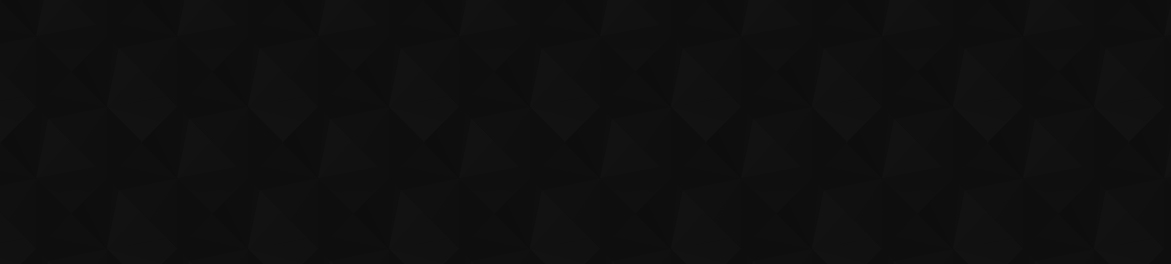
- 195
- 315 499
Chieh Wu
United States
Приєднався 3 лис 2015
Відео
20. K-means, Agglomerative, Spectral Clustering
Переглядів 1514 днів тому
20. K-means, Agglomerative, Spectral Clustering
19. Linear Discriminant Analysis (LDA)
Переглядів 1814 днів тому
19. Linear Discriminant Analysis (LDA)
18. Principal Component Analysis ( PCA )
Переглядів 1714 днів тому
18. Principal Component Analysis ( PCA )
Lecture Example 3: sampling from p(x| U are in love with Prof. Wu and use the lyrics from example 2)
Переглядів 4414 днів тому
example of sampling from a conditional distribution of songs.
Lecture Example 2: sampling from p(x| "in love with Prof. Wu")
Переглядів 1614 днів тому
AI generated song demonstrating sampling from a conditional distribution.
Lecture Example 1: sampling from p(x|"in love with prof wu")
Переглядів 2414 днів тому
Lyrics Yo, Professor Wu is the best and her TA Arielle is not bad too. With Prof. Wu we’re diving deep, it’s a math crusade, Matrix, vectors - the tools of the trade. From calculus slides to the ML game, Simplify equations, let’s ignite the flame. (Verse 1) Big data vibes, I see the gradient flow, Partial derivatives, yeah, I’m in the know. Functions with inputs, multi-dimensional ride, Linear ...
Math Crusaders: DS4400 Theme Song (Lyrics by Phil, Voice by Emily)
Переглядів 8114 днів тому
Math Crusaders: DS4400 Theme Song (Lyrics by Phil, Voice by Emily)
13. Understanding Information theory
Переглядів 2314 днів тому
Professor Chieh Wu's UA-cam lecture and accompanying slides introduce information theory, starting with the concept of information as inversely related to probability. The lecture then progresses to entropy (measuring uncertainty before an event), cross-entropy (measuring the difference between two probability distributions), KL divergence (the excess entropy added by one distribution to anothe...
12. Inversion, Rejection, Importance, KDE Sampling
Переглядів 714 днів тому
12. Inversion, Rejection, Importance, KDE Sampling
11. Maximum Likelihood (MLE) and Kernel Density Estimation (KDE)
Переглядів 2514 днів тому
11. Maximum Likelihood (MLE) and Kernel Density Estimation (KDE)
10. Recognizing the most common Distributions
Переглядів 1014 днів тому
10. Recognizing the most common Distributions
9. LASSO, Ridge Regression, and Elastic Net Regularization
Переглядів 1014 днів тому
9. LASSO, Ridge Regression, and Elastic Net Regularization
Theme Song (Lyrics by Phil and Voice by Emily)
Переглядів 10714 днів тому
Theme Song (Lyrics by Phil and Voice by Emily)
8. The lecture on Expectation Variance
Переглядів 914 днів тому
8. The lecture on Expectation Variance
6. Data Preprocessing and Intro to regression
Переглядів 921 день тому
6. Data Preprocessing and Intro to regression
5. Gradient Descent Vs Closed form explained
Переглядів 4221 день тому
5. Gradient Descent Vs Closed form explained
2. Review of Vector Matrices operations
Переглядів 1421 день тому
2. Review of Vector Matrices operations
please debut
Simple and better
Superb 🎉
How'd I get to this video?
Thanks 👍
Amazing thank you sir
At 15:00 the inverse (-1) is missing in the pseudoinverse
30:50 Should the denominator when computing the Integral operator be 5 instead of 2 (the number of data points)? But does not alter the reasoning anyway...
TOC of the lecture: 01:28 Going back to kernel Theory 03:20 Operators & Functionals 05:36 Linear Operators 07:45 Integral Operator 11:03 Practice your understanding of Integral Operator 12:38 The dimensions of Integral Operators 14:15 Integral Operators Solutions 15:17 Let's quickly review the concept of Span 16:40 Identify the Minimum basis vectors 18:30 Solution to Minimum basis vector 19:20 The minimum set of basis vectors 22:04 Solution 23:32 Symmetric matrices can be split into 3 matrices 24:51 Let's apply the same idea to RKHS 27:30 Eigenfunction allows us to use fewer basis functions 29:35 Practice understanding Eigenfunctions 31:32 More on Integral Operators 34:23 Integral Operators and Eigenfunctions 36:14 How to actually get the Eigenfunctions 40:11 Rosasco's conclusion leads to a very amazing result 42:02 Using Eigenfunctions instead of basis vectors 45:03 Let's now construct the kernel matrix 48:30 Understanding Mercer's Theorem 49:27 Is Rosasco's telling the truth?
TOC of the lecture: 00:45 Recap & Warm up 03:26 Answer 04:10 Remembering the Span in Linear Algebra 05:54 Let's extend the idea of Span to function Space 07:43 Answer for Span in RKHS 08:16 We previously learned ... 10:27 The Moore-Aronszajn Theorem 15:05 The Moore-Aronszajn Theorem in real life 19:43 The Implications of the Moore-Aronszajn Theorem 21:06 The Representer Theorem 21:51 Let's now apply Kernels to Regression 25:07 Where is the advantage? 28:48 Example using Gaussian Kernel 32:09 Evaluating after obtaining α 33:27 Using α to get f(x) 36:09 Answer these questions to consolidate what we learned 37:46 Kernelizing Linear Algorithms 40:12 Python code 40:23 But program your own Kernel Regression & "Thinking through is the key to learning"
TOC of the lecture: 00:48 A function is a combination of functions 03:00 Naming the components of a function 05:58 The basis tells you the space of possible functions, the coeffs tell you the exact function 09:33 Changes how we understand functions 14:16 Functions as points 16:48 The perspective shift 19:49 The Hypothesis perspective 22:02 The wrong hypothesis 25:10 Picking the correct hypothesis 26:20 What is f(x)? And the reproducing property 29:43 The visualization of the Reproducing Property 32:25 Another Visualization of mapping the data into RKHS 33:08 Consolidate these concepts 33:28 Understanding spaces 33:34 A set is a collection of distinct elements 34:00 A space is just a set with some defined perspectives 35:54 Here is a list of major spaces 37:03 Reproducing Kernel Hilbert Space (RKHS) 38:54 Let's define a Kernel 39:52 Let's define a Reproducing Kernel 42:12 What is a Reproducing Kernel? 44:52 How do we use Reproducing Kernels? 49:13 The kernel Matrix 51:03 Try to answer these questions to consolidate the ideas 51:15 Practice using the knowledge This lecture is a gem thanks! The Gretton's lecture referenced in this video: www.gatsby.ucl.ac.uk/~gretton/coursefiles/rkhscourse.html
Great video. Solve lots of puzzles.
This is a very underrated video. Thanks, professor! I hope you keep posting and growing your channel.
Ek number..
34:26 Integral operators and eigenfunctions
What a lovely way of explaination
What the professors don't tell you is that in real life there isn't a formula that can be differentiated and that the 'terrain" isn't like a bowl with an easy path to the minimum.
Thanks again
Thanks!
`Thank you for making these videos! My major is electrical engineering, so having these concepts explained via calculus and statistics makes learning machine learning more approachable. It turns a scary black box into small problems that can be solved.
really intuitive explanations!
Absolute wonder of a video!!!❤❤❤
Thank you very much.
Thanks a lot, Sir! I had been trying to understand the concept since 2 days, and this video gave a very easy and straightforward explanation. Thank You!
This is one of the great and refreshing video for me. I have been looking for some classy materials for kernals and Random Fourier Topics in past few days. Finally, landed at - spot on explaination - this is what I have been looking for. Thank you.🙇
Super Explanation...
Thanks for your incredible explanation in the simplest way!
Is ppt available ?
please public it
university asked me to take it down
i will republish it next semester
hii sir why have hidden the videos
Thank you very much
very good explanation loved it.. can you make video on PCA in machine learning
Watch this lecture series. ua-cam.com/play/PLdk2fd27CQzQo1DEthJ5MDBHoC66_1aDN.html
Brilliant!
Thanks. May I know where can I download the lectures or suggest a book
Thanks for having everything here.
Very good explained !
thank you for this! very useful
Just by looking at it, I know the minimum is at 1,1 so why do you use gradient descent to find it?
Just learning kernels thru ridge regression super helpful thanks !
Timestamps: 15:24
Very nicely explained.
Hi @chiehwu, your teaching style has really helped me grasp the intricacies of derivatives and understand their role in various algorithms. Thank you for sharing these fantastic lecture videos that connect concepts to real-life problems. I've been taking notes by pausing the videos, but it interrupts my flow. Could you please share the slides or notes on platforms like GitHub or Drive for easy download and future reference? Thanks a lot!
That is really a smart explanation
Super informative
Very nice explanation indeed. Yet I still have a question. At 21:02 you say that sum(q(z))=1 and can be excluded from the 2second member of the distribution. But why not on the first???
"Promo SM"
Very nicely explained. Thank you.
Please post links to slides and other materials.
no sound at all?
magnificent
thank you so so much :)