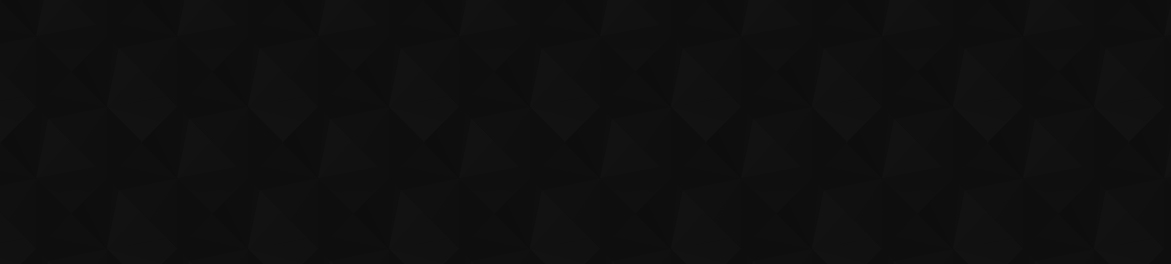
- 6
- 27 727
Stanford Contrastive & SS Learning Group
United States
Приєднався 1 лют 2021
Discussion group on contrastive and self-supervised learning, 2021.
Livestreamed discussion groups will take place every other Friday (dates below) from 2pm-2:30pm:
Feb 26, 2021 02:00 PM. Speaker: Dan Fu, Topic: SimCLR
Mar 12, 2021 02:00 PM
Mar 26, 2021 02:00 PM
Apr 9, 2021 02:00 PM
Apr 23, 2021 02:00 PM
Livestreamed discussion groups will take place every other Friday (dates below) from 2pm-2:30pm:
Feb 26, 2021 02:00 PM. Speaker: Dan Fu, Topic: SimCLR
Mar 12, 2021 02:00 PM
Mar 26, 2021 02:00 PM
Apr 9, 2021 02:00 PM
Apr 23, 2021 02:00 PM
Representation Learning for Sequence Data with Deep Autoencoding Predictive Components
Presenter: Siyi Tang
Affiliation: Stanford University
Article's title: Representation Learning for Sequence Data with Deep Autoencoding Predictive Components
Authors: Bai, Junwen, Weiran Wang, Yingbo Zhou, and Caiming Xiong
Institutions: Cornell University, Google, Salesforce Research
Paper: arxiv.org/abs/2010.03135
Abstract:
"We propose Deep Autoencoding Predictive Components (DAPC) -- a self-supervised representation learning method for sequence data, based on the intuition that useful representations of sequence data should exhibit a simple structure in the latent space. We encourage this latent structure by maximizing an estimate of predictive information of latent feature sequences, which is the mutual information between past and future windows at each time step. In contrast to the mutual information lower bound commonly used by contrastive learning, the estimate of predictive information we adopt is exact under a Gaussian assumption. Additionally, it can be computed without negative sampling. To reduce the degeneracy of the latent space extracted by powerful encoders and keep useful information from the inputs, we regularize predictive information learning with a challenging masked reconstruction loss. We demonstrate that our method recovers the latent space of noisy dynamical systems, extracts predictive features for forecasting tasks, and improves automatic speech recognition when used to pretrain the encoder on large amounts of unlabeled data."
Affiliation: Stanford University
Article's title: Representation Learning for Sequence Data with Deep Autoencoding Predictive Components
Authors: Bai, Junwen, Weiran Wang, Yingbo Zhou, and Caiming Xiong
Institutions: Cornell University, Google, Salesforce Research
Paper: arxiv.org/abs/2010.03135
Abstract:
"We propose Deep Autoencoding Predictive Components (DAPC) -- a self-supervised representation learning method for sequence data, based on the intuition that useful representations of sequence data should exhibit a simple structure in the latent space. We encourage this latent structure by maximizing an estimate of predictive information of latent feature sequences, which is the mutual information between past and future windows at each time step. In contrast to the mutual information lower bound commonly used by contrastive learning, the estimate of predictive information we adopt is exact under a Gaussian assumption. Additionally, it can be computed without negative sampling. To reduce the degeneracy of the latent space extracted by powerful encoders and keep useful information from the inputs, we regularize predictive information learning with a challenging masked reconstruction loss. We demonstrate that our method recovers the latent space of noisy dynamical systems, extracts predictive features for forecasting tasks, and improves automatic speech recognition when used to pretrain the encoder on large amounts of unlabeled data."
Переглядів: 820
Відео
DINO: Emerging Properties in Self-Supervised Vision Transformers
Переглядів 5 тис.3 роки тому
Presenter: Michael Zhang Affiliation: Stanford University Article's title: DINO: Emerging Properties in Self-Supervised Vision Transformers Authors: Mathilde Caron, Hugo Touvron, Ishan Misra, Hervé Jégou, Julien Mairal, Piotr Bojanowski, Armand Joulin Institutions: Facebook AI Research, Inria, Sorbonne University Paper: arxiv.org/abs/2104.14294 Article's abstract: "In this paper, we question if...
ALIGN: Scaling Up Visual and Vision-Language Representation LearningWith Noisy Text Supervision
Переглядів 9693 роки тому
Full paper: arxiv.org/pdf/2102.05918.pdf Presenter: Nandita Bhaskar Stanford University, USA Abstract: Pre-trained representations are becoming crucial for many NLP and perception tasks. While representation learning in NLP has transitioned to training on raw text without human annotations, visual and vision-language representations still rely heavily on curated training datasets that are expen...
thanks for sharing guys
In 24:05, I think the green bar is only present for the 2048 dimensional size (in the y-axis) and not for the other dimensionalities because the representation 'h' is fixed (2048) according to the caption in the figure 8 of the paper. In the presence of a linear or non-linear layer, the output dimension may be altered (32, 64, 128 etc), but for no-projection, the 'h' is directly being used in the loss function. Since, h is fixed for 2048, it is not being compared for other dimensional sizes.
Really good explanation! You eased my burden! Can you share paper presentation slides Dan Fu?
Khub valo laglo.
It was a good summary indeed...seeing this after going through the paper actually makes more sense!
The group is awesome.. they are asking most question that the audience might ask!
This is, like, impossible to, like, listen to, right?
Good work
What is the intuition behind using pooling rather than strides to downsize the features for U-Net MSS? Based on the explanation provided by florian for multi scale, why not use pyramid architecture models like pspnet?
why is that 2 FC Layers layers become non-linear at 25:00?
I think for the 1 FC case, it's just the linear layer without activation, and in the 2 FC case, there is a relu activation between them that makes it nonlinear (btw "relu" in that slide is written in a small font so it's hard to see)
👌 Great work mate. You should check promosm!! ! It’s a great way to quickly grow your channel.
Thank you, this was really helpful to me to understand the concepts of this paper. I want to share this presentation to the South Korean researchers. Would you mind if I write a blog post about this presentation in Korean and introduce this video(by attaching this video link)?
Thank you, glad it helps! We would be more than happy that you write a blog post in Korean and link the video. Very nice initiative and good luck with the writing!
How can i Participate
Thank you, this was a really good summary.
Glad it was helpful!
Hi- For visualisation of masks, it is mentioned in the paper that the mask is obtained by thresholding the self attention maps to keep 60% of the mass. What does the mass represent here? Can you please explain this thresholding technique a bit. Thank you
Is this group open to scientific comments or not?!!!! I put a critic for the ViT method and it has been deleted, really weird behaviour!
Hi Sherif, yes this channel is very open to scientific comments and feedback from the community, thank you very much for participating. I am not sure what happened with your comment. I am only able to see the beginning of your comment in the channel notifications. Do you mind trying to post it again? I suspect it may have been automatically deleted for some reason. I see that we got the notification about your comment twice, so my best guess right now would be that you submitted it twice by accident and that it was detected as spam. But that's just a wild guess. If you are still having issues, just send your comment to stanfordcontrastivelearning [at] gmail.com and we will repost it with quotation marks. We do not want to censor anybody!
The attention maps visualization is from the output CLS token
Nice video! Minor remark on the last question: we do show comparison with other self-supervised losses for Jaccard distance with deit-S 16x16 in Appendix :). Our conclusion is that the segmented heat maps appear for all the SSL works we experimented with!
Thank you for the comment, that clarifies it! And keep up the good work. Reviewing the paper was very nice!
Great explanation guys!! Is there a slack or discord channel where I could connect with you and contribute in the future?
Hi Kartik, thank you! Very nice to hear that you want to contribute too! Would you like to only participate in the discussion or also present a paper yourself?
@@stanfordcontrastivesslearn3141 thank you for the reply! I would like to present a paper. If that's possible?
@@kartiksachdev8807 Do you already know what paper you would like to present?
@@stanfordcontrastivesslearn3141 yes, I have one paper in mind.
@@kartiksachdev8807 Ok nice! You can write us at stanfordcontrastivelearning [at] gmail.com, send us a bio, let us know what article you would like to present, and we will give you the instructions.
It's good to see that you guys are explaining latest SOTA techniques. Keep up the good work guys!
Thanks. Happy to hear that it also helps you guys online!
@@stanfordcontrastivesslearn3141 My pleasure!
Start at 1:25
Start at 4:40
starts at 6:30 contrastive loss 9:20 self supervised contrastive loss 15:30 key findings 20:07
thanks