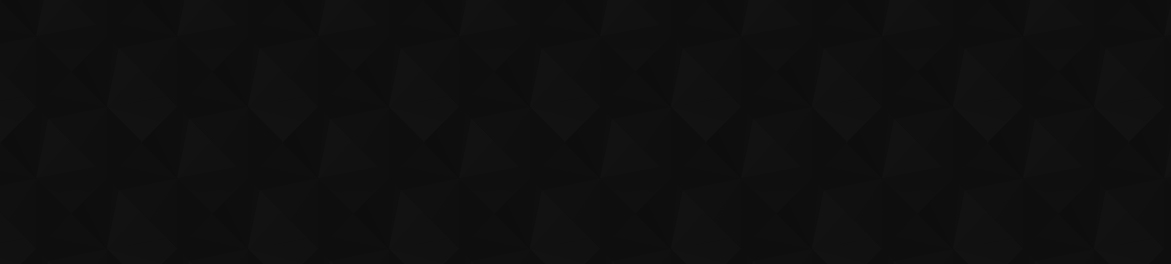
- 94
- 31 995
Serious Solvers
South Africa
Приєднався 6 бер 2024
Making advanced math and engineering easy to understand for everyone-learn, explore, and master complex concepts!
Expectation of Discrete Random Variables
In this video, we look into the concept of expectation (or expected value) for discrete random variables. From its definition as a weighted average to its significance in probability and real-world applications, we break it down step-by-step with intuitive explanations and examples.
🔍 What You'll Learn:
What is the expectation of a discrete random variable?
Why it’s a “weighted average” and what it represents.
A practical, conceptual derivation of the expectation for the binomial and Poisson random variables.
Whether you're a student, engineer, or just curious about probability theory, this video will help you understand the expectation of random variables in a way that makes sense!
00:00 - Introduction
00:54 - Conceptual overview
06:56 - Expectation of binomial random variable
15:49 - Expectation of Poisson random variable
🔍 What You'll Learn:
What is the expectation of a discrete random variable?
Why it’s a “weighted average” and what it represents.
A practical, conceptual derivation of the expectation for the binomial and Poisson random variables.
Whether you're a student, engineer, or just curious about probability theory, this video will help you understand the expectation of random variables in a way that makes sense!
00:00 - Introduction
00:54 - Conceptual overview
06:56 - Expectation of binomial random variable
15:49 - Expectation of Poisson random variable
Переглядів: 47
Відео
What Exactly is the Poisson Distribution? Where Does it Come From?
Переглядів 26014 днів тому
In this video, we look at the Poisson distribution, a powerful tool for modeling rare events and understanding randomness. Starting from its roots in the binomial distribution, we unravel its meaning, assumptions, and real-world significance. Through real-world examples-like safeguarding satellites from meteoroid strikes-we demonstrate how it predicts probabilities in high-stakes scenarios. Whe...
How Binomial Random Variables Simplify Real World Problems
Переглядів 56Місяць тому
Binomial random variables are everywhere - from predicting quiz test results to modeling business outcomes. In this video, we’ll explore how this powerful concept helps us understand and solve real-world problems in simple, relatable ways. Whether you're tackling probabilities in school, diving into data analysis, or just curious about the math behind everyday events, this video breaks it all d...
Permutations vs Combinations EXPLAINED Simply
Переглядів 117Місяць тому
Confused about permutations and combinations? Wondering when order matters and when it doesn’t? This video makes it crystal clear with simple explanations! Learn how to differentiate these concepts and apply them to solve problems. In this video, you'll discover: ✅ What permutations and combinations are. ✅ The key difference: when does order matter?
What Are Random Variables? Simplified Explanation
Переглядів 1,3 тис.Місяць тому
🎲 What Are Random Variables? Discover the Math Behind Uncertainty! 🎲 Ever wondered how mathematicians and scientists make sense of uncertainty? Whether it's predicting weather patterns, analyzing stock trends, or rolling dice in a game, random variables are the secret ingredient that connects random events to real-world numbers. In this video, we'll break down the basics of random variables. ✅ ...
Artificial Neural Networks Made Simple: Learn & Create One in Excel (No Coding!)
Переглядів 7 тис.Місяць тому
Curious about Artificial Neural Networks (ANNs) but intimidated by coding? In this video, we break down the basics of ANNs step-by-step using a simple example built in Excel-no programming required! Watch as we demystify how neural networks learn, adjust weights, minimize errors, and recognize patterns in data. Key highlights include: ✅ How neurons, layers, and weights work together ✅ Visualizi...
Principal Component Analysis Made Simple: Why PCA is a Game-Changer in Data Analysis
Переглядів 9312 місяці тому
Discover how Principal Component Analysis (PCA) simplifies complex datasets to uncover key insights! Using examples like weather data and health metrics, we break down concepts like variance, covariance, and eigenvectors to show how PCA identifies patterns and reduces dimensionality. Watch as we explain each step-from building a covariance matrix to finding directions of maximum variance-all wi...
Overview of Inner Product Spaces, Orthogonality, Gram Schmidt Method, and Hilbert Spaces
Переглядів 2222 місяці тому
In this video, we explore some of the foundational concepts in linear algebra that play crucial roles in quantum mechanics and advanced mathematics: inner product spaces, orthogonality, the Gram-Schmidt process, and Hilbert spaces. We start by explaining the concept of inner products-how they help define angles, lengths, and the idea of orthogonality. We’ll see how the inner product provides us...
Matrices in Quantum Mechanics - Hermitian, Unitary, Eigenstates
Переглядів 2,2 тис.2 місяці тому
We look at how linear algebra is applied to some foundational ideas in quantum mechanics, such as Hermitian and unitary matrices. Starting with the double-slit experiment, we discuss why particles, like electrons, exhibit wave-like behavior and how this ties into the concepts of quantum states and superposition. We'll break down key ideas like the quantum state vector, eigenstates, and why Herm...
Matrix Powers Explained: Unlocking the Secrets of Diagonalization and Steady States
Переглядів 1,2 тис.2 місяці тому
In this video, we explore matrix diagonalization and why it simplifies raising matrices to higher powers. Using an example of population movement, we uncover how repeated multiplication reveals steady states-long-term patterns in dynamic systems. Watch as we break down diagonalization step by step, making complex calculations simple and practical. 00:00 - Introduction - what is diagonalization,...
Easy trick to remember 9x table
Переглядів 502 місяці тому
The tens digit goes up: 0, 1, 2, 3, ..., 8. The ones digit goes down: 9, 8, 7, 6, ..., 1.
What Exactly are Eigenvalues and Eigenvectors? What is the point of them? Why do we learn about it?
Переглядів 9 тис.2 місяці тому
Many videos and textbooks just tell you how you how to determine eigenvalues and eigenvectors. However, there is often very little contextualization. What are they? What is the point of doing it? What is the value and purpose of determining them? This video answers all these questions. 00:00 - What are eigenvalues and eigenvectors? What is the point of doing it? 06:50 - Significance of eigenvec...
Laplace Transforms - A Full Course in One Video
Переглядів 2913 місяці тому
This video gives in a single video, a complete course in Laplace Transforms that would be useful to students and professionals looking to apply Laplace Transforms in various fields such as engineering, physics, and chemistry. 00:00:00 - Introduction - why study Laplace Transforms 00:01:33 - Definition of Laplace Transforms 00:02:54 - Laplace Transform of a constant 00:05:28 - Laplace Transform ...
Convolution (Laplace Transform) - What does Convolution Even Mean?? SIMPLEST EXPLANATION
Переглядів 3753 місяці тому
Many videos and textbooks just write the mathematical definition of convolution and then apply Laplace Transforms to it. However, there is often very little contextualization. What is convolution? How is it done? What is the point of doing it? Why apply the Laplace Transform to it? This video answers all these questions using a simple analogy of water from a tap filling up a container. This com...
6. How to Find the Inverse of a Matrix in Four Easy Steps
Переглядів 753 місяці тому
6. How to Find the Inverse of a Matrix in Four Easy Steps
4. A Useful Property of Determinants that can Greatly Simplify Calculations
Переглядів 543 місяці тому
4. A Useful Property of Determinants that can Greatly Simplify Calculations
3. Why Systems of Equations Need to be Consistent.. What does Consistency Even Mean?
Переглядів 633 місяці тому
3. Why Systems of Equations Need to be Consistent.. What does Consistency Even Mean?
2. How to Evaluate Third Order Determinants
Переглядів 963 місяці тому
2. How to Evaluate Third Order Determinants
The Determinant Demystified: The Easiest Explanation of What They Actually Are
Переглядів 3123 місяці тому
The Determinant Demystified: The Easiest Explanation of What They Actually Are
Complex Analysis - A Full Course in One Video.. Brush up on CA in one afternoon
Переглядів 3144 місяці тому
Complex Analysis - A Full Course in One Video.. Brush up on CA in one afternoon
3. Integral Functions: Error Function and Gaussian Distribution
Переглядів 4344 місяці тому
3. Integral Functions: Error Function and Gaussian Distribution
2. Integral Functions - The Beta Function
Переглядів 814 місяці тому
2. Integral Functions - The Beta Function
1. Integral Functions: The Gamma Function
Переглядів 2324 місяці тому
1. Integral Functions: The Gamma Function
9. What Exactly is the Dirac Delta Function? What is the Laplace Transform of the DDF?
Переглядів 764 місяці тому
9. What Exactly is the Dirac Delta Function? What is the Laplace Transform of the DDF?
8. Laplace Transform of Periodic Functions
Переглядів 574 місяці тому
8. Laplace Transform of Periodic Functions
7. What Exactly is the Heaviside Unit Step Function? What is the POINT of it? SIMPLEST EXPLANATION
Переглядів 424 місяці тому
7. What Exactly is the Heaviside Unit Step Function? What is the POINT of it? SIMPLEST EXPLANATION
6. Solving Differential Equations with Laplace Transforms
Переглядів 904 місяці тому
6. Solving Differential Equations with Laplace Transforms
5. Inverse Laplace Transforms with Partial Fractions
Переглядів 664 місяці тому
5. Inverse Laplace Transforms with Partial Fractions
Awesome pls keep us updated 🎉
Nicely done..
Excellent video!
I haven't seen this explained in this way before and just like your video on EigenVectors and EigenValues, my penny has dropped and this all makes sense now. Thank you once again!
I’m glad you’re making this video 🎉 I hope that you can make a video in matrix factorization please 😊
Thank you this has helped tp crystallise a vague understanding of "a vector that doesn't change under a linear transformation".
Thank you so much 😊
man, thank you so much for your explanation. you have gained a new sub
The best explanation of deep neural networks ever
Great content my fellow Mathematician ❤❤ I have subscribed
Thanks a lot
Keep going pro we want full play list for statistics 🎉 we enjoying by this your explanation is amazing
I asked chat gpt to recommend me a video and it recommended yours
Damn thanks man
this is the best explanation of ANN, i hope you create a playlist of everything about ml
Thank you! Yes, I will definitely look at doing that
Very good explanation. Thank you for sharing the Excel file
great video brother
Glad you liked it :)
Great explanation!
For those That prefer a mechanical analog you can look at harmonics of a guitar string and such. The video I present is another mechanical method of quantizing a system. It is one of two methods where structures can actually be produced. ua-cam.com/video/wrBsqiE0vG4/v-deo.htmlsi=waT8lY2iX-wJdjO3 Area under a curve is often equivalent to energy. Buckling of an otherwise flat field shows a very rapid growth of this area. If my model applies, it may show how the universe’s energy naturally developed from the inherent behavior of fields. Under the right conditions, the quantization of a field is easily produced. The ground state energy is induced via Euler’s contain column analysis. Containing the column must come in to play before over buckling, or the effect will not work. The sheet of elastic material “system” response in a quantized manor when force is applied in the perpendicular direction. Bonding at the points of highest probabilities and maximum duration( ie peeks and troughs) of the fields “sheet” produced a stable structure when the undulations are bonded to a flat sheet that is placed above and below the core material.
@ 15:25 You don't have a different wave function at each position. There is only one wave function. But at different positions the wave function has different (complex) values! These values are related to the probability of finding the particle in that position.
100%. My apologies. I think I misspoke there. A single continuous wavefunction across the whole space takes on probability amplitudes psi1, psi2 etc for each position
@@SeriousSolvers psi1 = psi(x1), etc.
@@SeriousSolvers can you do a noncommutative Basil J. Hiley vid? thanks
Helpful,please do something on Hilbert spaces
Great video! Nice overview of QM
Glad you enjoyed it
ua-cam.com/video/gJDgISHWfCg/v-deo.html
nice video i just don't understand something, in PCA the eignvector of matrix variance covariance why does it give us the maximum variance of the data spread ?
So basically the covariance matrix is set up in a way that tells us the spread of data in all possible directions. The eigenvectors of the covariance matrix correspond to the principal components. These are the directions or axes where the data is spread out the most, or has the most variability. Now, in my video I explained that if a matrix transforms a vector, and we get a resulting vector is in the same direction as the original vector, then it is an eigenvector. The same holds true of the covariance matrix. Here, the eigenvectors represent directions where the variance is stable, unchanging, and not fluctuating with direction. That means that the variance is being maximized in that particular direction. We can think of a simple scatter on the x-y plane. If most of the points lie on a diagonal line, then the eigenvector will be in the direction of that diagonal because that is where the data has the maximum stable spread. The scaling factor is the eigenvalue. The largest eigenvalue will give us the maximum variance along that eigenvector.
@@SeriousSolvers thank you so much i really appreciate it.
Nicely done
Thanks!
Great explanation!
Thanks for this wonderful content , can you make a video for why determinant is the surface !
Can we say " in a nutshell" the eigen vector and eigen value is the same intuition of a vector and it's unit vector ?, my second question, why to pick Lambda = 3 not 4 ? How do we know what to pick ?
@@Hassan-t4s you are right that it looks like eigenvectors have something in common with unit vectors. If we have a vector in a certain direction, it's unit vector is in the same direction as well but has a magnitude of 1. However, an eigenvector has more subtleties, and a bit more utility as well. Eigenvectors are vectors that experience no change in direction if a transformation is applied to it. It tells us specifically about something that isn't changing in a system, like the centre of a spinning wheel. With regards to lambda equal to 4, it's not the case that you choose a particular value. The answer to the question is that both 3 and 4 are eigenvalues. However, if you substitute 4 into the main equation to get the eigenvector, you'll get a trivial solution, i.e. both x1 and x2 are equal to zero. In general, you are supposed to substitute both to find the eigenvectors. Perhaps I should have mentioned this in the video for the sake of completeness. My apologies
@@SeriousSolvers in others words, as scheme programmer, it's all about a function taking a vector, apply * of a matrix and give back another vector; if the scale factor, the eigenvalue is 1, the eigenvector can be seen as the fixed point of that function related to that specific matrix. i guess, it's a property of each matrix to have or not some eigenvectors ...
@@marc-andrebrun8942 You're absolutely right-eigenvectors and eigenvalues are tied to how a matrix transforms vectors. It's interesting to see these concepts connect across different fields, like programming in scheme
@@SeriousSolvers programming in scheme is mind blowing because is much more than any other CS languages; mainly because from the beginning, LISP stick closely to the maths, lambda calculus; the LISP's tower number is pretty smart! C, C++, Python, Java, Pascal, to talk about some most popular, are like a house or a flat that you can rent it for a while, but you take it as it is, if it fits yours needs. Scheme is more than that : it gives you acces to an ideal store of stuffs and concepts such that you can build your own flat or house. among "crazy thing" of symbolic programming, is that the symbols can represent "data" or "code", so that, data & code can become the same thing ... en.wikipedia.org/wiki/Homoiconicity
Great!
125th view It will have millions of views one day
Thank you!
thank you
You're welcome!
Thank you bro! Great video to remember complex analysis. My professor at the university had a bad level to teach this....
on some of bounded conditions, by mistake U forgot signed. For ex., hwf can it be: infinity<y<0?
please do a laplace of three multiple funtions
Hi, great video this is very similar to my Further Engineering Maths book I wonder if this is a standard way of teaching the subject? though more seriously how do you deal with the infinite cases of these polygons, I have been shown the "triangle" with a single vertex being taken to infinity however I don't see how that limiting value for the term in the product will go to 1 i.e. (w-u)^{\frac{α}{\pi}-1} I would assume as you take the vertex to infinity the α would go to 0 thus the exponent would go to -1 thus you would get (w-u)^-1 which isn't the expected behaviour of at the limit the value of this term in the product going to 1. Is there a better way of explaining how this limiting process works?
also fyi I'm annoyed that I didn't find this channel earlier this is nearly exactly what I have been looking for on yt thx keep up the good work
Hi there. Appreciate the engagement. Part of my approach is inspired by the book Advanced Engineering Mathematics by Stroud. It's quite intuitive, and I haven't seen it in many other books. I hope I've understood your query correctly. You are right that the exponent goes to -1. This means that u goes to infinity, and the term behaves like 1/u. Taking the limit suggests that the term approaches 0 rather than 1. However, the overall integral doesn't go to 0. This terms "smallness" is compensated by the rest of the integrand and the overall scaling factor "A".
Thank you! Glad it's helpful
@@SeriousSolvers yh that makes sense my book is further engineering maths by KA Stroud so that probably the similarity (Same Author). thanks and for the reply on my other question, I take it that as u goes to infinity the w in the denominator becomes negligable fast enough that as you take the limit the term becomes like a constant "sooner" that it becomes like zero
Excellent!
Thank you, glad you liked it!
Nice!
Thank you sir
Make sure to like and subscribe