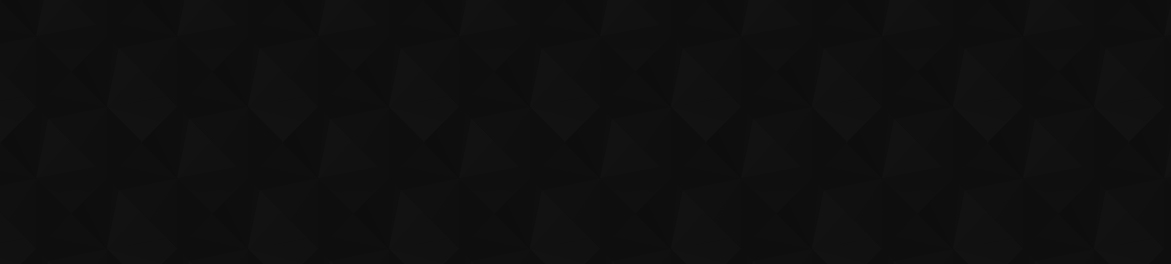
- 48
- 370 525
Netflix Data
Приєднався 18 лип 2016
Unbundling the Data Warehouse: The Case for Independent Storage
Speaker: Jason Reid (Co-founder & Head of Product at Tabular)
This tech talk is a part of the Data Engineering Open Forum at Netflix 2024. Unbundling a data warehouse means splitting it into constituent and modular components that interact via open standard interfaces. In this talk, Jason Reid discusses the pros and cons of both data warehouse bundling and unbundling in terms of performance, governance, and flexibility, and he examines how the trend of data warehouse unbundling will impact the data engineering landscape in the next 5 years.
If you are interested in attending a future Data Engineering Open Forum, we highly recommend you join our Google Group (groups.google.com/g/data-engineering-open-forum) to stay tuned to event announcements.
This tech talk is a part of the Data Engineering Open Forum at Netflix 2024. Unbundling a data warehouse means splitting it into constituent and modular components that interact via open standard interfaces. In this talk, Jason Reid discusses the pros and cons of both data warehouse bundling and unbundling in terms of performance, governance, and flexibility, and he examines how the trend of data warehouse unbundling will impact the data engineering landscape in the next 5 years.
If you are interested in attending a future Data Engineering Open Forum, we highly recommend you join our Google Group (groups.google.com/g/data-engineering-open-forum) to stay tuned to event announcements.
Переглядів: 4 271
Відео
Reflections on Building a Data Platform From the Ground Up in a Post-GDPR World.
Переглядів 1,4 тис.2 місяці тому
Speaker: Jessica Larson (Data Engineer & Author of “Snowflake Access Control”) This tech talk is a part of the Data Engineering Open Forum at Netflix 2024. The requirements for creating a new data warehouse in the post-GDPR world are significantly different from those of the pre-GDPR world, such as the need to prioritize sensitive data protection and regulatory compliance over performance and c...
Data Productivity at Scale
Переглядів 1,4 тис.2 місяці тому
Speaker: Iaroslav Zeigerman (Co-Founder and Chief Architect at Tobiko Data) This tech talk is a part of the Data Engineering Open Forum at Netflix 2024. The development and evolution of data pipelines are hindered by outdated tooling compared to software development. Creating new development environments is cumbersome: Populating them with data is compute-intensive, and the deployment process i...
Automating the Data Architect: Generative AI for Enterprise Data Modeling
Переглядів 7 тис.2 місяці тому
Speaker: Jide Ogunjobi (Founder & CTO at Context Data) This tech talk is a part of the Data Engineering Open Forum at Netflix 2024. As organizations accumulate ever-larger stores of data across disparate systems, efficiently querying and gaining insights from enterprise data remain ongoing challenges. To address this, we propose developing an intelligent agent that can automatically discover, m...
Real-Time Delivery of Impressions at Scale
Переглядів 2,1 тис.2 місяці тому
Speaker: Tulika Bhatt (Senior Data Engineer at Netflix) This tech talk is a part of the Data Engineering Open Forum at Netflix 2024. Netflix generates approximately 18 billion impressions daily. These impressions significantly influence a viewer’s browsing experience, as they are essential for powering video ranker algorithms and computing adaptive pages, With the evolution of user interfaces t...
Welcome Address for the Data Engineering Open Forum 2024
Переглядів 9402 місяці тому
Max Schmeiser (Vice President of Studio and Content Data Science & Engineering) extends a warm welcome to all attendees, marking the beginning of our inaugural Data Engineering Open Forum. If you are interested in attending a future Data Engineering Open Forum, we highly recommend you join our Google Group (groups.google.com/g/data-engineering-open-forum) to stay tuned to event announcements.
Machine Learning Powered Auto Remediation in Netflix Data Platform
Переглядів 1,7 тис.2 місяці тому
Speakers: Stephanie Vezich Tamayo (Senior Machine Learning Engineer at Netflix) Binbing Hou (Senior Software Engineer at Netflix) This tech talk is a part of the Data Engineering Open Forum at Netflix 2024. At Netflix, hundreds of thousands of workflows and millions of jobs are running every day on our big data platform, but diagnosing and remediating job failures can impose considerable operat...
Data Quality Score: How We Evolved the Data Quality Strategy at Airbnb
Переглядів 2,6 тис.2 місяці тому
Speaker: Clark Wright (Staff Analytics Engineer at Airbnb) This tech talk is a part of the Data Engineering Open Forum at Netflix 2024. Recently, Airbnb published a post to their Tech Blog called Data Quality Score: The next chapter of data quality at Airbnb. In this talk, Clark Wright shares the narrative of how data practitioners at Airbnb recognized the need for higher-quality data and then ...
Netflix Data Engineering Tech Talks - Media Data for ML Studio Creative Production
Переглядів 3,1 тис.8 місяців тому
In the last 2 decades, Netflix has revolutionized the way video content is consumed, however, there is significant work to be done in revolutionizing how movies and tv shows are made. In this video, Sr. Data Engineers Amanual Kahsay and Dao Mi showcase how data and insights are being utilized to accomplish such a vision. #netflix #datascience #dataengineering #etl #bigdata
Netflix Data Engineering Tech Talks - Start Stop Continue for optimizing complex ETL jobs
Переглядів 3,6 тис.8 місяців тому
Judit Lantos, Data Engineer, Member Experience Data Engineering, shares a case study to demonstrate an effective approach for optimizing complex ETL jobs. #netflix #datascience #dataengineering #etl #bigdata
Netflix Data Engineering Tech Talks - Psyberg, An Incremental ETL Framework Using Iceberg
Переглядів 5 тис.8 місяців тому
Abhinaya Shetty and Bharath Mummadisetty, Data Engineers from Netflix’s Membership Data Engineering team, introduce Psyberg, an incremental ETL framework. Learn about how Psyberg leverages Iceberg metadata to handle late-arriving data, and improves data pipelines while simplifying on-call life! #netflix #datascience #dataengineering #etl #bigdata
Netflix Data Engineering Tech Talks - Knowledge Management - Leveraging Institutional Data
Переглядів 3,5 тис.8 місяців тому
Tristan Reid, software engineer, shares experiences about the Knowledge Management project at Netflix, which seeks to leverage language modeling techniques and metadata from internal systems to improve the impact of the more than 100,000 memos that circulate within the company #netflix #datascience #dataengineering #etl #bigdata
Netflix Data Engineering Tech Talks - Building Reliable Data Pipelines
Переглядів 8 тис.8 місяців тому
Holden Karau, OSS Engineer, Data Platform Engineering, talks about the importance of reliable data pipelines and how to build them covering tools from testing to validation and auditing. The talk uses Apache Spark as an example, but the concepts generalize regardless of your specific tools. Some related projects include: github.com/holdenk/spark-testing-base github.com/unionai-oss/pandera githu...
Netflix Data Engineering Tech Talks - Streaming SQL on Data Mesh
Переглядів 6 тис.8 місяців тому
Mark Cho, Guil Pires and Sujay Jain, Engineers from Data Platform talk about how a managed Streaming SQL using Apache Flink can help unlock new Stream Processing use cases at Netflix. You can read more about Data Mesh, Netflix's next generation stream processing platform, here: netflixtechblog.com/data-mesh-a-data-movement-and-processing-platform-netflix-1288bcab2873 #netflix #datascience #data...
Netflix Data Engineering Tech Talks - Data Processing Patterns
Переглядів 12 тис.8 місяців тому
Lee Woodridge and Pallavi Phadnis, Data Engineers at Netflix, talk about how you can apply different processing strategies for your batch pipelines by implementing generic abstractions to help scale, be more efficient, handle late-arriving data, and be more fault tolerant. #netflix #datascience #dataengineering #etl #bigdata
Netflix Data Engineering Tech Talks - The Netflix Data Engineering Stack
Переглядів 33 тис.8 місяців тому
Netflix Data Engineering Tech Talks - The Netflix Data Engineering Stack
Welcome to the world of Data Engineers at Netflix
Переглядів 24 тис.3 роки тому
Welcome to the world of Data Engineers at Netflix
2021 Apache Flink Meetup - Hosted by Netflix
Переглядів 6 тис.3 роки тому
2021 Apache Flink Meetup - Hosted by Netflix
Flink Meetup at Netflix (Los Gatos) - January 28, 2020
Переглядів 1,1 тис.4 роки тому
Flink Meetup at Netflix (Los Gatos) - January 28, 2020
Apache Cassandra Meetup Hosted by Netflix
Переглядів 2,2 тис.4 роки тому
Apache Cassandra Meetup Hosted by Netflix
Netflix Meetup - #SheRules in Big Data
Переглядів 1,2 тис.4 роки тому
Netflix Meetup - #SheRules in Big Data
Women in Big Data Meetup - Hosted by Netflix (Chicago)
Переглядів 7405 років тому
Women in Big Data Meetup - Hosted by Netflix (Chicago)
Netflix hosts the Women in Big Data Organization in Los Gatos and Chicago
Переглядів 1,9 тис.5 років тому
Netflix hosts the Women in Big Data Organization in Los Gatos and Chicago
Druid Meetup hosted by Netflix (11/14/2018)
Переглядів 4,1 тис.5 років тому
Druid Meetup hosted by Netflix (11/14/2018)
Netflix Research: Machine Learning Platform
Переглядів 3,3 тис.6 років тому
Netflix Research: Machine Learning Platform
Netflix Research: Experimentation & Causal Inference
Переглядів 7 тис.6 років тому
Netflix Research: Experimentation & Causal Inference
Quite interesting that a survey was performed so that the points were grounded by data
How can we connect with Betty Li? Any LinkedIn please?
Can you enable captioning of the videos?
Whats the point of posting this without proper capture
This is really cool. Great high level summary of what there is to know about modelling and AI. Thanks Jide
Thanks for sharing the internals at such details level. QQ as per initial design it was mentioned that for quick response request goes to key value data store and then towards the end it was mentioned those requests are catered by Cassandra.Also nowhere in actual design the request is going to impression table as shown in initial design
Buffet of choices also confusing and fattening. There is beauty in a few lean options. Who likes a complex menu of this or that?
Too few details on how the model was actually trained. One of the slides says OpenAI -> Weaviate. How does it actually happen? Weaviate is just a database after all: how the queries towards it are built? A blogpost with details will be highly appreciated. The idea is great but some additional technical details have to be disclosed.
Exceedingly high level
I'd expect Netflix's channel to upload better resolution and size of the slides. :/
"my ducatti is current broken again" lollll
Can you share the paper/blog link please? thx.
Very detailed talk, extremely informative.. Great work Tulika!
amazing
awesome presentation and perfect timing for me. i have to give a presentation in a few days explaining all this new composability in data/database word and why developers should care about it.
Good job keep it up 👍
Veri nice 👍
Ahhh I wish we had the slides in this one 😢
The slides kick in around the 8th minute. So the viewers miss out on some memes, but the core parts of the talk are still there 😂
Very nice presentation. Keep it up.
Very informative, keep up 👍
Great
Great ! ❤
Great! Informative 👍👍
The Struggle of Enterprise Data Modeling: A Data Architect's Journey [03:27](ua-cam.com/video/DtzIIVJq8wA/v-deo.html) Transitioning from data architect to generative AI expert - Discussing journey as a data professional over 16 years, focusing on data modeling and architecture roles at various companies - Detailing challenges faced as a data architect in managing data schemas, infrastructure, and collaborating with developers on data placement [06:54](ua-cam.com/video/DtzIIVJq8wA/v-deo.html) Challenges with disparate data and maintaining consistency in large organizations. - Data was duplicated and scattered across different teams, leading to difficulties in answering questions. - Complex processes of pulling and joining data from disparate systems and writing code for data consistency and unification. [10:21](ua-cam.com/video/DtzIIVJq8wA/v-deo.html) Automating data discovery, mapping, and integrations for a unified and accessible data view. - The AI agent automates data mapping, integrations across multiple organizations, and discovers data and relationships. - It also interprets metadata, infers data types and constraints, builds an ontological model, and continuously updates the model. [13:48](ua-cam.com/video/DtzIIVJq8wA/v-deo.html) Automating data architecture through generative AI - Data modeling involves logical and physical perspectives including entity attributes, relationships, inventory, structure definition, and data population. - Data collection sources range from Postgres, S3, Data Lake, operational systems like Salesforce and Zendesk, involving querying, schema inference, and reverse engineering SQL code. [17:15](ua-cam.com/video/DtzIIVJq8wA/v-deo.html) Using generative AI to enhance data modeling and querying - The process involved building a data ontology and pushing it into a vector database, specifically Weavio, to enable querying and building multiple levels of relationships - The aim was to provide a user-friendly experience by enabling free text search without the need to build a separate model [20:42](ua-cam.com/video/DtzIIVJq8wA/v-deo.html) Generative AI interprets queries for quick data access - Generative AI interprets user queries accurately - Feedback loop ensures data accuracy and user satisfaction [24:09](ua-cam.com/video/DtzIIVJq8wA/v-deo.html) Automated model updates and data tracking for improved decision-making - Ensures agents can learn and adapt by monitoring ontology and updating models with new data sources. - Removes the need for explicit database specifications, enabling intuitive free text search for better decision-making. [27:35](ua-cam.com/video/DtzIIVJq8wA/v-deo.html) Automating Data Architect with Generative AI - Implemented Snowflake data warehouse for executives to improve data queries and comparisons - Considering enhancing system with knowledge graph and open AI integration for better results
Quite an elaborate insights into inpressions🎉
Informative, good job!
Great content, learnt a lot....I wanted to know [ any viewer can answer as well if they got the answer] , how they ensured that in their SQS has no duplicates ? also, if batches are 10 mins apart, can't we use HWM table in OLTP systems to ensure we get ACID complaint ???
Hello netflix, i would like to be hired by you guys. I am a slightly below average software engineer. Maybe you guys could let me sweep the floors or something? I can be a FAANG janitor! Would just ask for maybe like $11 an hour plus a salad from the cafeteria maybe. I look forward to hearing back from you guys.
Wtf is this guy wearing?
doesn't really matter tbh
Thanks for sharing this to public!
Gonna be in the team soon!!
Wow, Must see video for every reliable data engineer!
What is High play starts in the example for Context specific Audits @11:30
How I wish
Intro dope afff
how you avoiding too high tide of a changes? meaning - is any late data arriving triggers Psyberg? even just few thousand of rows? or you accumulating changes at some sort of gates/elevators and process when enough late data accumulated to justify downstream reprocessing?
Thanks for sharing. For the comparison between Extractor pattern and DRY principle, it stands but it's not exactly the same driver: DRY principle in programming is to avoid to replicate the same logic - as code - multiple times (to avoid repetitions and incoherences). And this logic can be applied multiple times during the run. Here, the goal is to avoid repetitions for the run itself.
Is there any way you can enable transcript on the youtube video
will they open source Maestro like Airbnb/Airflow??
very smart, using new Acronyms for old Audit tables.
Straight up talk 👏
Where can I download this slide?
Great job Pallavi and Lee! Just had a couple questions: 1. Iceberg and Spark: do you have any challenges running these? Spark Shell not working after adding support for Iceberg, dependency issues w/ AWS and Iceberg, etc.? 2. Why use SQS instead of Kafka? 3. How do you overcome interpreting sessions / sessionization in real time?
Great presentation! Thank you for sharing this! 4:56 - why use Iceberg instead of Delta Lake and Hudi? 8:26 - how do data engineers verify quality data? Isn't that the business office's or data science team's responsibility? 10:08 - DE isn't always told when data source context changes/is updated. 100% true. 12:33 - sometimes = always, in my experience. :) 13:42 - why use python? perhaps due to the schedules? wouldn't scala be faster? 17:55 - Janitor sounds like an incredibly helpful tool!
So insightful. Thanks!
4:15 whats go table standard s?
Very useful.
This is such an exciting talk. I faced this problem in my experience working as a DE. Identifying and reprocessing those late-arriving records is resource-intensive and time consuming for sure. Thanks to Iceberg for making it easy and possible to put together a solution with the help of metadata.
Very useful information, thanks for sharing, I am a data engineer and found this series very useful. When you are talking about processed partition , how is the partition selected, through a CTE or Subquery?
Someday.... I'll work for Netflix 😊