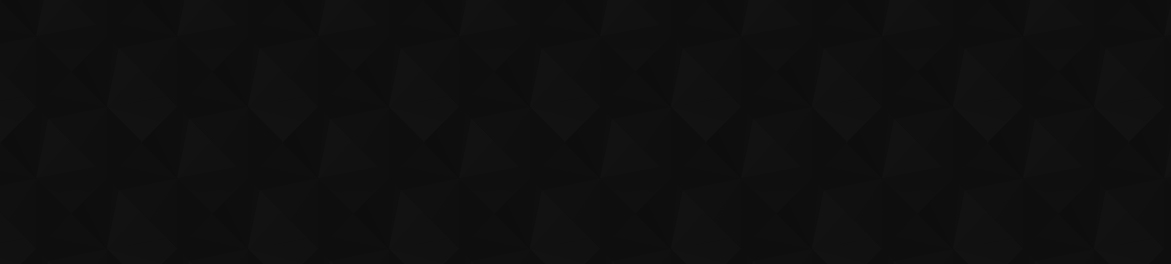
- 7
- 6 828
Lore Van Oudenhove
Belgium
Приєднався 3 чер 2012
The Ultimate AI Agent Guide: How I Built & Deployed an AI Agent with LangGraph, LangServe & AWS
In this tutorial, I’ll guide you through building and deploying a customer support chatbot for a solar panel company using LangGraph, LangServe, and AWS Copilot. This video provides a complete, hands-on approach to creating, testing, and deploying an AI agent within an API that can seamlessly integrate with any frontend application. Your chatbot will be ready to handle real customer inquiries, from maintenance tips and service scheduling to calculating energy savings, helping customers maximize their solar energy benefits.
👩🏫 What You'll Learn:
- Core concepts of LangGraph and LangServe for building and deploying AI agents
- Setting up a complete AI agent workflow using LangChain and LangGraph
- Techniques for integrating Tavily Websearch and AWS Bedrock
- Best practices for using LangServe to seamlessly deploy AI agents on AWS Copilot
By the end, you’ll have the skills to deploy powerful, real-world AI agents that deliver practical solutions for customer support!
If you find this video helpful, don’t forget to give it a thumbs up and subscribe to my channel for more AI tutorials!
📚 Resources
GitHub Repo with code: github.com/Pairrot-Lore/ai-agent-deploy.git
UA-cam tutorial with details on the AI agent development: ua-cam.com/video/oF6pajb-lsY/v-deo.html
Medium article detailing AI agent deployment: To be released soon!
Medium article with details on the AI agent development: medium.com/@lorevanoudenhove/how-to-build-ai-agents-with-langgraph-a-step-by-step-guide-5d84d9c7e832
🔗 Connect
Instagram: @lorevanoudenhove.ai
LinkedIn: Pairrot
Threads: @lorevanoudenhove.ai
Medium: @lorevanoudenhove
🚀 Visit My Website: www.pairrot.eu/
🤖 Tags: AI, LangGraph, LangServe, LangChain, AWS Bedrock, AI agent
Timestamps
Music by Bensound.com
License code: ZZAZMMAHQHTZG4Z8
👩🏫 What You'll Learn:
- Core concepts of LangGraph and LangServe for building and deploying AI agents
- Setting up a complete AI agent workflow using LangChain and LangGraph
- Techniques for integrating Tavily Websearch and AWS Bedrock
- Best practices for using LangServe to seamlessly deploy AI agents on AWS Copilot
By the end, you’ll have the skills to deploy powerful, real-world AI agents that deliver practical solutions for customer support!
If you find this video helpful, don’t forget to give it a thumbs up and subscribe to my channel for more AI tutorials!
📚 Resources
GitHub Repo with code: github.com/Pairrot-Lore/ai-agent-deploy.git
UA-cam tutorial with details on the AI agent development: ua-cam.com/video/oF6pajb-lsY/v-deo.html
Medium article detailing AI agent deployment: To be released soon!
Medium article with details on the AI agent development: medium.com/@lorevanoudenhove/how-to-build-ai-agents-with-langgraph-a-step-by-step-guide-5d84d9c7e832
🔗 Connect
Instagram: @lorevanoudenhove.ai
LinkedIn: Pairrot
Threads: @lorevanoudenhove.ai
Medium: @lorevanoudenhove
🚀 Visit My Website: www.pairrot.eu/
🤖 Tags: AI, LangGraph, LangServe, LangChain, AWS Bedrock, AI agent
Timestamps
Music by Bensound.com
License code: ZZAZMMAHQHTZG4Z8
Переглядів: 527
Відео
Master AI Agent Development with LangGraph Studio
Переглядів 1,7 тис.Місяць тому
In this tutorial, I’ll walk you through the process of building AI agents using LangGraph and LangGraph Studio. Whether you're an AI engineer or someone looking to explore AI workflows, this video will provide step-by-step guidance on creating and testing AI agents efficiently. What You'll Learn: - Basic concepts of LangGraph: nodes, edges, and state - How to set up a LangGraph AI agent workflo...
How to build an AI chatbot using LangChain, Claude, and PineCone
Переглядів 5453 місяці тому
In this tutorial, I'll walk you through the process of building an AI chatbot using LangChain, Claude by Anthropic, PineCone, and AWS Bedrock. Whether you're a beginner or just looking to expand your AI skills, this video will guide you step-by-step on how to create a chatbot that can interact with your data locally. What You'll Learn: - How to set up a Python environment for AI development. - ...
How to build a custom chatbot API using AWS?
Переглядів 1867 місяців тому
🤖🚀Unlock the power of enterprise-scale AI agents in this comprehensive tutorial! Discover how to build an API for a custom AI chatbot using AWS Bedrock and Serverless. 📚 Resources github.com/Pairrot-Lore/chatbot-aws-bedrock-voiceflow medium.com/@lorevanoudenhove/how-to-build-enterprise-scale-generative-ai-agents-with-aws-bedrock-a-comprehensive-guide-a8b643cd97d4 🔗 Connect lorevan...
Content generatie met ChatGPT en custom GPTs
Переглядів 428 місяців тому
In het digitale tijdperk vormen custom GPT's, beter bekend als AI Agents, een revolutionaire oplossing om de efficiëntie binnen je onderneming te verhogen. Deze AI Agents worden zo ontwikkeld dat ze de unieke identiteit en waarden van je brand uitstralen. Je kunt AI Agents programmeren om bepaalde taken, zoals het maken van content, keer op keer op dezelfde wijze uit te voeren. Zo ben je verzek...
Learn To Build Custom Trained AI ChatBots (2023 Botpress Tutorial)
Переглядів 327Рік тому
🤖🚀Unlock the power of AI chatbots in this comprehensive tutorial! Discover how to create a personalized chatbot using the innovative Botpress platform. Elevate your website's conversion rates as we guide you through the process. The chatbot is designed to proficiently address customer inquiries while efficiently gathering essential information. Join us on this journey to AI chatbot mastery! 🔗 C...
How to build custom chatbots using Langchain and Weaviate
Переглядів 3,5 тис.Рік тому
Unlocking the Power of Conversational AI: Building Custom Chatbots with Langchain and Weaviate 🚀 In the rapidly evolving landscape of technology, the demand for innovative ways to enhance customer engagement and streamline operations has never been greater. Enter the world of AI-powered chatbots - the ultimate solution to automate conversations and personalize interactions. While OpenAI's Chat...
Thanks. How to stream the result back ? Now its collecting and sending as one response.
Thanks for the demo. For deploying to aws, which components it used. Is this solution is scalble to handle large traffic
Thanks
Very good tutorial.
@@alexramos587 Thanks! 👊
Very creative. Always learn something new.
Thank you! 🙏
Hello 13:00 What code statement(s) are generating the prompt inputs seen in your UI (which you are typing into)?. As you answer, assume others are not running on Apple devices. It's not clear in LangGraph here.
If you want to invoke the agent from CLI, you can use graph.stream({"messages": ("user", question)}). You can find more details on it in my Medium article medium.com/@lorevanoudenhove/how-to-build-ai-agents-with-langgraph-a-step-by-step-guide-5d84d9c7e832. 😊
This is a great video! Really helpful for getting started. I appreciate you going over the tools required (Pinecone, AWS) to actually train the model. I’m still working on collecting my corpus, but do you suggestions on ways to productionize a mode, outside of a Jupyter Notebook, perhaps how to host it on a simple website? I want to share my model for others to use and evaluate the responses!
Hey Katherine! That's a great question! Thy way I usually productionize my chatbots is by wrapping them in an API using LangServe and interacting with them via Voiceflow (they provide website integration out-of-the-box). I described this strategy in one of my Medium articles: medium.com/@lorevanoudenhove/production-ready-chatbots-with-langchain-langserve-pinecone-and-aws-e65a00e832e3. I hope this helps! 🙂
Super interessant!
Very clear explanation provided. Thank you Lore. But I have one question. Can we use custom embeddings instead of openai embeddings? Instead of using vectorizers like text2vec-open ai hugging face transformers etc.? If so how to add text into vector store based on these embeddings?
Using the Langchain framework you can also access the Cohore embeddings models. I would advise you to take a look at their documentation: python.langchain.com/docs/modules/data_connection/text_embedding/ 😊
Please how can i get api of weaviate ???
You should be able to retrieve the api of your weaviate vector database via the Weaviate console, if you click on details.
Great video However, I have found some inconsistencies between the code you are shoeing and the file linked on your description I have managed to work it out, thought it is something to note. Looking forwards to see more of your videos !
Thank you for your feedback! Highly appreciated!
Excllent and detailed information good job!
@lorevanoudenhove4946 Running the code at cell in 11:17 part, I get this error: {'error': [{'message': 'update vector: unmarshal response body: json: invalid number literal, trying to unmarshal "\\"rate_limit_exceeded\\"" into Number'}]}
Great video! Just a suggestion: It would help if you could share the ipynb files (e.g. thru collab) from your tutorials. :) Most UA-camrs do it. :)
Thank you so much for the feedback! I'll upload the notebooks soon 🙂
You can find my Google Collab and the data used in the tutorial here: drive.google.com/drive/folders/1n-cz65obR2gI4uyYoHdEa3ts99J965mr?usp=sharing 😊
How do you need to change the code when connecting to a local Weaviate docker image rather than than the cloud sandbox (which is valid only 14 days) ? Secondly, what needs to be changed when I want my own vector database to sit on top of the GPT-3 LLM, as an enhancement rather than a replacement?
Very helpful, thanks. Just curious, but what if I wanted instead of the reply "I don't know" to continue with the usual results from ChatGPT ... if the answer is not in my data, then something more general via the usual gpt model would be shown ?
you'd need to change the langchain qa class method prompt template. Or use a different chain.
Hello Lore thanks for video again. I have vectorstore like that "vectorstore = Weaviate(client, "Techs","description", attributes = ["url","author","title","path"])" and i have search in my documents its find 2 similarty data and ai combine them and answer my question So have can i these datas path below the answer need to give all finded documents path in there In this example its find 2 document and must be give 2 path url from there for example : Answer:.... Soruce 1 : {path 1} Source 2 : {path 2}
Great overview thank you so much for putting this together! very helpful!
Hello Lore thanks for video its very helpful, i have a question to you What is the best method to upload a large JSON dataset with over 12,000 entries, each containing 'title', 'description', and 'author' keys, where the 'description' text in each entry averages around 1000 characters, to Weaviate?
Great tutorial Lore! I enjoyed the pace and easiness. Do you have any tutorials about connecting the bot to a website and put it to work?
Hey Gaston! Happy to hear that you liked the tutorial! I might make a tutorial on that soon, thanks for the suggestion 😁
@@lorevanoudenhove Thanks Lore! I'll keep an eye 😀
What open source LLMs can be used with this design?
Hey Michael! You can use many different LLMs using Langchain, such as the ones available via HuggingFace. On this page you can find an overview of all LLMs integrated in Langchain: python.langchain.com/docs/integrations/llms/ I hope this helps 😁
Super interesting video, very informative, want to learn more creating chatbots!!
Awesome video, Lore!
Thanks! 😁
github?
Hey! Thanks for your comment! I currently don't have a GitHub repository for the code but you can find most of the code in my Medium article: medium.com/p/78ecdbe383c8#c4d3-df9225f3246. I hope this helps! :)
@@lorevanoudenhove hey thanks for your reply
This is an awesome video. I was able to incorporate a bunch of pdfs into weaviate and make queries, but I wanted to modify the chatbot part of the project a bit. It seems like I can ask a question and get a response, but what if I want to further the conversation and ask another question based on the previous response. Basically, I want the chatbot to remember history so I can make a full conversation as opposed to a question/response. Is there an easy way to implement this because I can imagine a scenario where not only does it have to remember the previous context, but it also has to rerun a similarity search in weaviate.
Hey Joe! Happy to hear the video was useful! It is definitely possible to add chat history to your query. Langchain has some great documentation about this: python.langchain.com/docs/use_cases/question_answering/how_to/chat_vector_db. If you would be interested I might create a tutorial about this :)
Thank you for the video, it was very informative. I have a question: I want to upload a very long text to Weaviate. I've installed Weaviate via Docker and wrote the PHP code to post via the API. However, when I try to post a long text, the Weaviate Docker container crashes. Is there a limit for the text size that can be sent to Weaviate, or is there a specific approach I should follow?
Hey Erdem! Glad to hear you liked the video 😁 Regarding your question, I would advise you to split your text into smaller chunks. In the video, I used chunk_size=1000 but you can lower this if you want. I hope this resolves the issue!