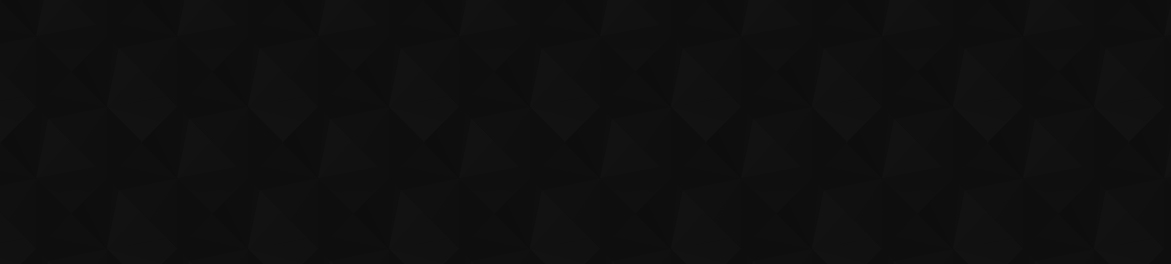
- 179
- 42 003
ACM SIGMETRICS
Приєднався 1 тра 2020
Welcome to the official UA-cam channel of ACM SIGMETRICS!
SIGMETRICS is the ACM Special Interest Group (SIG) for the computer systems performance evaluation community.
SIGMETRICS promotes research in performance analysis techniques as well as the advanced and innovative use of known methods and tools. It sponsors conferences, such as its own annual conference (SIGMETRICS), publishes a newsletter (Performance Evaluation Review), and operates a mailing list linking researchers, students, and practitioners interested in performance evaluation.
SIGMETRICS is the ACM Special Interest Group (SIG) for the computer systems performance evaluation community.
SIGMETRICS promotes research in performance analysis techniques as well as the advanced and innovative use of known methods and tools. It sponsors conferences, such as its own annual conference (SIGMETRICS), publishes a newsletter (Performance Evaluation Review), and operates a mailing list linking researchers, students, and practitioners interested in performance evaluation.
Rising Star Award Talk - Prof. Giulia Fanti
From Epidemics to Blockchains: The Delicate Interplay between Networks and Distributed Systems
Переглядів: 377
Відео
Keynote Talk - Prof. Nick Duffield
Переглядів 1382 роки тому
Inspiring Data Science Research through Collaboration with University Operations
Keynote Talk - Prof. Milind Tambe
Переглядів 1462 роки тому
AI for Social Impact: Results from Deployments for Public Health and Conservation
Achievement Award Talk - Prof. Balaji Prabhakar
Переглядів 3362 роки тому
The Unreasonable Effectiveness of Mathematical Methods in the Analysis of Networked Systems
8B4 One Proxy Device Is Enough for Hardware-Aware Neural Architecture Search
Переглядів 872 роки тому
8B4 One Proxy Device Is Enough for Hardware-Aware Neural Architecture Search
8B3 A Formalism of DNN Accelerator Flexibility
Переглядів 1322 роки тому
8B3 A Formalism of DNN Accelerator Flexibility
8B2 A Comprehensive Empirical Study of Query Performance Across GPU DBMSes
Переглядів 642 роки тому
8B2 A Comprehensive Empirical Study of Query Performance Across GPU DBMSes
8B1 CachePerf: A Unified Cache Miss Classifier via Hybrid Hardware Sampling
Переглядів 1812 роки тому
8B1 CachePerf: A Unified Cache Miss Classifier via Hybrid Hardware Sampling
7B4 Data-Driven Network Path Simulation with iBox
Переглядів 682 роки тому
7B4 Data-Driven Network Path Simulation with iBox
7B3 Fusing Speed Index during Web Page Loading
Переглядів 482 роки тому
7B3 Fusing Speed Index during Web Page Loading
7B2 Power of Bonus in Pricing for Crowdsourcings
Переглядів 372 роки тому
7B2 Power of Bonus in Pricing for Crowdsourcings
7B1 YourAdvalue: Measuring Advertising Price Dynamics without Bankrupting User Privacy
Переглядів 332 роки тому
7B1 YourAdvalue: Measuring Advertising Price Dynamics without Bankrupting User Privacy
7A3 Unleashing the Power of Paying Multiplexing Only Once in Stochastic Network Calculus
Переглядів 1072 роки тому
7A3 Unleashing the Power of Paying Multiplexing Only Once in Stochastic Network Calculus
7A2 Age-Dependent Differential Privacy
Переглядів 1112 роки тому
7A2 Age-Dependent Differential Privacy
7A1 Free2Shard: Adversary-resistant Distributed Resource Allocation for Blockchains
Переглядів 572 роки тому
7A1 Free2Shard: Adversary-resistant Distributed Resource Allocation for Blockchains
6B4 NURA: A Framework for Supporting Non-Uniform Resource Accesses in GPUs
Переглядів 382 роки тому
6B4 NURA: A Framework for Supporting Non-Uniform Resource Accesses in GPUs
6B3 Data Convection: A GPU-Driven Case Study for Thermal-Aware Data Placement in 3D DRAMs
Переглядів 212 роки тому
6B3 Data Convection: A GPU-Driven Case Study for Thermal-Aware Data Placement in 3D DRAMs
6B1 Towards Efficient Sparse Matrix Vector Multiplication on Real Processing-in-Memory Architectures
Переглядів 662 роки тому
6B1 Towards Efficient Sparse Matrix Vector Multiplication on Real Processing-in-Memory Architectures
6A4 Learning To Maximize Welfare with a Reusable Resource
Переглядів 632 роки тому
6A4 Learning To Maximize Welfare with a Reusable Resource
6A3 Stationary Behavior of Constant Stepsize SGD Type Algorithms: An Asymptotic Characterization
Переглядів 642 роки тому
6A3 Stationary Behavior of Constant Stepsize SGD Type Algorithms: An Asymptotic Characterization
6A2 Robustness and Consistency in Linear Quadratic Control with Untrusted Predictions
Переглядів 472 роки тому
6A2 Robustness and Consistency in Linear Quadratic Control with Untrusted Predictions
6A1 Asymptotic Convergence Rate of Dropout on Shallow Linear Neural Networks
Переглядів 492 роки тому
6A1 Asymptotic Convergence Rate of Dropout on Shallow Linear Neural Networks
5B4 The First 5G-LTE Comparative Study in Extreme Mobility
Переглядів 742 роки тому
5B4 The First 5G-LTE Comparative Study in Extreme Mobility
5B3 Argus: Predictable Millimeter-Wave Picocells with Vision and Learning Augmentation
Переглядів 622 роки тому
5B3 Argus: Predictable Millimeter-Wave Picocells with Vision and Learning Augmentation
5B2 NG-Scope: Fine-Grained Telemetry for NextG Cellular Networks
Переглядів 1292 роки тому
5B2 NG-Scope: Fine-Grained Telemetry for NextG Cellular Networks
5B1 A Detailed Look at MIMO Performance in 60 GHz WLANs
Переглядів 692 роки тому
5B1 A Detailed Look at MIMO Performance in 60 GHz WLANs
5A4 Sequential Fair Allocation: Achieving the Optimal Envy-Efficiency Tradeoff Curve
Переглядів 1872 роки тому
5A4 Sequential Fair Allocation: Achieving the Optimal Envy-Efficiency Tradeoff Curve
5A3 Real-time Bidding for Time Constrained Impression Contracts in First and Second Price Auctions
Переглядів 552 роки тому
5A3 Real-time Bidding for Time Constrained Impression Contracts in First and Second Price Auctions
5A2 Online Caching Networks with Adversarial Guarantees
Переглядів 392 роки тому
5A2 Online Caching Networks with Adversarial Guarantees
In the example, at 39:09, there is a mistake regarding the last interval [t3, t4] of h(tj|x) because the discrete hazard should not be greater than 1, as it represents a probability.
Best introduction video that I have found!
Excellent
This was a great start man❤
Thanks so much for this clear explanation 🎉
thks for sharing
Good to see an unbiased and down to earth analysis of current solutions. Sadly the paper is paywalled.
Great Lecture!
Great video with beautiful explanation. Thank you for your valuable time sir.
Thank you for a good explanation, but other sources say that Zx - the Page Matrix - must be an Lx[T-L] Hankel matrix constructed from the original sequence rather than just the same sequence reshaped into Lx[T/L]. In other words, it must have the i'th column consisting of elements from X1(i) to X1(i + L) - not from X1(i*L) to X1(i*L+L). So, which is correct?
Very good.
Great
Thank you
How does this compare to Empirical Dynamic Analysis?
What to do with high censored rates/data in a "narrowed" down subset?
Another thing from third parties: Titanic is a hoax join da resistance
😜 𝚙𝚛𝚘𝚖𝚘𝚜𝚖
No wonder this guy is from CMU, great content, and clear explanation.
thanks!
Great presentation, thank you!
Thank you for your research .. I am conducting research into counterfeit cryptocurrency an this video helped me immensely
h28 vum.fyi
tremendous and helpful .great job!
great job ... helpful for us .sir keep it up......
Good Job
Perspicuous!
What a crisp and clear presentation. Nicely done!
Nice lucid presentation, thank you. When you "leave one out" for cross-validation, are you filling in gaps (like predicting Q3 numbers if you know Q1,Q2,Q4), or exclusively predicting into the future (like predicting Q4 if you know Q1,Q2,Q3) ?
Hi Ymir - thanks for your note. We're using all permutations for cross validation - but the results are the same if done sequentially (but of course fewer test samples)
Great work!
This presentation summarizes a research study on Edge Intelligence conducted at the Vienna University of Technology and the University of Ottawa. It is accepted for publication at the ACM SIGMETRICS 2020 conference as well as Proceedings of the ACM on Measurement and Analysis of Computing Systems. Table of Contents: 00:25 Use case scenario 04:19 Edge data analytics 05:36 Research goal 06:18 Staleness control problem 09:04 SCEDA algorithm 12:53 Evaluation 18:57 Key points ACM Reference Format: Atakan Aral, Melike Erol-Kantarci, and Ivona Brandić. 2020. Staleness Control for Edge Data Analytics. Proc. ACM Meas. Anal. Comput. Syst. 4, 2, Article 38 (June 2020), 24 pages. doi.org/10.1145/3392156 Atakan Aral, Melike Erol-Kantarci, and Ivona Brandić. 2020. Staleness Control for Edge Data Analytics. In ACM SIGMETRICS / International Conference on Measurement and Modeling of Computer Systems (SIGMETRICS ’20 Abstracts), June 8-12, 2020, Boston, MA, USA. ACM, New York, NY, USA, 2 pages. doi.org/10.1145/3393691.3394219 Abstract: A new generation of cyber-physical systems has emerged with a large number of devices that continuously generate and consume massive amounts of data in a distributed and mobile manner. Accurate and near real-time decisions based on such streaming data are in high demand in many areas of optimization for such systems. Edge data analytics bring processing power in the proximity of data sources, reduce the network delay for data transmission, allow large-scale distributed training, and consequently help meeting real-time requirements. Nevertheless, the multiplicity of data sources leads to multiple distributed machine learning models that may suffer from sub-optimal performance due to the inconsistency in their states. In this work, we tackle the insularity, concept drift, and connectivity issues in edge data analytics to minimize its accuracy handicap without losing its timeliness benefits. To this end, we propose an efficient model synchronization mechanism for distributed and stateful data analytics. Staleness Control for Edge Data Analytics (SCEDA) ensures the high adaptability of synchronization frequency in the face of an unpredictable environment by addressing the trade-off between the generality and timeliness of the model. Making use of online reinforcement learning, SCEDA has low computational overhead, automatically adapts to changes, and does not require additional data monitoring. Contact Author: Dr. Atakan Aral Vienna University of Technology (TU Wien) Institute of Information Systems Engineering atakan.aral@tuwien.ac.at | +43-1-58801-188734 | rucon.ec.tuwien.ac.at