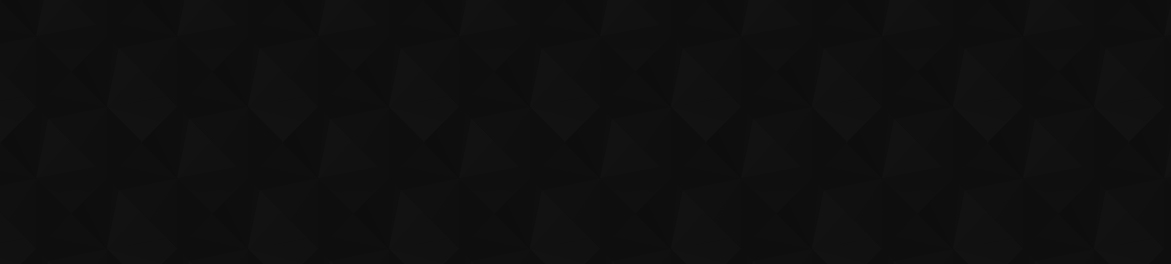
- 135
- 219 496
Data Heroes
United Kingdom
Приєднався 28 вер 2010
Welcome to my channel! Here, I dive into all things data science, AI, and machine learning. I create tutorials, hands-on projects, and deep dives into analytics topics to make these skills accessible for everyone-from beginners to experts. My focus is on practical applications, so whether I’m building neural networks, exploring large language models, or demonstrating data visualization techniques, I keep it actionable and straightforward.
My goal is to help you build real-world skills and solve complex problems using data. Subscribe to stay updated on the latest in data science, essential AI tools, and tips to boost your analytics workflow and career.
For business inquiries, partnerships, or sponsored content opportunities, please reach out to me at diogo@thedatahero.com. Let’s work together to create valuable content for the data science community!
My goal is to help you build real-world skills and solve complex problems using data. Subscribe to stay updated on the latest in data science, essential AI tools, and tips to boost your analytics workflow and career.
For business inquiries, partnerships, or sponsored content opportunities, please reach out to me at diogo@thedatahero.com. Let’s work together to create valuable content for the data science community!
The AMAZING Science Behind Large Language Models!
Free eBook on Prompt Engineering: thedatahero.com/prompt-engineering-ebooks/
Discover how large language models (LLMs) like GPT-3 and GPT-4 are reshaping AI with groundbreaking advancements.
In this video, we break down key concepts like few-shot learning, zero-shot learning, and scaling laws, referencing pivotal papers like "Language Models are Few-Shot Learners" (2020) and "Scaling Laws for Neural Language Models" (2020).
I also explore recent insights on factual accuracy in LLMs from Jerry Wei et al. (2024), highlighting innovations like the SAFE method.
Whether you're curious about AI breakthroughs or ethical concerns, this video offers a concise, expert analysis of where AI is headed.
Discover how large language models (LLMs) like GPT-3 and GPT-4 are reshaping AI with groundbreaking advancements.
In this video, we break down key concepts like few-shot learning, zero-shot learning, and scaling laws, referencing pivotal papers like "Language Models are Few-Shot Learners" (2020) and "Scaling Laws for Neural Language Models" (2020).
I also explore recent insights on factual accuracy in LLMs from Jerry Wei et al. (2024), highlighting innovations like the SAFE method.
Whether you're curious about AI breakthroughs or ethical concerns, this video offers a concise, expert analysis of where AI is headed.
Переглядів: 96
Відео
AI Agents with CrewAI, Explained
Переглядів 9414 днів тому
Learn CrewAI here: www.udemy.com/course/generative-ai-rag/?referralCode=5543F4D3C8910801FBB0 Learn how to set up and automate tasks with CrewAI agents in this tutorial. Discover how to use AI agents effectively to streamline workflows, improve productivity, and get hands-on with CrewAI's templates for automated agency tasks. This video covers: Setting up CrewAI agents Automating tasks with AI E...
Complete AI Agents with OpenAI Swarm Multiagent Framework
Переглядів 47021 день тому
OpenAI Swarm is available in my Udemy course about GenAI, RAG and AI Agents: www.udemy.com/course/generative-ai-rag/?referralCode=5543F4D3C8910801FBB0 Discover how OpenAI Swarm's multi-agent framework revolutionizes AI automation! In this tutorial, we dive into the power of AI agents and their collaborative capabilities within OpenAI Swarm. Learn how these advanced systems work together to opti...
Amazon Chronos Architecture: Gen AI for Time Series
Переглядів 249Місяць тому
Check my FREE ebook on Prompt Engineering: Welcome to a deep dive into the architecture of Amazon Chronos, an innovative tool that applies large language models (LLMs) to transform time series forecasting. In this video, we'll explore key components of Chronos, including tokenization, training, and inference processes, all of which contribute to its robust forecasting capabilities. Discover how...
WANT to Master N-BEATS Model Architecture? WATCH THIS NOW
Переглядів 174Місяць тому
Here is my collection of free eBooks: thedatahero.com/ebooks/ Learn about the N-BEATS model architecture for time series analysis and forecasting in this video. Understand how this model works in data science and how to implement it in Python for accurate predictions.
Temporal Fusion Transformers, EXPLAINED. Advanced Time Series Forecasting
Переглядів 1,5 тис.Місяць тому
Check my colleection of free eBooks here: thedatahero.com/ebooks/ In this video, we'll dive into Temporal Fusion Transformer (TFT) for time series forecasting. Learn how to implement the TFT model and explore its applications in time series analysis. Whether you're new to deep learning or looking to enhance your skills in time series forecasting, this tutorial will guide you through the steps t...
What is RAG? RAG architecture, explained
Переглядів 1912 місяці тому
Get my free eBook on Prompt Engineering here: thedatahero.com/prompt-engineering-ebooks/ Curious about RAG architecture? In this video, we break down what RAG is and how it works. Learn all about retrieval augmented generation in simple terms!
Python for Data Analysis Promo Video
Переглядів 352 місяці тому
Python for Data Analysis Video: www.udemy.com/course/regression-analysis/?referralCode=B9069FC3F04C76F54B37 Are you ready to transform how you handle data? Dive into Python with me, and emerge as a force in data analysis and business intelligence. This course isn't just about learning Python; it's about mastering the skills that will make you indispensable in today's data-driven world. Why Lear...
RAG and Generative AI with Python Promo Video
Переглядів 782 місяці тому
See the course here: www.udemy.com/course/generative-ai-rag/?couponCode=LAUNCH Are you struggling to build RAGs? As the amount of digital content grows exponentially, it becomes increasingly challenging to create AI models that can efficiently sift through vast data to provide accurate and meaningful responses. Traditional search engines and basic AI models often fall short in delivering the co...
Attention is all you need. Transformer architecture explained
Переглядів 2462 місяці тому
Attention is all you need. Transformer architecture explained
Tokenization for Generative AI and RAG, Explained
Переглядів 1662 місяці тому
Tokenization for Generative AI and RAG, Explained
Time Series Analysis and Forecasting with Python Promo Video
Переглядів 953 місяці тому
Time Series Analysis and Forecasting with Python Promo Video
Python for Data Analysis Free Crash Course (9 hours!)
Переглядів 3253 місяці тому
Python for Data Analysis Free Crash Course (9 hours!)
Forecasting Models & Time Series Analysis for Business in R Promo Video
Переглядів 614 місяці тому
Forecasting Models & Time Series Analysis for Business in R Promo Video
3 Free GenAI Models from Google and Meta: Complete Step-by-Step
Переглядів 1384 місяці тому
3 Free GenAI Models from Google and Meta: Complete Step-by-Step
Transform Your Business with GenAI: Top 5 Strategies
Переглядів 554 місяці тому
Transform Your Business with GenAI: Top 5 Strategies
Do this to become a Data Analyst for free
Переглядів 1265 місяців тому
Do this to become a Data Analyst for free
Mastering ChatGPT Prompt Engineering: The Ultimate Free Ebook🚀
Переглядів 1915 місяців тому
Mastering ChatGPT Prompt Engineering: The Ultimate Free Ebook🚀
Data Analysis Gone Wrong: Don't Make These 3 Common Mistakes!
Переглядів 1756 місяців тому
Data Analysis Gone Wrong: Don't Make These 3 Common Mistakes!
Data Science in 2024: Insights & Obstacles
Переглядів 1037 місяців тому
Data Science in 2024: Insights & Obstacles
Top 5 Pandas Tricks You Don't Know About
Переглядів 2127 місяців тому
Top 5 Pandas Tricks You Don't Know About
Is Data Science Dead in the Age of AI?
Переглядів 1977 місяців тому
Is Data Science Dead in the Age of AI?
Statistics with Excel Crash Course (4 hours!)
Переглядів 7397 місяців тому
Statistics with Excel Crash Course (4 hours!)
Improve Critical Thinking with Decision-Making Course - Level Up Your Skills
Переглядів 55410 місяців тому
Improve Critical Thinking with Decision-Making Course - Level Up Your Skills
ChatGPT API for Education: Python Chatbots with OpenAI
Переглядів 33511 місяців тому
ChatGPT API for Education: Python Chatbots with OpenAI
How To Create Custom GPTS With ChatGPT (For Beginners)
Переглядів 15 тис.Рік тому
How To Create Custom GPTS With ChatGPT (For Beginners)
Gordon Ramsay Meets AI: Fusion Cooking with ChatGPT!
Переглядів 180Рік тому
Gordon Ramsay Meets AI: Fusion Cooking with ChatGPT!
Python & OpenAI: Advanced ChatGPT Bot Development Tutorial
Переглядів 323Рік тому
Python & OpenAI: Advanced ChatGPT Bot Development Tutorial
How do you handle dates that are the same? So lets say I have multiple 1/1/2024 rows, how should I modify the data?
If you want to master OpenAI Swarm, Ai Agents and more, enroll my course here (link contains a special offer): www.udemy.com/course/generative-ai-rag/?referralCode=5543F4D3C8910801FBB0
If you want to master CrewAI, Ai Agents and more, enroll my course here (link contains a special offer): www.udemy.com/course/generative-ai-rag/?referralCode=5543F4D3C8910801FBB0
wow great! Can TFT Model use for rainfall induced landslide prediction? with variables like rainfall , soil moisture , slope. Can it be used even if there's limited dataset? or it requires large dataset? thank youu
Deep learning models excel with large datasets. You watched the video, right? Imagine applying that complexity to a small dataset. It would like overfit
so TFT is not appropriate for landslide prediction?
@@MrToua-i9i TFT is not appropriate for small data sets. It is definitely appropriate for landslide prediction
What a fucking fantastic video
Thanks!
Good intro ty
Welcome!
Thank you for clear explenation about it
Welcome 😁
...
This was the first video that popped up when i searched how is cholera spread. Thanks!! Love your voice too.
Thanks!
Good advice for someone who is trying to break into this job market like me. Thanks for sharing.
Glad to hear it :)
Here from the Coursera course. 😁
Welcome!
Thank you for your good Explaination. I'm writing at the moment my Bachelor Thesis about this and the Video really helped me.
Glad to hear it :)
GAAARRN,🧘♂ i love this video. i was searching for TFT and for this kind of explanation, love it
Glad to hear it!
I've just gone down to the comments section to say the same thing. Absolutely amazing, thank you @DataHeroes
@@tomasmichek5842 thanks!
Hi, will this be covered in your course? Thanks
It is covered already in the course :)
Here is my collection of free eBooks: thedatahero.com/ebooks/
Thanks a lot.
My pleasure :)
explicação top mano, obrigado! Alguma sugestão de onde estudar mais a fundo, com demonstração matemática etc? Só pela pura curiosidade mesmo haha
Tens o research paper mas de resto não conheço
Scammer
Thanks..keep going(:
Thanks!
This is sad to watch. I'm almost considering buying this shitty and overpriced microsoft poduct so the Devs don't realize they have not accomplished anything in their lives
It's Open Source :)
What does RAG stand for?
Retrieval Augmented Generation
@@DataHeroeshey uh.....Im kind of overwhelmed a little cause I don't know about computers that much but uh...can you tell me the purpose or use of RAG and what does it do?
I wish I could have a talk with you, I wanna know how to get this and install it in my pc.
You use python programming to set it up :)
Check my free ebook on prompt engineering here: thedatahero.com/prompt-engineering-ebooks/
can it predict stock prices?
Stock prices are unpredictable :(
Best Python for data science tutorial ever. I'm using it to ramp back up on Python after a 5 year hiatus. Can't wait to see what the reward gift is! 😀
Thanks!
Sir, I have determined soil Exchangeable acidity along with 7 other soil properties for 850 soil samples. I want to check like you did in the video by keeping Exchangeable acidity as Dependent and 7 other soils properties using Random Forest. Could you kindly share your invaluable knowledge.
Can you replicate the code? That should work :)
Get the Resources to tag along the course here: data-heroes-2.ck.page/python-crash-course
Your video are great just one thing you need creativity to thumbnail,title and opening section so people get hook ,KEEP GOING
Thanks!
Diogo Resende, you're a true data hero. 😍
Thanks!
Get the materials for this video here: data-heroes-2.ck.page/genai_conversation
Hello! What about the "effect" option? I found that in my code it is necessary to specify [effect = "twoways"] when accounting both for state and year fixed effects, otherwise it just accounts for the first specified (in this example "state").
Nice concepts; thank you for making this video. 👍
Thanks!
Get the free ebook here: data-heroes-2.ck.page/prompt_engineering
To get the script and video: data-heroes-2.ck.page/openai_video
I LOVE THIS!!!!!!!!
Thanks!
Hi! I need help for my codes, i had this code: # Determine the number of steps to forecast steps = len(filtered_x_future) # Handle cases where no exogenous variables are used if fit_sarima.model.k_exog == 0: return fit_sarima.get_forecast(steps=steps) # Construct the exog array for forecasting exog_shape = (fit_sarima.model.nobs + steps, fit_sarima.model.k_exog) exog = np.zeros(exog_shape) exog[:fit_sarima.model.nobs, :] = fit_sarima.model.exog # Ensure the filtered_x_future has the correct shape if filtered_x_future.shape[1] != fit_sarima.model.k_exog: # Adjust the filtered_x_future array to match the required shape if filtered_x_future.shape[1] < fit_sarima.model.k_exog: # Pad with zeros if there are fewer columns pad_width = fit_sarima.model.k_exog - filtered_x_future.shape[1] filtered_x_future = np.pad(filtered_x_future, ((0, 0), (0, pad_width)), 'constant') else: # Truncate if there are more columns filtered_x_future = filtered_x_future[:, :fit_sarima.model.k_exog] exog[fit_sarima.model.nobs:, :] = filtered_x_future return fit_sarima.get_forecast(steps=steps, exog=exog) But it kept showing errors even tho i asked chatgpt: cannot reshape array of size 320 into shape (16,10), Provided exogenous values are not of the appropriate shape. Required (16, 10), got (32, 10). Do you perhaps know how can i resolve this?
Get the free ebook here: data-heroes-2.ck.page/prompt_engineering
Download the Propensity Score Matching Python script: data-heroes-2.ck.page/psm_python
promo sm 💪
If you want to make this R script yours, get it here: data-heroes-2.ck.page/xgboost_r
If date column is not present in dataset?
You can create one with Pandas
@@DataHeroes how
Download here the Cluster Analysis script and the data for this project: data-heroes-2.ck.page/cluster_analysis
Hello author , i tried your experiment on my dataset, features are not printed in shap?
What was the error? Did you try with the example code?
@@DataHeroes output is not getting printing properly since features are overlapping
Get here the Apriori script with extra content: data-heroes-2.ck.page/apriori
very amazing tutorial! very informative and funny 😀
Thanks!
ultimate causal analysis ! loved the tutorial
Thanks!
Download the R script from this video here: data-heroes-2.ck.page/fixed_effects_regression
FREE EBOOK on Conjoint Analysis with Python: data-heroes-2.ck.page/conjoint_analysis_ebook
Great video. Do you have any video where you discuss hyper parameter tuning for xgboost in R?
I do have an online course on it :) you can see it in my discount portal
HALLELUJAH
Here is the link to get the script: data-heroes-2.ck.page/sarimax