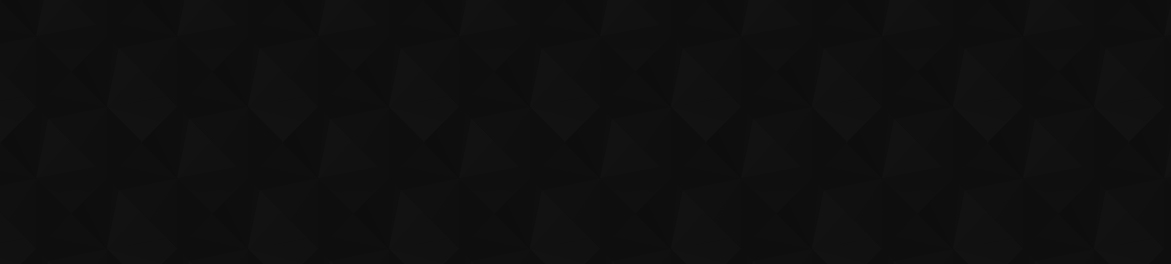
- 78
- 330 909
Hudson & Thames
United Kingdom
Приєднався 19 лип 2020
Our mission is to promote the scientific method within investment management by codifying frameworks, algorithms, and best practices to build the world’s central repository of ready-to-use implementations.
Conditional Portfolio Optimization
Join the Hudson and Thames Reading Group: hudsonthames.org/reading-group/
In this session we are discussing a paper about the latest advancements in portfolio optimization. "Conditional Portfolio Optimization using Machine Learning," presents a new method that adapts capital allocations to market regimes. This CPO method leverages machine learning and big data to provide an optimal solution to commercial problems such as portfolio optimization that adapts to the environment. In multiple use cases, the authors have demonstrated that it outperforms conventional methods.
In this session we are discussing a paper about the latest advancements in portfolio optimization. "Conditional Portfolio Optimization using Machine Learning," presents a new method that adapts capital allocations to market regimes. This CPO method leverages machine learning and big data to provide an optimal solution to commercial problems such as portfolio optimization that adapts to the environment. In multiple use cases, the authors have demonstrated that it outperforms conventional methods.
Переглядів: 1 135
Відео
Deep Reinforcement Learning for Trading
Переглядів 3,4 тис.8 місяців тому
Join the Hudson and Thames Reading Group: hudsonthames.org/reading-group/ This Friday for our weekly reading group we explored the paper: "Deep Reinforcement Learning for Trading”. The authors adopt Deep Reinforcement Learning(RL) algorithms for designing trading strategies in continuous futures contracts. These algorithms outperform classical time series momentum strategies, delivering positiv...
Scientific Discovery in Quantitative Finance
Переглядів 1,5 тис.8 місяців тому
This lecture we review the first 4 chapters of Marcos Lopez de Prado's latest paper from ADIA Labs, which lays out the foundation of the scientific discovery process in finance and how Causal Inference is the next step. Based on: Causal Factor Investing: Can Factor Investing Become Scientific?
FinGPT: Open-Source Financial Large Language Models
Переглядів 20 тис.Рік тому
Join the Hudson and Thames Reading Group: hudsonthames.org/reading-group/ We explored the paper and open-source project, “FinGPT: Open-Source Financial Large Language Models”. It aims to democratize access to high-quality financial data and stimulate innovation in applications like robo-advising and algorithmic trading. Collaboration within the AI4Finance community is encouraged, and the associ...
Git, Branching, and Pull Requests
Переглядів 763Рік тому
Welcome to our video on Git fundamentals presented by Michael Struwig, the CEO of Hudson & Thames. If you have some basic familiarity with Git, and would like to learn how to think about source control, branching and pull requests, this video is perfect for you.
PortfolioLab Demo Video
Переглядів 1,5 тис.Рік тому
PortfolioLab: Portfolio optimisation and allocation library A modern guide to portfolio optimisation: hudsonthames.org/modern-guide-to-portfolio-optimization/
ArbitrageLab for Pairs Trading, Demo Video
Переглядів 2,8 тис.Рік тому
An overview of ArbitrageLab, Hudson and Thames' flagship pairs trading python library. Statistical arbitrage pairs trading strategies: Review and outlook: www.econstor.eu/bitstream/10419/116783/1/833997289.pdf The definitive guide to pairs trading: hudsonthames.org/definitive-guide-to-pairs-trading/
MlFinLab Demo Video
Переглядів 1,1 тис.Рік тому
Learn more: hudsonthames.org/mlfinlab/ MlFinLab is a collection of production-ready algorithms (from the best journals and graduate-level textbooks), packed into a python library that enables portfolio managers and traders who want to leverage the power of machine learning by providing reproducible, interpretable, and easy to use tools.
Model Interpretability using the Model Fingerprint
Переглядів 1,4 тис.2 роки тому
Join the reading group: hudsonthames.org/reading-group/ The first lecture from the Hudson and Thames Reading Group, focuses on a model interpretability algorithm known as the model fingerprint, first published in the Journal of Financial Data Science. The algorithm decomposes model predictions into linear, nonlinear, and interaction components and studies a model’s predictive efficacy using the...
Cointegration Tear Sheet Example
Переглядів 1,5 тис.2 роки тому
Join our reading group! hudsonthames.org/reading-group/ Visualization of a cointegration dashboard.
OU Model Tear Sheet Example
Переглядів 8972 роки тому
Join our reading group! hudsonthames.org/reading-group/ OU Model Dashboard
Feature Importance Workshop
Переглядів 1,9 тис.2 роки тому
Join our reading group! hudsonthames.org/reading-group/ In this workshop, we use feature importance techniques such as: MDI, MDA, Clustered Feature Importance (CFI), The Model Fingerprint, Shapley values, and LIME. We do so using breast cancer data as it is a benchmark dataset that allows the user to easily understand how the models work, and to build an intuition.
Modelling: Label Concurrency and Cross Validation
Переглядів 1,3 тис.2 роки тому
Join our reading group! hudsonthames.org/reading-group/ This lecture explores label concurrency and the pros and cons of various cross-validation techniques in financial machine learning. Namely: K-Fold, Walk Forward, and Purged K-Fold.
Modelling: Sample Weights
Переглядів 1,6 тис.2 роки тому
Join our reading group! hudsonthames.org/reading-group/ MlFinLab supports two methods of applying sample weights. The first is weighting an observation based on its given return as well as average uniqueness. The second is weighting an observation based on a time decay.
Bagging and Boosting in Financial Machine Learning
Переглядів 1,4 тис.2 роки тому
Join our reading group! hudsonthames.org/reading-group/ Learn how ensemble methods such as bagging and boosting are used in financial machine learning.
11 Stylized Facts of Financial Time Series
Переглядів 2,6 тис.2 роки тому
11 Stylized Facts of Financial Time Series
Labelling Techniques in Trading: Filters and Fixed Time
Переглядів 2 тис.2 роки тому
Labelling Techniques in Trading: Filters and Fixed Time
Labelling Techniques in Trading: Triple-Barrier and Meta-Labelling
Переглядів 4,2 тис.2 роки тому
Labelling Techniques in Trading: Triple-Barrier and Meta-Labelling
Trailer: Portfolio Optimization Workshop
Переглядів 4792 роки тому
Trailer: Portfolio Optimization Workshop
Hierarchical Equal Risk Contribution (HERC)
Переглядів 2,4 тис.2 роки тому
Hierarchical Equal Risk Contribution (HERC)
Nested Clustered Optimization (NCO)
Переглядів 1,3 тис.2 роки тому
Nested Clustered Optimization (NCO)
Theory-Implied Correlation Matrix (TIC)
Переглядів 1,1 тис.2 роки тому
Theory-Implied Correlation Matrix (TIC)
Wrapping up MVO and learning about Denoising, Detoning, and Shrinkage methods.
Переглядів 1,1 тис.2 роки тому
Wrapping up MVO and learning about Denoising, Detoning, and Shrinkage methods.
Portfolio Optimization: Mean-Variance Optimization and the Critical Line Algorithm.
Переглядів 3,5 тис.2 роки тому
Portfolio Optimization: Mean-Variance Optimization and the Critical Line Algorithm.
Great content! I have a question about bootstrapping in time series data: Since financial time series are sequential and each data point depends on the previous ones, doesn't bootstrapping break this dependency by randomly sampling points? How do we preserve the temporal structure while still using bootstrapping? Would block bootstrapping or another method be more appropriate?
Horrible microphone quality. Thanks for uploading tho
Can someone tell me why the simulation is necessary here? Since we can get real data from yfinance?
23:40 Shouldnt it be a buy trade?
The best way to use simulation data as to create your own data in an abitrage formation that way you account for all possible outcomes. The problem with using real or large data you might be moving in cycles if you are not awere they may look different but in reality they are the same. What iam trying to say using data as a sample of simulation you may end up getting not all possible outcomes of which is a walk forward method .
Amazing book. 🎉
is there a code?
Hi! Thanks for the great video- wondering is there a link to the Presentation for the ML approach?
Try to understand Prado's concepts, your videos help a lot, thanks. Where can I get the source code and data to play myself?
Your course and its possible influence are impressive! I would be delighted to work with you to spread the word about it. Let's talk about how I can effectively assist in getting the word out. I'm interested in hearing your opinions! Warm regards.
Fake
Whats the difference between using the spread a - b or the ratio a/b when looking for stationarity and cointegration?
A-B = used to calculate the volatility spread. A/B = used to calculate the price spread.
Too much mouse-clicking noise...
what is the point of making a state space with a big dimension ?
amazing video
Hoodsohne and tomes need to get better speekers
explication is too vague
Your mic is so bad
акцент выдал с первой секунды(
The tick imbalance bars concept is still unclear. The book seems to jump right into the algorithm rather than explaining the concept behind it. What sources do you recommend referring to aside from this video and your book?
The book is the only place, I can made a video on it if you'd like.
@@AlgebraicContinuation That would be amazing!
Hello, I hope this message finds you well. I would greatly appreciate a few minutes of your time for a constructive conversation regarding your Udemy course. If possible, would you be available for a quick call? Thank you in advance for considering my request.
This video is missing several key aspects of explaining TBM and its practical considerations.
please next time only put up a video with 10,000 trades mininum and 20+ assets
Thanks for the clear and easy to understand lecture. ❤❤
Random comment, I hear your South African accent lol. Much love from SA!
So, is this approach correct? First, we train the primary model using historical data. Then, we train a secondary model to identify false positives. For instance, if the primary model predicts a positive outcome, we rely on the secondary model to assess whether to trust that prediction or not. We don't apply this process to negatives since it doesn't impact our pnl. However, considering market stationarity and other factors, I'm unsure how to effectively evaluate these models. Traditional train-test methods are flawed due to the non-stationary nature of the market, and it seems we lack reliable tools to prevent overfitting.
Quality stuff, thank you
could you give an idea about the architecture used for DQN?
Somebody's been quite busy in last few hrs :D I am not complaining, good to see quality content after a big break :) Excited for more.
You know, it's a shame that clickbait financial videos have millions of views, when videos like this are where the real money is
These eastern Europeans barely can string an Articulate sentence
Understand the rapid development timeline of large language models and its implication for open-source initiatives. 0:25 Adopt low-rank adaptation (Lora) and quantization techniques to make the training of large language models more accessible. 5:59 Explore FinGPT as a potential framework for generating financial large language models, despite its current limitations. 10:00 Recognize the significance of data quality over data scale in fine-tuning large language models. 13:14 Consider creating open, goal-specific, fine-tuned financial large language models to advance the field beyond the capabilities of closed models like Bloomberg GPT. 18:18 Publish and share data sets and trained model weights to democratize the development of financial large language models. 21:32 Challenge proprietary control over large language models and contribute to the open-source development to ensure equitable access and innovation. 21:53
they just wanted to get published in NeuralIPS conf. and get some media attention. FinGPT is a crap. I looked at the code. I can develop that in a week. But what is the point of developing such a useless open source which solves nothing. Bloomberg GPT is also crap if any one evaluated it in detail.
Have examples of a good project?
Your take on BB terminal?
Problem. Can it replicate radial asymmetry and association strength being an increasing function of volatility and financial distress
You have mentioned machine learning many times in the video. Isnt Pairs trading based on Statistics (White Box Models) not on Machine Learning (Black Box Models)?
Pairs trading is a spectrum with many approaches to solving the problem of trading a mean reverting spread. Machine learning is largely used to construct these spreads, or find components to do so.
If the beta is calculated by the MLL then why overfit the ou model with another calculate😂
Truly enjoy watching your research and analysis, keep it going .
I think the project has advanced in the last couple of months.