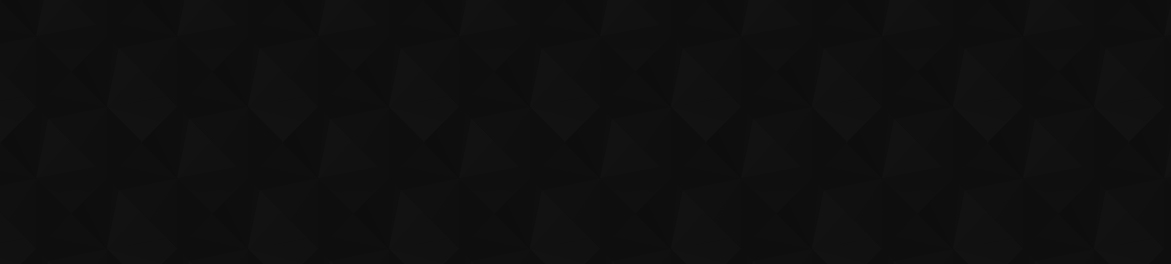
- 22
- 97 911
Dirty Quant
Australia
Приєднався 8 вер 2020
I want to share my knowledge of statistics, coding and all things quant with the world, without confusing you any more than you already are.
A no BS approach, plenty of dad jokes and sweet hip hop beats to match
A no BS approach, plenty of dad jokes and sweet hip hop beats to match
Value at Risk (VaR) Explained in 5 minutes - NO MUSIC
Explaining Value at Risk isn't easy.
Here is an alternative approach using men's clothing and a sense of humor
Here is an alternative approach using men's clothing and a sense of humor
Переглядів: 828
Відео
Value at Risk (VaR) Explained in 5 minutes
Переглядів 3,6 тис.2 роки тому
Explaining Value at Risk isn't easy. Here is an alternative approach using men's clothing and a sense of humor
Testing for Normality - The Jarque Bera Test - NO MUSIC
Переглядів 5042 роки тому
This video has no music so you can watch it at a faster rate Easy test to check if our data is even close to being Normally Distributed Version with music: ua-cam.com/video/IZDpmV-i-VY/v-deo.html Github notebook: github.com/tinoproductions/DirtyQuant/blob/master/JB Test for Normality.ipynb
Testing for Normality - The Jarque Bera Test
Переглядів 6 тис.2 роки тому
Easy test to check if our data is even close to being Normally Distributed Github notebook: github.com/tinoproductions/DirtyQuant/blob/master/JB Test for Normality.ipynb
PDF? CDF? WTF?! Distribution Transformations and how to use them - NO MUSIC
Переглядів 7672 роки тому
This video has no music so you can watch it at a faster rate Let's go through Probability Density Functions, Cumulative Distribution Functions and Inverse Cumulative Distribution Functions and how to use them. Link to PDF: dirtyquant.com/uploads/short-url/tDqEiY9sDncv578kIbd58B35VZc.pdf Github notebook: github.com/tinoproductions/DirtyQuant/blob/master/CDF PDF WTF Mastering Distribution Transfo...
PDF? CDF? WTF?! Distribution Transformations and how to use them
Переглядів 9982 роки тому
Let's go through Probability Density Functions, Cumulative Distribution Functions and Inverse Cumulative Distribution Functions and how to use them. Link to PDF: dirtyquant.com/uploads/short-url/tDqEiY9sDncv578kIbd58B35VZc.pdf Github notebook: github.com/tinoproductions/DirtyQuant/blob/master/CDF PDF WTF Mastering Distribution Transformations.ipynb
2 Minutes of Bad Maths Jokes
Переглядів 8063 роки тому
2 minutes of childish, juvenile and silly maths jokes.
Impostor Syndrome for Quants - No Music
Переглядів 4323 роки тому
How do we overcome impostor syndrome in a highly technical field like quant finance? These are my thoughts on the topic and how best to tackle it This version has no music if you like to speed up your videos Join the discussion: dirtyquant.com
Impostor Syndrome for Quants
Переглядів 8413 роки тому
How do we overcome impostor syndrome in a highly technical field like quant finance? These are my thoughts on the topic and how best to tackle it. Join the discussion: dirtyquant.com
Exploring Seeing Theory - The Best Website to Visually Learn Statistics (No Music)
Переглядів 7373 роки тому
The most beautiful website to visually understand concepts in statistics seeing-theory.brown.edu/ This video has no music so you can watch it at a faster rate Join the discussion: dirtyquant.com
Exploring Seeing Theory - The Best Website to Visually Learn Statistics (with Music)
Переглядів 1,7 тис.3 роки тому
The most beautiful website to visually understand concepts in statistics seeing-theory.brown.edu/ Join the discussion: dirtyquant.com
Skewed Student-t Distribution (Hansen 1994) using Python
Переглядів 2,2 тис.3 роки тому
A no-formulas, graphical introduction to the Skewed Student-t Distribution using the Hansen (1994) specification. We look at the CDF, inverse CDF and PDF. Join the discussion: dirtyquant.com Github link to the notebook: github.com/tinoproductions/DirtyQuant/blob/master/Skewed Student-t Hansen (1994).ipynb
Top 5 Reasons You Should Be Writing Your Own Python Packages
Переглядів 8293 роки тому
Why I think writing your own code rather than relying on Python packages is the smart way forward. Join the discussion: dirtyquant.com
Introduction to DCC - Dynamic Conditional Correlation Models
Переглядів 11 тис.3 роки тому
A no-formulas, graphical introduction to Dynamic Conditional Correlation (DCC) models and why they are useful, all using simple Python libraries. Join the discussion: dirtyquant.com Github link to the notebook: github.com/tinoproductions/DirtyQuant/blob/master/DCC Model.ipynb EDM Detection Mode by Kevin MacLeod is licensed under a Creative Commons Attribution 4.0 licence. creativecommons.org/l...
A Simple Introduction to Copulas
Переглядів 33 тис.3 роки тому
A no-formulas, graphical introduction to Copulas and why they are useful, all using simple Python libraries. Join the discussion: dirtyquant.com Github link to the notebook: github.com/tinoproductions/DirtyQuant/blob/master/Introduction to Copulas.ipynb
Build a GARCH Simulator - Next Level Backtesting
Переглядів 4,6 тис.3 роки тому
Build a GARCH Simulator - Next Level Backtesting
Cholesky Decomposition: Take your Backtesting to the Next Level
Переглядів 8 тис.3 роки тому
Cholesky Decomposition: Take your Backtesting to the Next Level
The Metropolis-Hastings Algorithm (MCMC in Python)
Переглядів 11 тис.3 роки тому
The Metropolis-Hastings Algorithm (MCMC in Python)
Genesis of GARCH - Why you have been measuring volatility wrong all your life
Переглядів 6 тис.3 роки тому
Genesis of GARCH - Why you have been measuring volatility wrong all your life
How to Speed Up Your Regressions in Python
Переглядів 1,2 тис.3 роки тому
How to Speed Up Your Regressions in Python
useless
Hi one question, around 5:10, why you divide all the random data generated by 100? You didn't mention in the video. But can you please advise what's the purpose? thanks!
Correct me if I'm wrong, but at 11:30, it appears you aren't using the Hastings ratio like you described in the preamble, just the Metropolis one.
Nevermind, just realized what transition density you were using!
great thanks man
Great video. I loved "loading word" and "let's play a game" - hilarious! Thanks 🤣👌
so does this mean if our algorithm passes the backtest using this simulated paths it will be profitable in the future? or what other assumption do we need more?
The channel name is quite misleading, it should be CLEAN Quant!!! Thank you so much for your works!
Thanks for the video! Just wanted to ask if in your opinion does make sense to use this to model implied volatilities, or might be better to use a multivariate OU process (with log(x)). Thanks!
thanks for the amazing video. do you have an idea how to calculate the composite likehood with pairwise method over all constituents, meaning over all neighboring paris of assets to estimate the two dcc parameters alpha and beta? i like to understand how to calculate on a big data set like you have done with the complete s&P 500. Pakel et al. (2020) - Fitting Vast Dimensional Time-Varying Covariance Models suggets to do that with pairwise likelihood. do have have an idea how to implement it in your code?
Hey, I was wondering about a source for the CDF and PPF? The PDF is defined in Hansen's paper but I don't see a derivation of the CDF and PPF. Is there a follow up paper in the literature that derives these?
Hello. Last plot can't be executed. Something went wrong.
this video is really amazing , explanation was simple and on point cool editing too.
Is there any website similar to this , for basic mathematics for Data Science? Thanks PP
Straight Outta Pandas 🤣
From what I am reading, I am getting an idea of what copulas do, but I am trying to figure how to apply copulas to my problems is the tough part. Plus, I am thinking of publshing my work in a journal that is more measurement science oriented, so I really want to get it right. I am good at math until it comes to proofs and I notice statistics tend use more a mathematician type presentation that say engineering and physics math.
Excuse me, but what is the presenter referring to by marginals?
Hi mate, marginals is just a fancy word for the distribution of the 2 separate datasets. So time can have a certain distribution and money spent a totally different one. These 2 datasets are the marginals.
Amazing video! Thank you so much
You are super welcome
very usefull thanx aloot
Welcome mate!
nice explanation, but distracting music and b-roll of keyboard.
True annoying background noise. I closed the video because of this
9:26 brain afk...
okay this not semantics in linguistics, cool video though
Sorry but is explainied so so bad....really. It was easier to understand the Literatur then this video...
Lol. Sorry to disappoint.
@@dirtyquant disappointment just like my son
Hi! First of all many thanks for your video. You clarified a lot of stuff that is a necessity for my thesis. I do run into an issue. I want to use the student's skew t distribution for my DCC-GARCH model. I have adjusted dist to 'skewt'. In the function garch_t_to_u. Also, i have added eta and lambda as parameters. Then, udata = t.cdf(std_rest, lambda). However, now opt.out.success provides a False and I do not have any estimations. Could you please let me know how I can adjust the code to use the skewed T distribution? If I make mistakes in my adjustments as explained above please let me know. Many thanks in advance!
Hi mate. Can you post this on dirtyquant.com and I will look at it later
Hey.. very helpful video.. can you help me the code of DCC-MIDAS-X Model which is somewhat similar to this only.. i am unable to incorporate this model.. please help
Thanks very helpful video. Could you please suggest how can exogenous variable X be incorporated into DCC garch model to see the impact of X on correlation of different assets
Glad you liked the video. Does the exogenous variable have a correlation with GARCH characteristics? You can’t just throw anything in there as it won’t converge. Just add it as another asset and see what it looks like. No problem in doing that. Remember we are looking at correlation of VOLATILITY, not returns.
@@dirtyquant Thanks for replying X has garch characteristics i want to check whether variable X impact the dynamic conditional correlation of two assets..like if it has positive or negative effect
It won’t have any effect on the other 2 variables. But you can look at the time series of the DCC and I find that useful. Let me know how you get on. You can post your findings on dirtyquant.com
Hi, it's me again lol. Do you have any related videos that's calculating DCC forecast model? Thank you!
Hi mate. DCC automatically does 1 step ahead forecast
@@dirtyquant Hi, I'm quite curious how to modify the code if I want 30 days ahead forecast. Do I just change the looping through Rt?
It was really a little complicated to me to calculate DCC and CCC (Constant Conditional Correlation) in Excel. I had to use a LGARCHMLE (leveraged model) with American Indexes: Nasdaq, S&P500 and Dow Jones. However, it's gonna be very difficult to calculate it in Python. I heard that R programming is very easy to estimate it. I didn't know that DCC can be calculated in Python too. What about CCC? Your code looks complicated. Can you share it? Regards.
Link in the description to my GitHub with all the code
Hi , it's a really helpful video. Really appreciated :)) I have tried to walked through your code. Just have a question. If I wanna get the DCC correlation of the stocks do I just extract the Rt value? Thanks in advance.😁
Hi, yes, but that will give you the correlation of volatility, not of the returns, as it’s way more stable.
@@dirtyquant Hi Dirtyquant, Thanks so much for getting beck so quickly! Is there any way that I can modify the code to get the return correlation. I tried input return instead of udata_list but the model shows not success.
The model is (likely) not going to converge if you use returns as they as far too unstable. You need to convert the returns to a uniform distribution using the appropriate CDF for that distribution. Again, I don’t think it will work as the autocorrelation of returns decays far too quickly so it will be incredibly noisy. Please try it and report back. :-)
Thank you! I’ll try it out 🥹
amazing thanks!
Welcome!
I discovered your channel today and I sincerely hope that you never stop making videos :)
Thank you. That is very flattering. I hope to make many more
When running the worksheet the beta co-efficient produced when fitting GARCH to the simulated time series with the shock value is no longer close to 0 as in the video, instead it's 0.89 as you suggested it should be (and the spike is no longe present in the plot of conditional volatility of simulated returns). Not sure why....
Great video, thanks for sharing!
Glad you like it mate!
Hey dirtyquant. Did you work first before pursuing your PhD? In general, do you recommend it compared to going into a phd program straight out of undergrad?
Ha! That’s a million dollar question. I had been working for 7 years before I did my PhD. Certainly provided a different perspective. There is no right or wrong way. The advantage of going straight after undergrad is that you have a PhD earlier, disadvantage is that you STILL have no experience and no business network once you are done. There is also a certain maturity that would benefit something as long and frustrating as a PhD. I would say, depends on your personal situation, but I would prefer going after some work experience (if you can detach yourself from the salary teat)
@@dirtyquant thanks for your perspective. I asked this as an undergrad, who is sitting here, one week from graduation from my bachelors degree, with two offers, one for a funded MS in statistics and a fully funded PhD in statistics. My last two years of school I really enjoyed classes and learning stats, and thought I would want to do a phd immediately. However, after I started reading more and more about the applications of statistics to finance, and especially the roles in quantitative research and analysis that are out there in hedge funds, investment banks and other financial institutions, it’s like that passion and interest for doing it has been erased for now. I have opted for going to my MS program and to try and land such a role to work first, and then possibly go back, because I have not found the drive to really go straight out of undergrad. It sucks cause I thought it was really passionate about this whole academic journey and now going back on what I originally planned on feels like a big deal. I guess what your saying is it must vary from person to person. For me I feel like after just getting a break from school after my MS and working will just clear my mind a bit before a phd. I also just felt very uncertain about going into a phd immediately, due to my lack of actual industry experience. However, over the last week, this has been a constant back and forth, and still I just don’t even know if me denying the phd offer is me making a huge mistake. I just figured I should listen to my gut and if I feel like I need a break I need a break. What do you think about this?
Basically the same is happening in your example here. The returns you are pulling have a mean value of 0.0178. The model has a mu of 0.0669, and some random price paths I just generated were also far above the return of 0.01.. ?
Hey Julian, I am out travelling (at PyCon actually) so can’t look into this too much, but you could just re-mean it to whatever you like. You subtract the average mean, and punch in the value that you like. Easy dirty quant solution!
@@dirtyquant Appreciate the answer! I could do this, but I want to use the simulated price paths in an academic paper so I need to justify decisions like this.. you see what I mean? Enjoy PyCon!!!
@@juliangoogle6022 now you got me thinking. Let me try and run some stuff now and see what is up. When you get an ear worm like this you can’t let it slide. Will get back to you
Ok, so I ran the simulator and it actually looks fine. There is a distribution for the mean as well. Sometimes it will be more, sometimes it will be less. It won't be a constant for each of those runs. Run the notebook and then look at sim_df.mean(axis=0).mean() It gave me something that's within the confidence interval of the output of garch_output.summary at the beginning of the notebook It will hold for academic papers 🙂
@@dirtyquant Yes so the mean of the sim_df.mean is consistent with the mu value of the GARCH model, but I suspect the mu value (and with that also the confidence intervals in the garch_output.summary) to be too optimistic, no? If I take the mean of the returns you pull from yahoo I get 0.021428 (with the command returns.mean()). And the mu in the GARCH model is 0.0524 --> more than double? So as a result all the price paths simulated with the GARCH model will be much more bullish than the real data we pulled right? For me, this doesn't make sense logically: I throw data with mean 0.02 into a model and the model returns the mean 0.05. This also makes the forecasts unsuitable for backtesting because they are way too bullish
Hi your videos are very helpful. thanks for making this knowledge available to everyone this is top notch.
Thank you David. Glad they help
Hey mate, one more question: How come that the sim returns all seem to take off pretty quickly after a certain number of days? Context: I use 36 years of Eurostoxx600 (index with 600 largest European companies) data to get the GARCH parameters. In real life, I obtain a CAGR of 4.35%, the mean CAGR of many many simulated returns based on these parameters is 8.3%. So it seems like the GARCH model is too bullish over a longer period of time...
If I compare it to the price paths I simulated with the Geometric Brownian motion, they pretty much stick to the drift and the random walk component, also in the long run ... How come the GARCH model loses its stated "constant mean" after a while?? And is there something I can do to avoid it? My idea is to use the log returns instead of the normal daily returns to "normalize" and hopefully stabilize the model a bit. EDIT: Using log returns didn't change a lot, I obtain very similiar results
Great video!
Thanks!
@@dirtyquant Hey you are even answering to comments, amazing! :D Quick question: The simulated price paths all have certain characteristics from the "real" returns in common, right? I am backtesting a strategy and would like to see how much those characteristics (I assume it's the mean and the standard deviation) can change until my strategy doesn't work anymore. Does this make sense to you? Where do I actually see what "characteristics" the GARCH simulator pulls from "returns"? How could I insert values here manually to see when my strategy doesn't work anymore? Thanks already!
@@juliangoogle6022 Hey, so you can play around with the Beta (the memory) and the alpha (the shock) which will define how much "Garchness" there is. At the same time, mu (the mean) nu (the degrees of freedom) and lambda (the skewness) will also define what the return series look like. I would start by fixing all the parameters except one, and changing it whilst feeding into your backtester and see when things start breaking down. most of these won't have massive realistic ranges (Beta is always around 0.9) so your search universe won't be huge. Hope that helped.
@@dirtyquant Okay got it, thank you! I think for the analysis, it would be more intuitive to a reader to see how much the volatility or the mean return can change until the strategy breaks instead of the greek parameters, is there a way to translate that in this case?
I also simulated price paths with the Geometric Brownian motion, here it is easy because the whole model consists of the mean and the volatility but I would love to do it with GARCH because this takes the volatiltiy clustering into account
👏👏👏
Thank you!
Such brilliant production quality!
Thank you. I am flattered
brilliant explaination, i spend longgg longg time to understand from other stuff, but this explanation was wow!
Ha! Glad it helped you out. I always struggled too but one day it clicked. Now I want to tell people about it :-)
why computing the garch volatility you multiply that cond vola by 1600? in this part of code: 'garch_vol_df = pd.concat([pd.DataFrame(model_parameters[x].conditional_volatility/100)*1600 for x in model_parameters], axis=1)'
256 days in a year. Square root is 16. Multiplied by 100 to make 0.01 or 1% equal to 1.00 in the code. 16 x 100 = 1600 Thanks for checking!
Thank you. Great video. Learnt heaps!
Glad it helped
Thanks alot. Very impressive. Can you help me in VARMA-CCC-GARCH?
Would love to understand copulas but couldn't watch this video with the music (and even talking DJ) overwhelming it -- how am I supposed to concentrate on what you're saying? I thought it must be an accident but it was apparently intentional. I guess all your videos are like that? Very strange choice -- unwatchable.
Haha. Sorry I can’t please everyone. Merry Xmas :-)
Really enjoy your videos, keep them up! Some ideas for next vids: various models for pricing and their applications (BS, Monte Carlo, Vasicek), numerical models, neural networks? Also, maybe to dig in deeper into these topics, ur doing a great job of introducing those concepts but if you could elaborate on them a little further it would be great
Appreciate the feedback mate! Thank you!
Wow that plot showing the CDF uniform distribution totally clicked for me. I had read about that before but it didn't make any sense until now
Very happy to hear you found some value Luke
Great video !
Thanks mate!
Great videos, would be better if the code was larger. It’s tough to see on mobile
Thanks mate. Yeah, will try and make it super zoom mode next time
List comprehensions?
What is the best way to contact you directly? I am trying to break into the quant industry and find your videos really helpful and was wondering if I could get your advice for graduate school. Let me know either way!
Hey, sorry I missed this message! Go to dirtyquant.com and we can chat there