місяць тому
Signifyd, an e-commerce fraud detection platform, uses machine learning and huge amounts of data to make instantaneous decisions on whether transactions-such as returns, chargebacks, and customer account creation-are fraudulent. In this webinar, guest speaker, Nathan Ackerman, Senior VP of Engineering at Signifyd, and Kevin Stumpf, CTO and Co-founder of Tecton, dive into Signifyd’s fraud detect...
Production Embeddings for Business Critical Predictive & Generative AI
Переглядів 18Місяць тому
Embeddings-condensed, rich representations of unstructured data-have revolutionized predictive and generative AI. However, productionizing these embeddings for critical business AI systems at scale is complex. In this webinar, we’ll show how embeddings can significantly enhance AI applications, enabling more accurate predictions and dynamic generative models. We'll tackle the main challenges of...
Productionize AI ML Faster, and with Less Tech Debt
Переглядів 44Місяць тому
If your AI/ML projects are moving too slowly, you might be like the 1000s of other ML teams out there who see the biggest culprit to dev velocity being the complex data engineering involved. If building, managing, and maintaining feature pipelines is tieing you and your team down, you’ll want to check out this talk. In this talk, David Wang and Sergio Ferragut at Tecton will present the data en...
Full RAG A Modern Architecture of Hyperpersonalization
Переглядів 40Місяць тому
Achieving next-level AI-powered personalization with traditional RAG (Retrieval-Augmented Generation) alone is tough, especially when it comes to adding real-time and user-specific context. Mike Del Balso, CEO and Co-Founder of Tecton, has a better way: In this talk, he introduces "Full RAG", an evolution of the traditional RAG architecture that incorporates real-time and user-specific data to ...
Powering Millions of Real Time Rankings at GetYourGuide
Переглядів 27Місяць тому
Delivering millions of personalized rankings daily is no easy feat, especially when it comes to incorporating real-time user behavior and ensuring model performance at scale. In this session, Mihail Douhaniaris and Martin Jewell from GetYourGuide share their journey of building a cutting-edge ranking system that incorporates real-time user behavior and ensures model performance at scale using T...
Building a Resilient, Real Time Fraud System at Block
Переглядів 53Місяць тому
-Implementing effective fraud detection in a complex, evolving ecosystem like Block’s is challenging, especially when it comes to real-time analysis and adapting to new fraud patterns. Niccolò Ronchetti, Staff Machine Learning Engineer at Block, presents an innovative approach: In this talk, he introduces a Bayesian-based approach for fraud detection at Block, designed to combat fraud across th...
Tecton 2024 Launch Event What's New and Coming Next
Переглядів 95Місяць тому
Join us for an exclusive unveiling of Tecton's major platform expansion, where our founders, Mike Del Balso, CEO, and Kevin Stumpf, CTO, will talk about Tecton's latest innovations and showcase how we're extending our capabilities to power both predictive ML and generative AI applications. Learn how Tecton is solving core challenges in AI development: from increasing access to enterprise-wide d...
Supercharge Production AI with Features as Code
Переглядів 16Місяць тому
Data is the backbone of AI/ML systems, but it is often the bottleneck in the development process. Data scientists and engineers grapple with the complexity of building and maintaining feature pipelines, ensuring consistency, data freshness, and achieving real-time performance. In this talk, Sergio Ferragut, Principal Developer Advocate, will explore how Tecton's declarative framework empowers d...
Real Time ML for Enhanced Customer Experiences at Varo Bank
Переглядів 22Місяць тому
In fintech, data is the cornerstone of innovation and customer-centric AI solutions. In this technical deep dive, Tim Hopper, Staff Software Engineer, Machine Learning on Varo Bank's ML platform team, presents how Varo streamlined their approach to productionizing data, driving AI/ML initiatives across multiple products and consumer experiences. Tim will delve into Varo's journey of democratizi...
Building Personalized Insurance Pricing with Real Time AI at Prima
Переглядів 25Місяць тому
Building and maintaining a real-time AI system for personalized insurance quotes presents significant challenges, particularly when it comes to maintaining feature consistency across environments, managing complex real-time pipelines, and ensuring system reliability for mission-critical pricing models. Prima, a leading Italian insurtech company, tackled these challenges head-on in their evoluti...
Machine Learning at Atlassian with Tecton
Переглядів 117Місяць тому
See how Atlassian, the software powerhouse behind Jira and Confluence, supercharged their ML workflow with Tecton. Join Joshua Hanson, Principal Engineer at Atlassian, as he reveals: How they slashed feature development time from months to just ONE DAY 🚀 The secret to serving MILLIONS of ML requests effortlessly 💪 The key to 99% model accuracy in production ML 🎯 // Sign up for the newsletter ww...
Machine Learning at Coinbase with Tecton
Переглядів 73Місяць тому
See how Coinbase, the crypto giant, revolutionized their ML workflow with Tecton. Join Joe McAllister, Senior Engineer at Coinbase, as he reveals: How the ML team doesn't waste time doing data engineering 🚀 Their secret to RAPID feature iteration with ease 💪 How Coinbase prevents fraud INSTANTLY ⚡ // Sign up for the newsletter www.tecton.ai/newsletter/ // Try Tecton www.tecton.ai/explore/ // Su...
Tecton 1.0 Launch
Переглядів 215Місяць тому
Check out this launch video to learn about Tecton's platform expansion in their 1.0 release on 9/17/24. It's all about providing a super simple dev experience to power GenAI and predictive ML applications with rich, contextual data. // Make AI applications smarter with tecton.ai // Sign up for the newsletter www.tecton.ai/newsletter/ // Try Tecton www.tecton.ai/explore/ // Subscribe to @tecton...
Making LLMs smarter with better context…a real PITA?
Переглядів 1732 місяці тому
LLMs need more than a simple RAG architecture with similarity search. They would be so much more useful for businesses if they could have access to rich, contextual data. // But it shouldn’t be this hard. // Make GenAI applications smarter with tecton.ai // Sign up for the newsletter www.tecton.ai/newsletter/ // Try Tecton www.tecton.ai/explore/ // Subscribe to @tecton8241
The BIGGEST blocker to building AI…why projects get stalled
Переглядів 19 тис.2 місяці тому
The BIGGEST blocker to building AI…why projects get stalled
Introducing Tecton and Google Cloud Platform (GCP)
Переглядів 305Рік тому
Introducing Tecton and Google Cloud Platform (GCP)
apply(risk) 2023 | Access Control in ML Feature Platforms
Переглядів 187Рік тому
apply(risk) 2023 | Access Control in ML Feature Platforms
apply(risk) 2023 | Fraud Prevention Best Practices In ML Observability & Emerging Approaches
Переглядів 208Рік тому
apply(risk) 2023 | Fraud Prevention Best Practices In ML Observability & Emerging Approaches
apply(risk) 2023 | Challenges at the Intersection of ML & Real-Time Data: Lessons Learned
Переглядів 141Рік тому
apply(risk) 2023 | Challenges at the Intersection of ML & Real-Time Data: Lessons Learned
apply(risk) 2023 | Fighting Financial Crime With Machine Learning at Tide
Переглядів 188Рік тому
apply(risk) 2023 | Fighting Financial Crime With Machine Learning at Tide
apply(risk) 2023 | A Decade of Risk Machine Learning: Some Lessons Learned
Переглядів 239Рік тому
apply(risk) 2023 | A Decade of Risk Machine Learning: Some Lessons Learned
apply(risk) 2023 | Solving Twitter's Bot Problem With Less Than 10 Lines of Code
Переглядів 134Рік тому
apply(risk) 2023 | Solving Twitter's Bot Problem With Less Than 10 Lines of Code
apply(risk) 2023 | Powering ML Fraud Detection Models With Advanced Aggregations
Переглядів 557Рік тому
apply(risk) 2023 | Powering ML Fraud Detection Models With Advanced Aggregations
apply(risk) 2023 | How to Build a Real-Time Fraud Detection Application With a Feature Platform
Переглядів 2,2 тис.Рік тому
apply(risk) 2023 | How to Build a Real-Time Fraud Detection Application With a Feature Platform
apply(risk) 2023 | Fighting Fraud with Machine Learning at Remitly
Переглядів 526Рік тому
apply(risk) 2023 | Fighting Fraud with Machine Learning at Remitly
apply(recsys) Conference 2022 | Workshop: Choosing Feast or Tecton for Your RecSys Architecture
Переглядів 1 тис.Рік тому
apply(recsys) Conference 2022 | Workshop: Choosing Feast or Tecton for Your RecSys Architecture
Wish the guest had shared some architectural pointers. It is no where on the website too.
Excellent
Whats this?
Excited
Tecton, ur content makes me happy
This was a great demo, thanks!
great but why so short
can i get the source code of these project if you dont mind
That concept of silos applies to python and r, python users don’t talk methodologies, they only see programming…m people of r only concern about methodology, not programming aspects…, everyone its working actually in silos…
Too fast explanation !
Is Monolith compatible with PyTorch also?
Great video! Any demos on how to materialize stream and real time data?
Hi, back again with one more question. How does FEAST handle realtime data? Does it register it straight to FEAST upon user interaction?
Amaizng talk! I've two questions from watching. 1. Is it easy to transition from Feast to Tecton? I want to start small then expand functionalities. 2. In Feast's doc, it says it is not for super low latency. It says it cannot guarantee p99 feature retriecal latency to be less than 10ms. Does Tecton reducs latency?
Hi, does feast support python 3.6?
Wish you would have talked more about the orders.csv and how the entity IDs are important for the training model on getting the features from the feature store. Also wish you would have mentioned that the feature store object can be stored/fetched online instead of just locally from the particular local repo.
Thanks for sharing your Real time and Batch Architectures!
Excellent video and very easy to follow...thank you!
Frances Wang is asking me about this. the lady is kind of my hero. I want to give her as much information about my experience as possible.
Btw: Transaction Processing Performance Council (TPC)
Man, I wish ScyllaDB was considered in this mix! Great talk, though.
You explained so well, and made it easy to understand thank you, Barr!
The diagrame in 7:18 do not consider streaming pipleine
?Ř????ϻ
Are there bookmarks for the different segments and talks?
currently uploading the individual videos now!
Conversation starts at 43:30
Can you share the repo that was presented in the video. It will be quite helpful.
Nice video on Data observability.👍
8sxft vyn.fyi
9jdbj vun.fyi
This was an interesting talk.. deserves more likes 👏🏻
@stepan Can you share the aws configurations if you don't mind in somewhere
Is there somewhere we can access the slides from this?
Thanks all that was a great time!
Hi! Where can we find the MLOps community?
They are at MLOps.Community